Learning Graph Representations Through Learning and Propagating Edge Features
IEEE TRANSACTIONS ON NEURAL NETWORKS AND LEARNING SYSTEMS(2023)
摘要
Graph convolutional networks have achieved considerable success in various graph domain tasks. Recently, numerous types of graph convolutional networks have been developed. A typical rule for learning a node's feature in these graph convolutional networks is to aggregate node features from the node's local neighborhood. However, in these models, the interrelation information between adjacent nodes is not well-considered. This information could be helpful to learn improved node embeddings. In this article, we present a graph representation learning framework that generates node embeddings through learning and propagating edge features. Instead of aggregating node features from a local neighborhood, we learn a feature for each edge and update a node's representation by aggregating local edge features. The edge feature is learned from the concatenation of the edge's starting node feature, the input edge feature, and the edge's end node feature. Unlike node feature propagation-based graph networks, our model propagates different features from a node to its neighbors. In addition, we learn an attention vector for each edge in aggregation, enabling the model to focus on important information in each feature dimension. By learning and aggregating edge features, the interrelation between a node and its neighboring nodes is integrated in the aggregated feature, which helps learn improved node embeddings in graph representation learning. Our model is evaluated on graph classification, node classification, graph regression, and multitask binary graph classification on eight popular datasets. The experimental results demonstrate that our model achieves improved performance compared with a wide variety of baseline models.
更多查看译文
关键词
Edge feature propagation,graph convolutional networks,graph representation learning
AI 理解论文
溯源树
样例
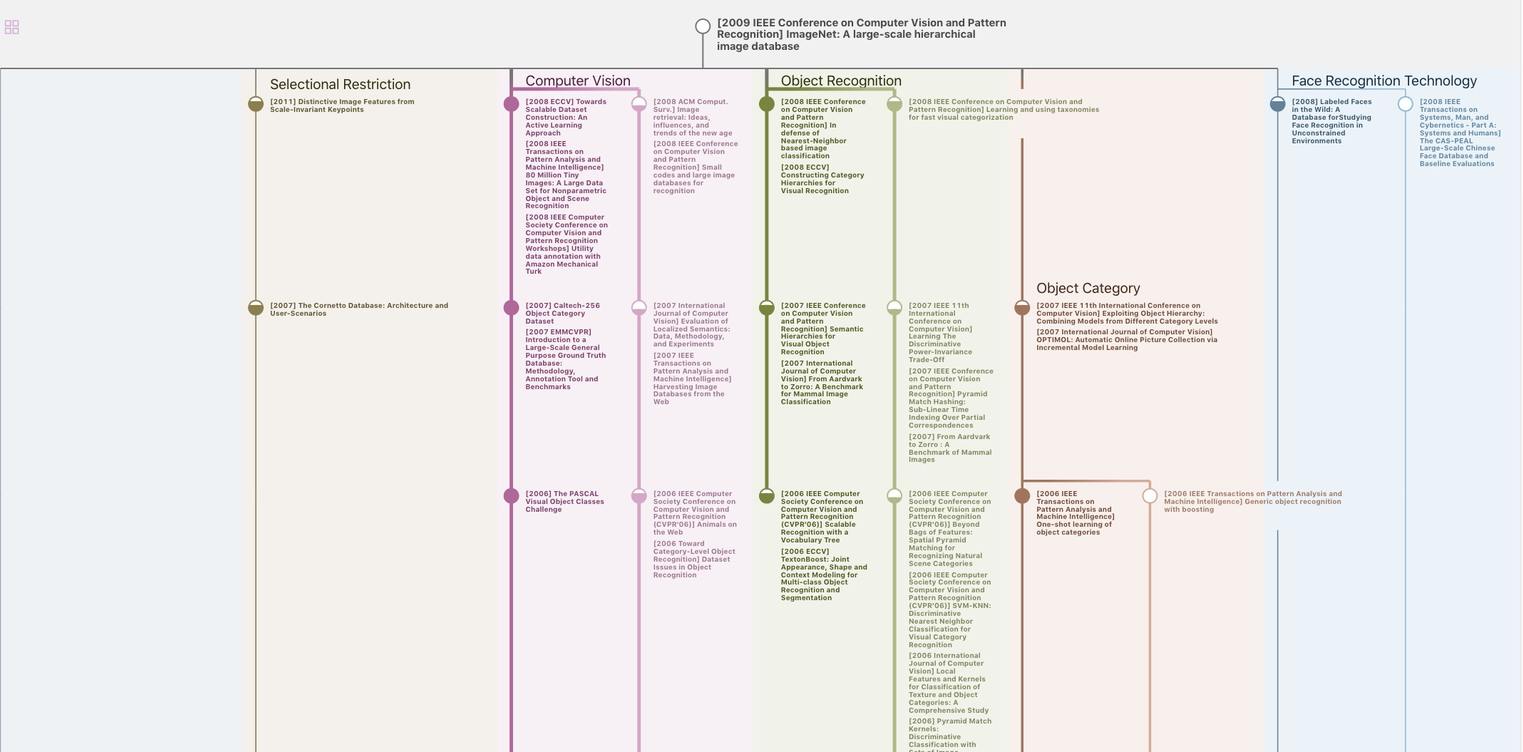
生成溯源树,研究论文发展脉络
Chat Paper
正在生成论文摘要