Estimation in discrete time coarsened multivariate longitudinal models.
Statistical methods in medical research(2023)
摘要
We consider the analysis of longitudinal data of multiple types of events where some of the events are observed on a coarser level (e.g. grouped) at some time points during the follow-up, for example, when certain events, such as disease progression, are only observable during parts of follow-up for some subjects, causing gaps in the data, or when the time of death is observed but the cause of death is unknown. In this case, there is missing data in key characteristics of the event history such as onset, time in state, and number of events. We derive the likelihood function, score and observed information under independent and non-informative coarsening, and conduct a simulation study where we compare bias, empirical standard errors, and confidence interval coverage of estimators based on direct maximum likelihood, Monte Carlo Expectation Maximisation, ignoring the coarsening thus acting as if no event occurred, and artificial right censoring at the first time of coarsening. Longitudinal data on drug prescriptions and survival in men receiving palliative treatment for prostate cancer is used to estimate the parameters of one of the data-generating models. We demonstrate that the performance depends on several factors, including sample size and type of coarsening.
更多查看译文
关键词
Coarsened data,Monte Carlo expectation maximization,longitudinal data,maximum likelihood,missing data,multi-state modeling
AI 理解论文
溯源树
样例
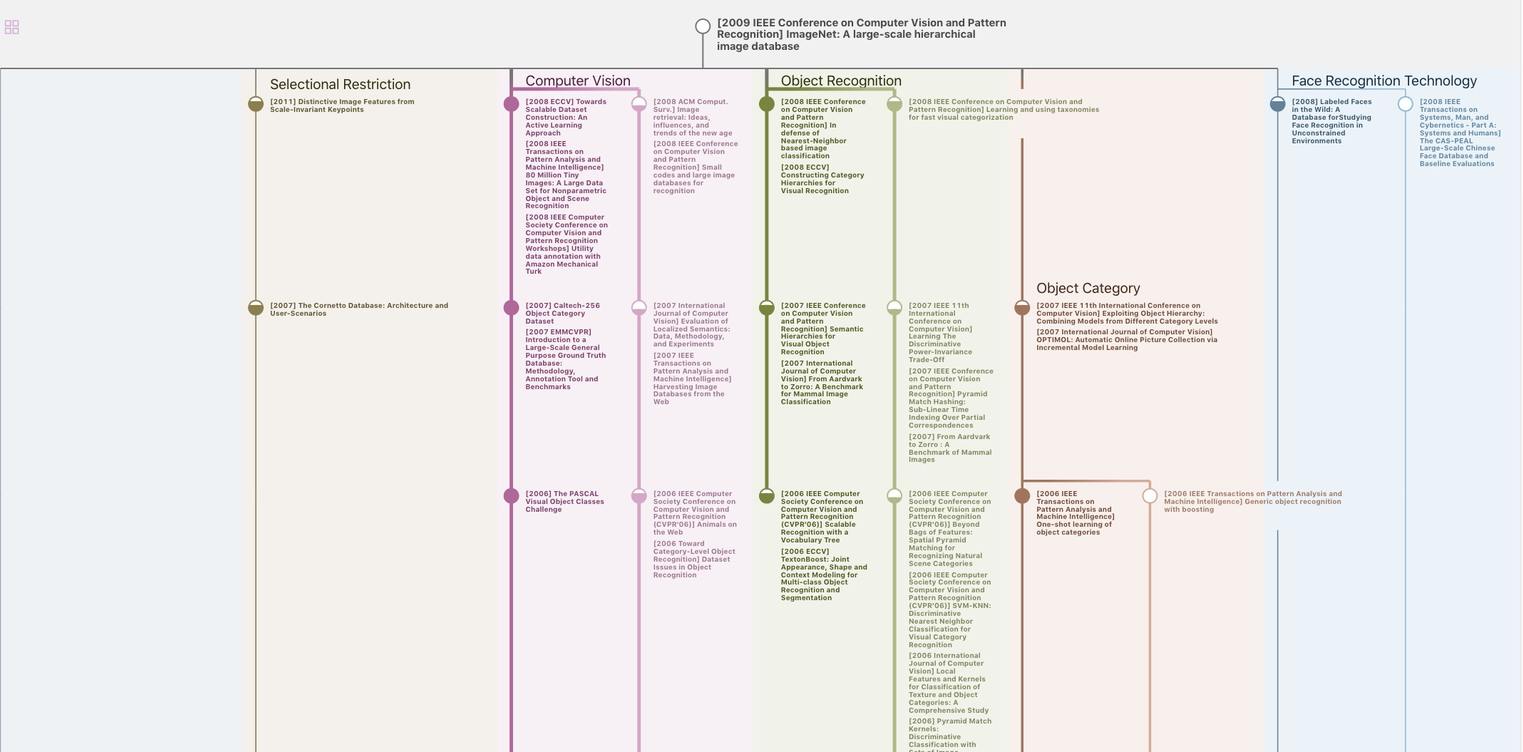
生成溯源树,研究论文发展脉络
Chat Paper
正在生成论文摘要