Evaluating Deep Learning Predictions for COVID-19 from X-ray Images Using Leave-One-out Predictive Densities
Neural computing & applications(2023)
摘要
Early detection of the COVID-19 virus is an important task for controlling the spread of the pandemic. Imaging techniques such as chest X-ray are relatively inexpensive and accessible, but its interpretation requires expert knowledge to evaluate the disease severity. Several approaches for automatic COVID-19 detection using deep learning techniques have been proposed. While most approaches show high accuracy on the COVID-19 detection task, there is not enough evidence on external evaluation for this technique. Furthermore, data scarcity and sampling biases make difficult to properly evaluate model predictions. In this paper, we propose stochastic gradient Langevin dynamics (SGLD) to take into account the model uncertainty. Four different deep learning architectures are trained using SGLD and compared to their baselines using stochastic gradient descent. The model uncertainties are also evaluated according to their convergence properties and the leave-one-out predictive densities. The proposed approach is able to reduce overconfidence of the baseline estimators while also retaining predictive accuracy for the best-performing cases.
更多查看译文
关键词
Bayesian learning,Markov chain Monte Carlo,COVID X-ray
AI 理解论文
溯源树
样例
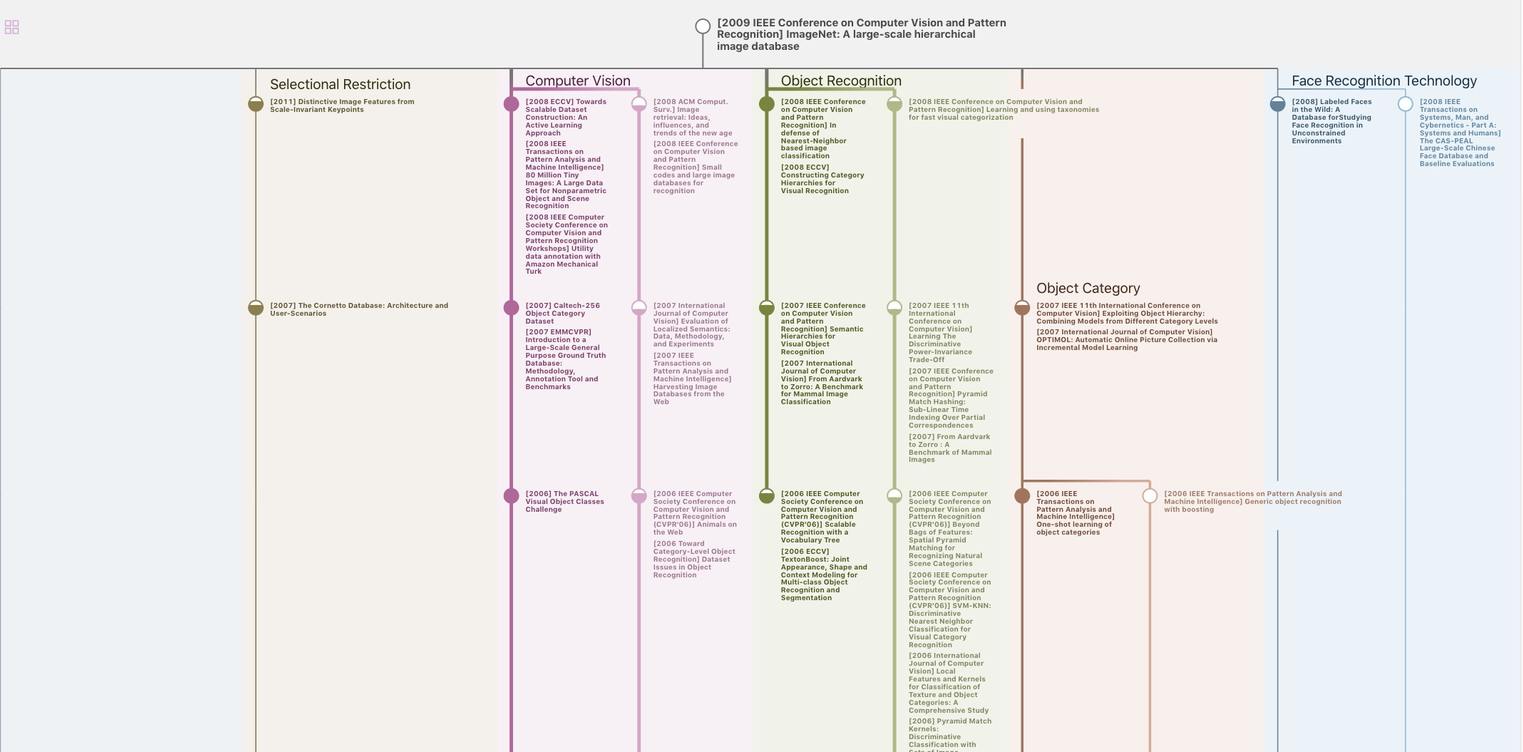
生成溯源树,研究论文发展脉络
Chat Paper
正在生成论文摘要