Fully automated analysis of NMR data with Bayesian nonparametric statistical learning
Biophysical Journal(2023)
摘要
The characterization of individual peaks in NMR spectra is an important but challenging step in the investigation of complex biomolecular systems. The major challenge arises due to peak identification which, conventionally, occurs prior to peak characterization. Unluckily, the identification of uncharacterized peaks is highly problematic because of noise and, most importantly, substantial overlap with nearby peaks. For this reason, often the analysis of NMR spectra proceeds by first relying on experts’ opinion to identify peaks and subsequent parameter fitting. Mathematically, peak identification is a problem of model selection and, within a nonparametric analysis framework, can be avoided altogether, this way eliminating the need for experts’ input. In this study, we apply Bayesian nonparametric statistics to develop a comprehensive mathematical framework for automated peak identification and characterization. Our approach relies on advanced statistical representations of FIDs and allows for: simultaneous identification and characterization of individual peaks in an NMR spectrum, accurate noise deconvolution, and extensions to multidimensional NMR or incorporation of specialized protocols. We show that our methods can correctly identify and characterize overlapping peaks without user input. We benchmark our analyses against ground truth peak characteristics using synthetic as well as experimental datasets.
更多查看译文
关键词
bayesian nonparametric statistical learning,nmr data
AI 理解论文
溯源树
样例
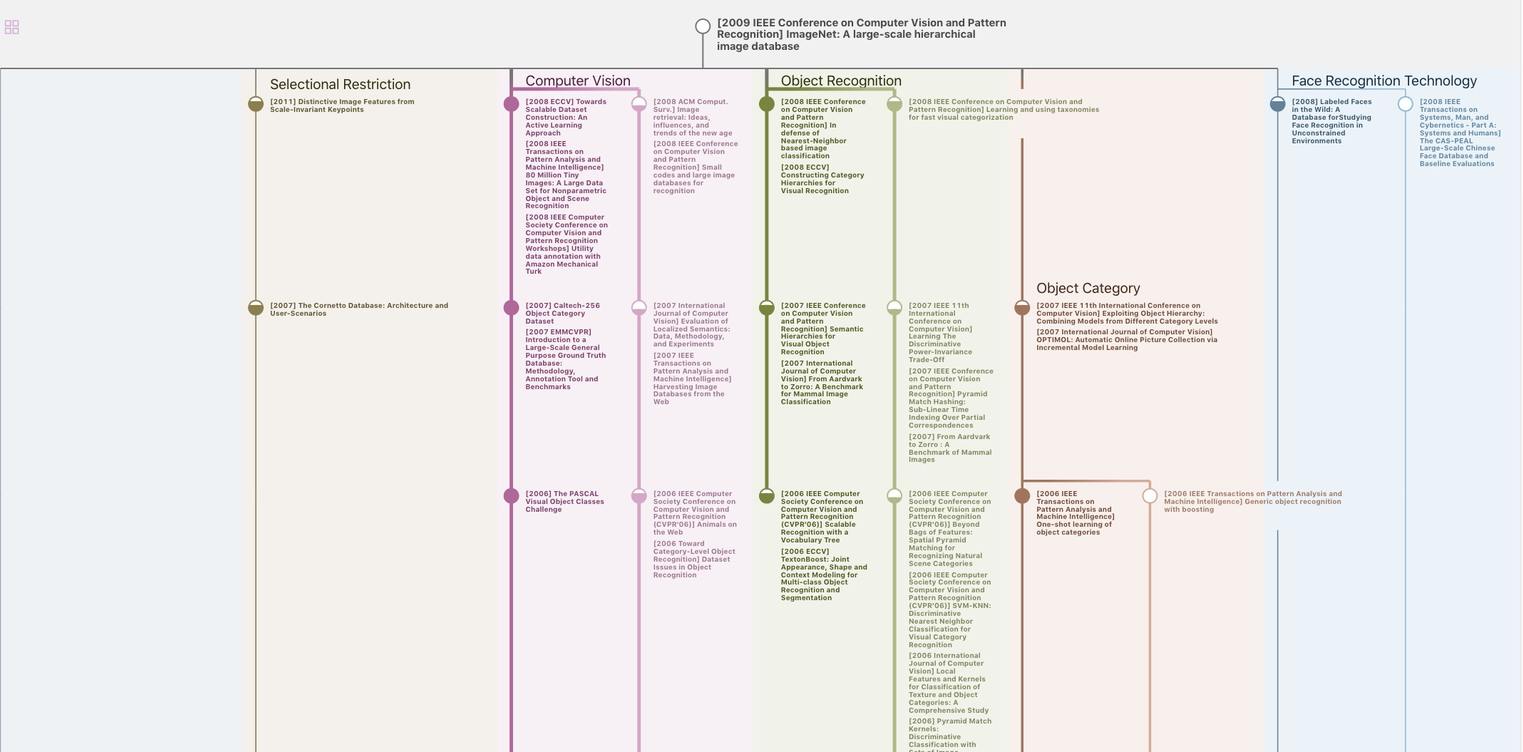
生成溯源树,研究论文发展脉络
Chat Paper
正在生成论文摘要