Dynamics-based Network Analysis Identifies Laci Rheostats with High Accuracy
BIOPHYSICAL JOURNAL(2023)
摘要
One current challenge in protein engineering involves accurately predicting distal mutations which lead towards a desired function. A more complex problem is identifying and characterizing putative “rheostat” sites which result in progressive, amino acid-dependent biophysical changes upon substitution. The E. coli LacI system contains several rheostat positions with respect to the binding of LacI inducer IPTG, which allosterically inhibits DNA binding. Here we employ in-house computational tools for characterizing network dynamics: Site-specific flexibility via the Dynamic Flexibility Index (DFI), residue pair coupling strength via the Dynamic Coupling Index (DCI), and Vibrational Density of States (VDOS) analysis to generate measures of position-specific dynamic-based metrics. Comparison of bound and unbound LacI shows specific regions of each system exhibit fundamentally different flexibilities where critical DNA binding domain positions show increased flexibility when bound to IPTG. Further, we assess asymmetry in dynamic coupling as a cause-effect measure between ligands/ligand binding positions and all other positions within the protein. Additionally, it is possible to calculate positional vibrational entropy through VDOS; this analysis, again, showed distinctions between bound and unbound simulations in both the DNA binding domain as well as the IPTG binding region. Taken together, the drastic differences of these dynamics-based metrics between bound and unbound conformations indicate their strength in construction unique dynamical fingerprints for both systems. Using a machine learning approach, we construct features based off of these dynamic properties from which we extract probabilities of a given position being “neutral”, “toggle” (i.e., abolishing biophysical activity upon mutation) or “rheostat” based upon experimental IPTG EC50 data of IPTG binding. Using a small subset of dynamic network features, we show it is possible to categorize, with accuracy rates of ∼0.9, a given position's probability of falling into one of the three above categories.
更多查看译文
关键词
Cell Signaling Dynamics
AI 理解论文
溯源树
样例
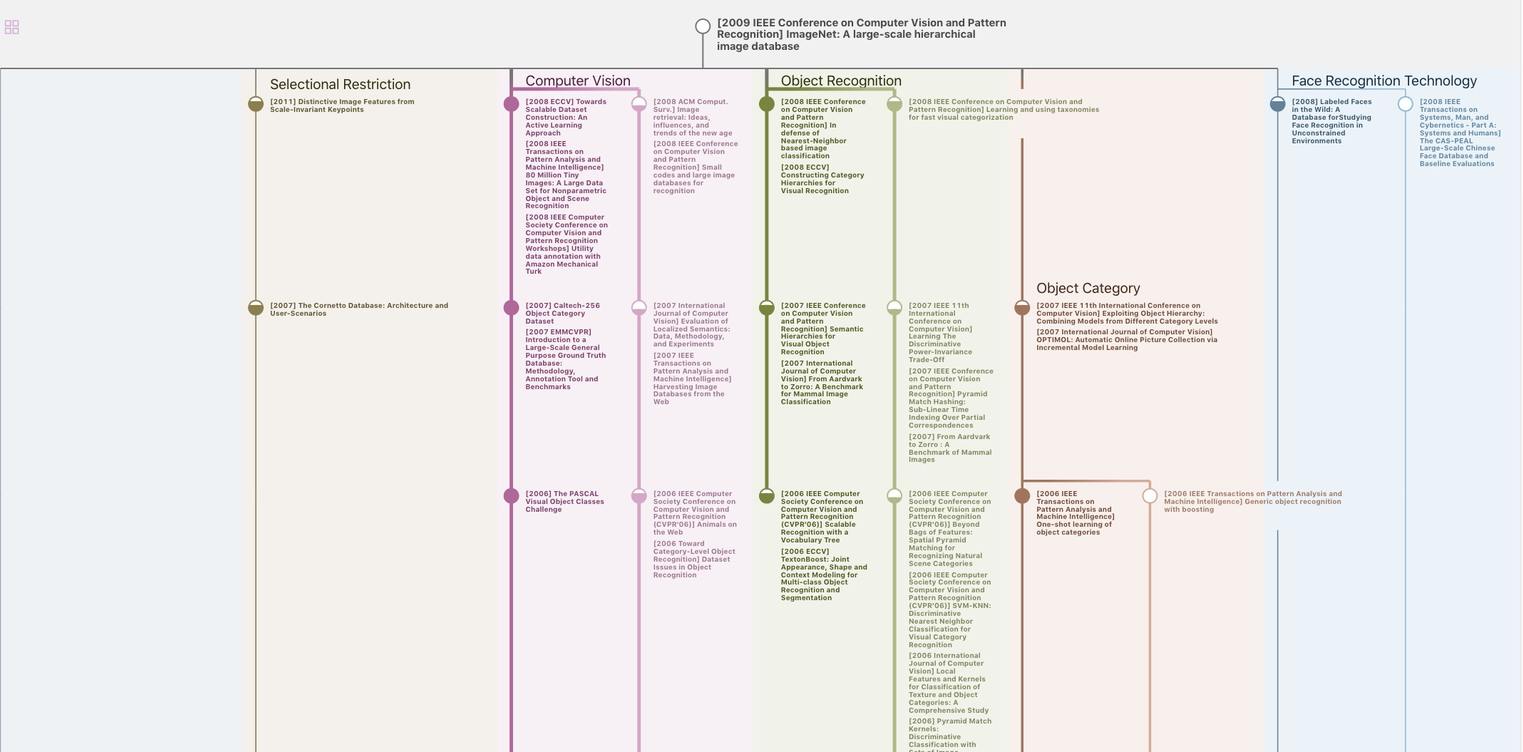
生成溯源树,研究论文发展脉络
Chat Paper
正在生成论文摘要