Machine learning guided sampling of protein transition pathways
Biophysical Journal(2023)
摘要
Capturing a protein conformational transition pathway is an important yet difficult task in biophysics. For example, X-ray-crystallography/cryo-EM techniques have captured open/closed states or inward/outward-facing conformations of many ion-channels/transporters; yet, it remains a challenge to experimentally capture transitioning pathways between these ground states due to their rather short-spanned nature. Multiple advanced techniques based on molecular dynamics (MD) simulations have provided great insights to fill such gaps by predicting hypothetical transition states and pathways. Such MD-based techniques, including both long equilibrium simulations and enhanced sampling ones, have provided insights to better understand how putative transition state conformations and pathways would look like. However, MD-based techniques generally take a long time; therefore, it would be of great utility to sample putative transition states along putative transition pathways more efficiently. We present a machine learning model, developed using variational autoencoder (VAE), with the goal of sampling the transition pathway between different protein's conformational states. Using VAE, which takes in protein raw trajectory coordinates generated by equilibrium MD simulations as input, the model is able to reconstruct a structurally close output. Such a VAE can therefore reduce the high-dimensional protein description in the full space into a meaningful low-dimensional representation. In this low-dimensional space, we can interpolate between the two states of interest. The interpolated pathway represents a putative transition-pathway in the higher-dimensional space, learned by VAE. After reconstruction of the sampled transition pathway into the full protein space, secondary structures, RMSDs, and other quality-control properties are used and compared with the functional mechanism of the protein. The reconstructed putative transition pathway can be used in further MD simulations. This machine learning guided MD approach will accelerate studying the full transition pathways of protein conformations by seeding good starting points generated by VAE for iterative MD simulations.
更多查看译文
关键词
protein transition pathways,sampling,learning
AI 理解论文
溯源树
样例
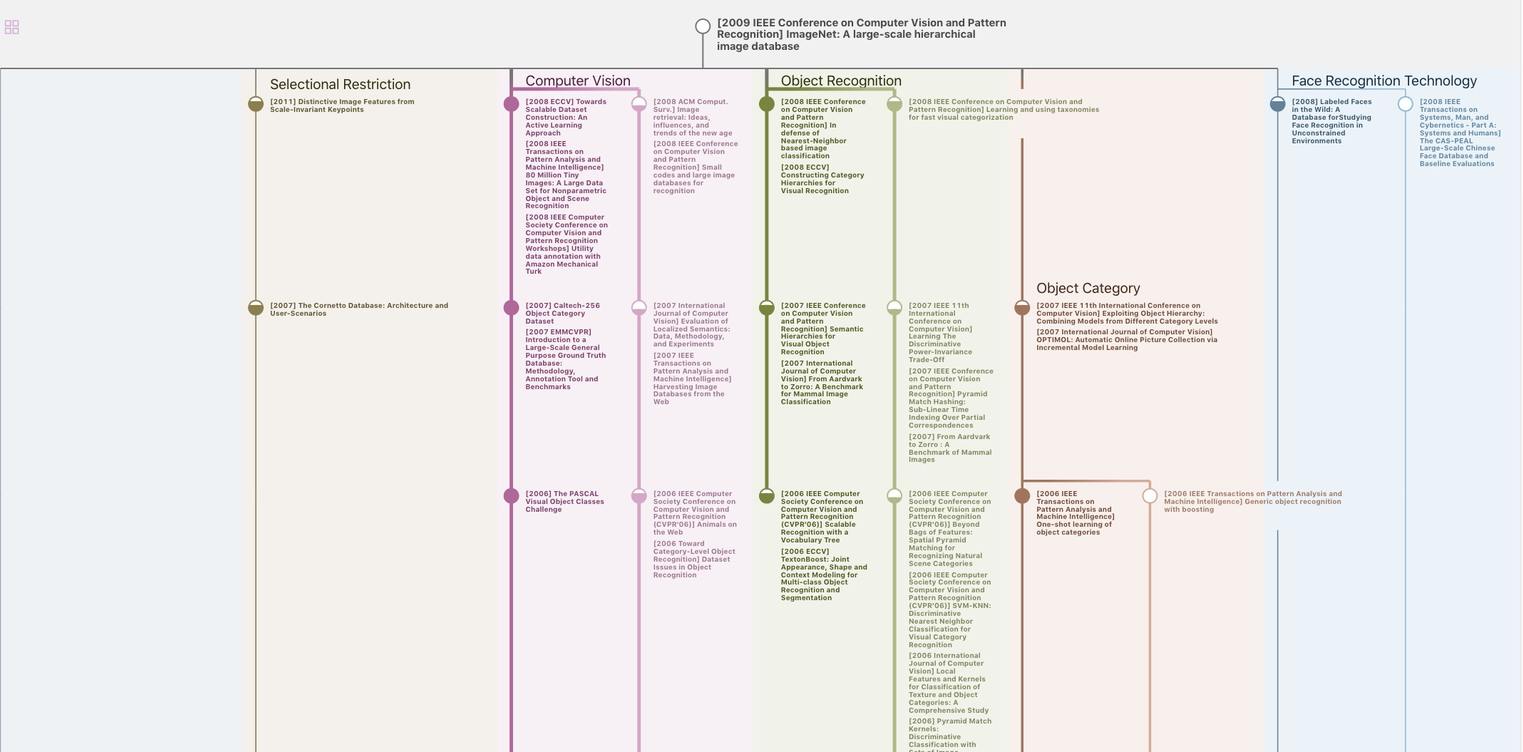
生成溯源树,研究论文发展脉络
Chat Paper
正在生成论文摘要