HPKNN: Hyper-parameter optimized KNN classifier for classification of poikilocytosis
INTERNATIONAL JOURNAL OF IMAGING SYSTEMS AND TECHNOLOGY(2023)
摘要
Red blood cells (RBCs) are the more crucial components of a healthy organism. Poikilocytosis is a disorder in which blood cells lose their standard shapes. The human bodies are affected by disorders like anemia and thalassemia because the structure of RBCs shape changes. Hematologists take longer time to detect abnormalities in RBCs. The proposed hyper-parameter optimizable K nearest neighbor (HPKNN) algorithm detects and classifies numerous types of poikilocytosis quickly and accurately. On the Chula-PIC-Lab and Erythrocytes IDB datasets, the proposed HPKNN algorithm was tested. After the preprocessing stage, the Freeman chain code has been utilized to retrieve the exact boundary shape of RBC morphology. On datasets of Erythrocytes IDB 1, 2, 3, and Chula-PIC-Lab(Chula-RBC-12), the morphological tools and Freeman chain code based segmentation provide 97.44% and 94.45% accuracy, respectively, which is superior than other methods. The proposed HPKNN classifier has an overall sensitivity of 97.9%, precision of 97.9%, and an f(1) score of 97.9% on the Chula-PIC-Lab (Chula-RBC-12) dataset to classify 10 classes, namely acanthocyte, dacrocyte, echinocyte, elliptocyte, normal, overlapped, schistocyte, spherocyte, stomatocyte, and target cell, which is greater than any other current approach. The HPKNN classifier achieves 98.085% precision, 98.085% sensitivity or recall, and 98.085% f(1)-score values on the Erythrocytes IDB datasets to classify circular, elongated, and other RBC classes.
更多查看译文
关键词
anemia,Freeman chain code,KNN classifier,poikilocytosis
AI 理解论文
溯源树
样例
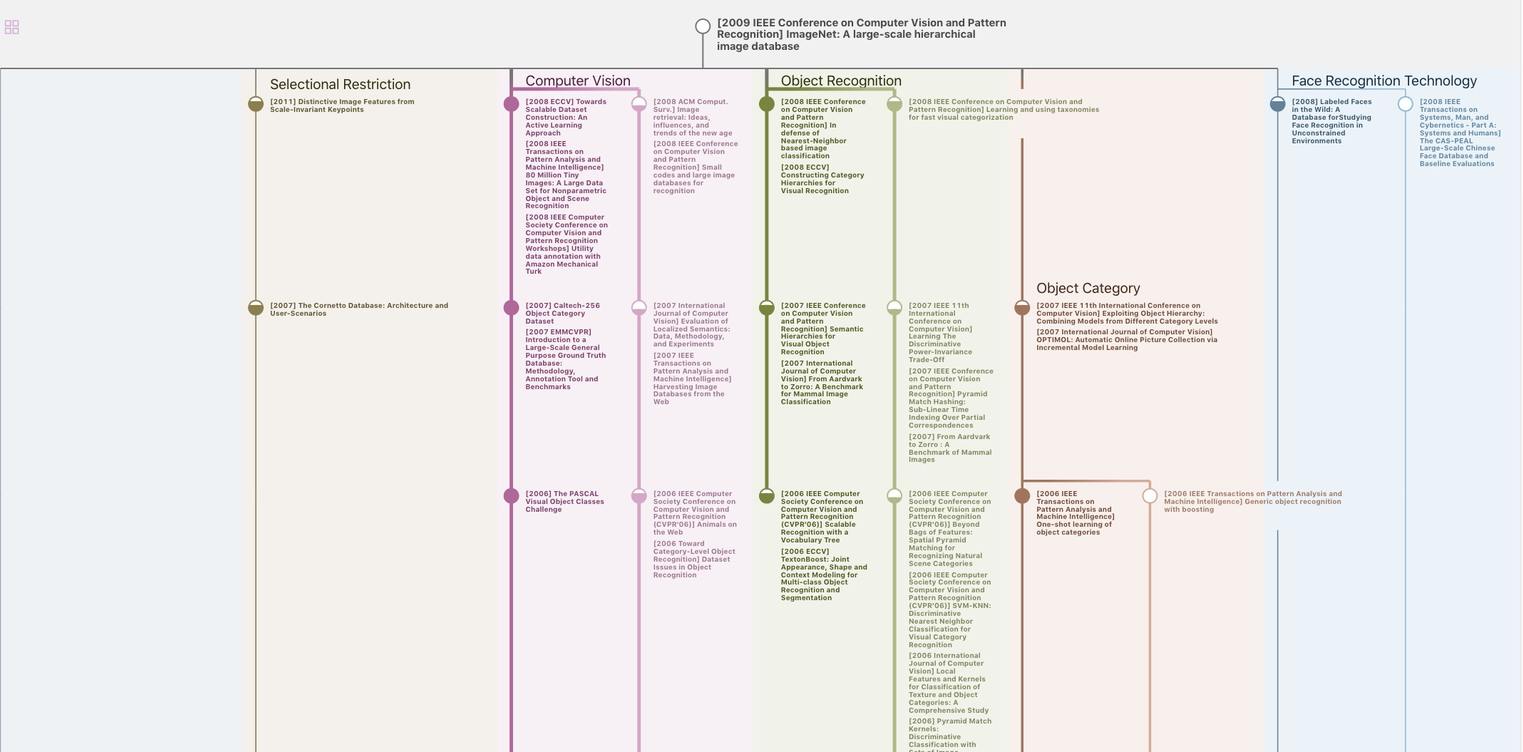
生成溯源树,研究论文发展脉络
Chat Paper
正在生成论文摘要