Multiscale Backcast Convolution Neural Network for Traffic Flow Prediction in The Frequency Domain
APPLIED SCIENCES-BASEL(2022)
摘要
With the construction of intelligent transportation systems in recent years, intelligent methods for the prediction of traffic flow are becoming more and more important, and accurate prediction plays a key role in enabling downstream scheduling algorithms. However, the accuracy of most current forecasting algorithms remains unsatisfactory. Because traffic depends on the time of the day and varies throughout the week, such as during peak commuting periods as opposed to other times, traffic flow data show evident cyclical patterns. We capitalize on this notion and propose a multiscale convolutional feedback network for frequency prediction based on frequency angle. We combine multiscale convolution (MSC) with dilated convolution, and increase the convolutional receptive field by expanding cavity size while retaining similar parameterization costs, and achieve multiscale convolution with kernels referring to different receptive fields. At the same time, we incorporate an autoencoding module by assigning the same set of hidden features to input reconstruction and output prediction, which results in enhanced stability of features within the hidden layers. When we tested our approach on the Traffic dataset, our model achieved the best performance as assessed via the three indicators measured using mean squared error (MSE), mean absolute error (MAE), and correlation coefficient (CORR), with improvements of 3.818%, 2.472% and, 0.1515%, respectively.
更多查看译文
关键词
traffic flow prediction,time series,convolutional neural networks,auto-encoder,intelligent transportation systems
AI 理解论文
溯源树
样例
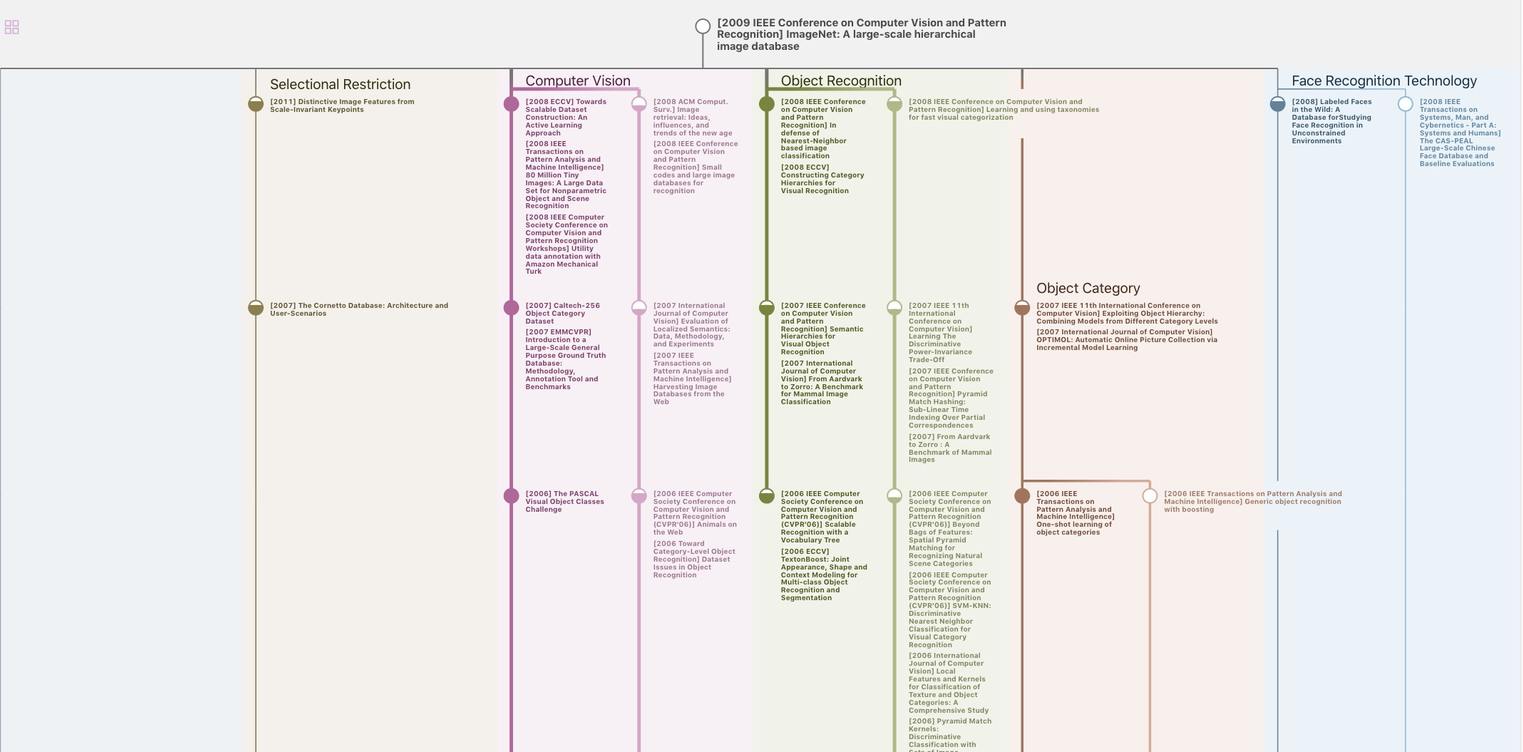
生成溯源树,研究论文发展脉络
Chat Paper
正在生成论文摘要