A Random Projection Approach to Hypothesis Tests in High-Dimensional Single-Index Models
JOURNAL OF THE AMERICAN STATISTICAL ASSOCIATION(2023)
摘要
In this article, we consider the problem of hypothesis testing in high-dimensional single-index models. First, we study the feasibility of applying the classical F-test to a single-index model when the dimension of covariate vector and sample size are of the same order, and derive its asymptotic null distribution and asymptotic local power function. For the ultrahigh-dimensional single-index model, we construct F-statistics based on lower-dimensional random projections of the data, and establish the asymptotic null distribution and the asymptotic local power function of the proposed test statistics for the hypothesis testing of global and partial parameters. The new proposed test possesses the advantages of having a simple structure as well as being easy to compute. We compare the proposed test with other high-dimensional tests and provide sufficient conditions under which the proposed tests are more efficient. We conduct simulation studies to evaluate the finite-sample performances of the proposed tests and demonstrate that it has higher power than some existing methods in the models we consider. The application of real high-dimensional gene expression data is also provided to illustrate the effectiveness of the method. for this article are available online.
更多查看译文
关键词
High-dimensional inference,Hypothesis testing,Random projection,Single-index model
AI 理解论文
溯源树
样例
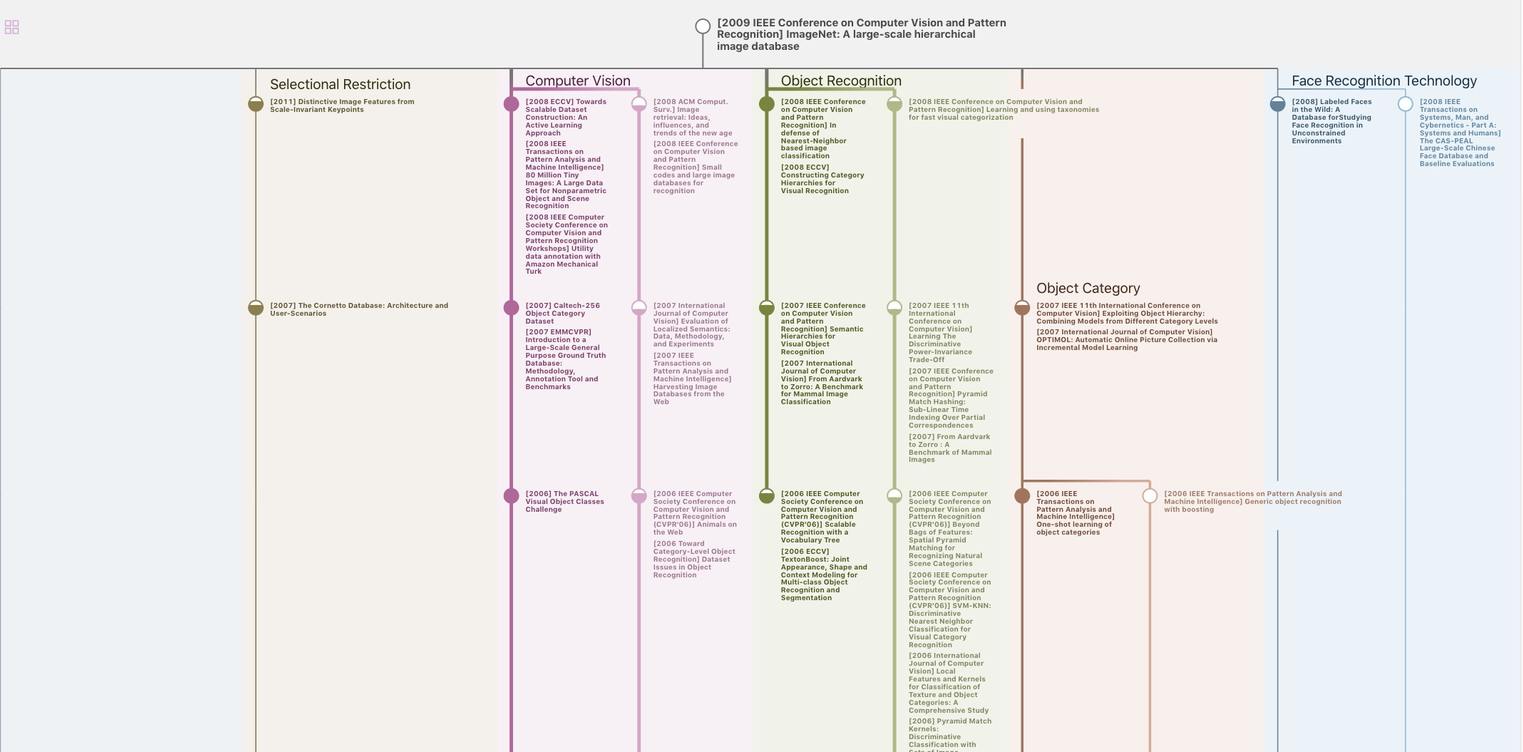
生成溯源树,研究论文发展脉络
Chat Paper
正在生成论文摘要