One-Shot Domain-Adaptive Imitation Learning via Progressive Learning Applied to Robotic Pouring
IEEE TRANSACTIONS ON AUTOMATION SCIENCE AND ENGINEERING(2024)
摘要
Traditional deep learning-based visual imitation learning techniques require a large amount of demonstration data for model training, and the pre-trained models are difficult to adapt to new scenarios. To address these limitations, we propose a unified framework using a novel progressive learning approach comprised of three phases: i) a coarse learning phase for concept representation, ii) a fine learning phase for action generation, and iii) an imaginary learning phase for domain adaptation. Overall, this approach leads to a one-shot domain-adaptive imitation learning framework. We use robotic pouring as an example task to evaluate its effectiveness. Our results show that the method has several advantages over contemporary end-to-end imitation learning approaches, including an improved success rate for task execution and more efficient training for deep imitation learning. In addition, the generalizability to new domains is improved, as demonstrated here with novel backgrounds, target containers, and granule combinations in the experiment. We believe that the proposed method is broadly applicable to various industrial or domestic applications that involve deep imitation learning for robotic manipulation, and where the target scenarios are diverse and human demonstration data is limited. For project video, please check our website.
更多查看译文
关键词
Efficient robot skill learning,imitation learning,robot and automation,one-shot learning,robotic pouring
AI 理解论文
溯源树
样例
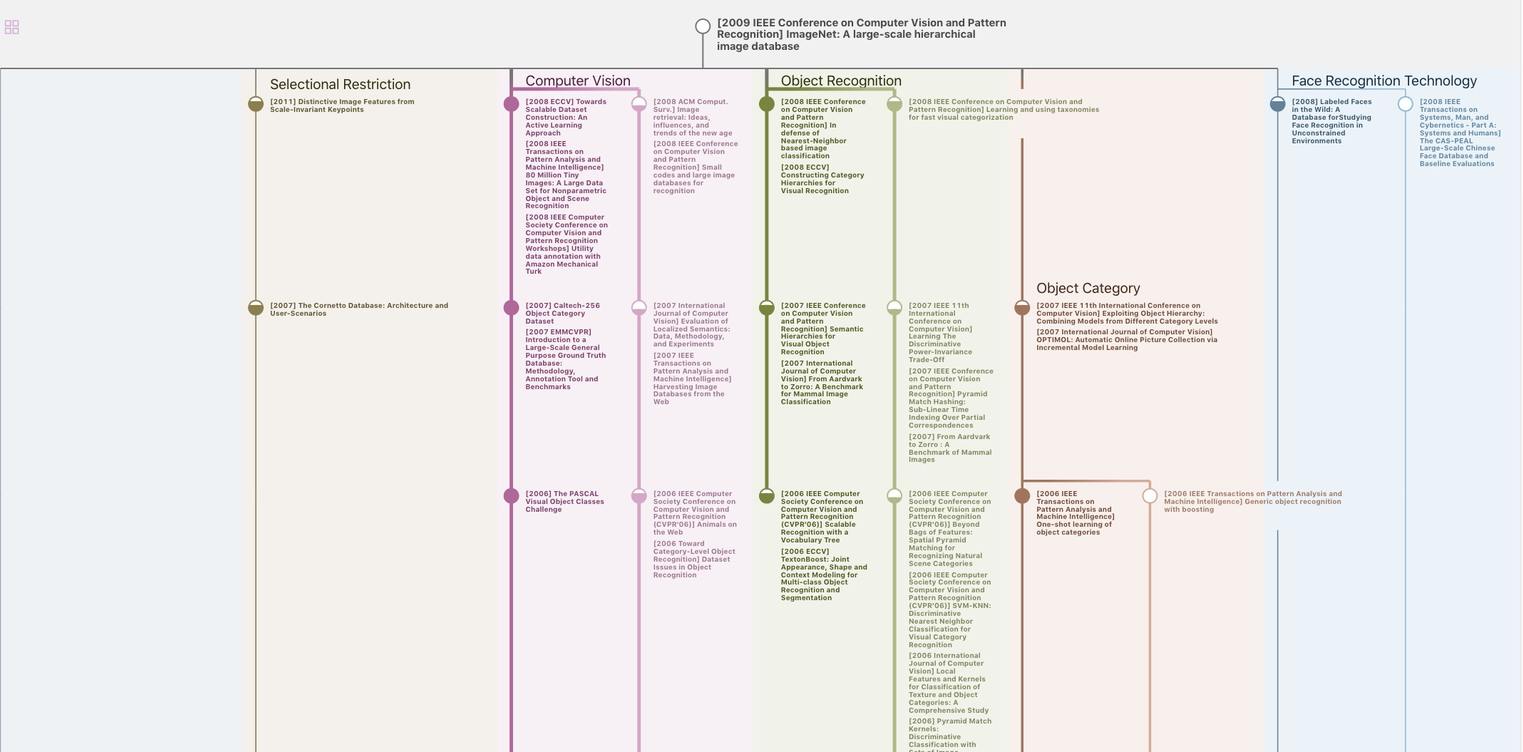
生成溯源树,研究论文发展脉络
Chat Paper
正在生成论文摘要