Safety-critical control for robotic systems with uncertain model via control barrier function
INTERNATIONAL JOURNAL OF ROBUST AND NONLINEAR CONTROL(2023)
摘要
Usually, it is difficult to build accurate dynamic models for real robots, which makes safety-critical control a challenge. In this regard, this article proposes a double-level framework to design safety-critical controller for robotic systems with uncertain dynamics. The high level planner plans a safe trajectory for low level tracker based on the control barrier function (CBF). First, the high level planning is done independently of the dynamic model by quadratic programs subject to CBF constraint. Afterward, a novel method is proposed to learn the uncertainty of drift term and input gain in nonlinear affine-control system by a data-driven Gaussian process (GP) approach, in which the learning result of uncertainty in input gain is associated with CBF. Then, a Gaussian processes-based control barrier function (GP-CBF) is designed to guarantee the tracking safety with a lower bound on the probability for the low level tracker. Finally, the effectiveness of the proposed framework is verified by the numerical simulation of UR3 robot.
更多查看译文
关键词
control barrier function,Gaussian processes,robotic system,safety-critical control,uncertainty
AI 理解论文
溯源树
样例
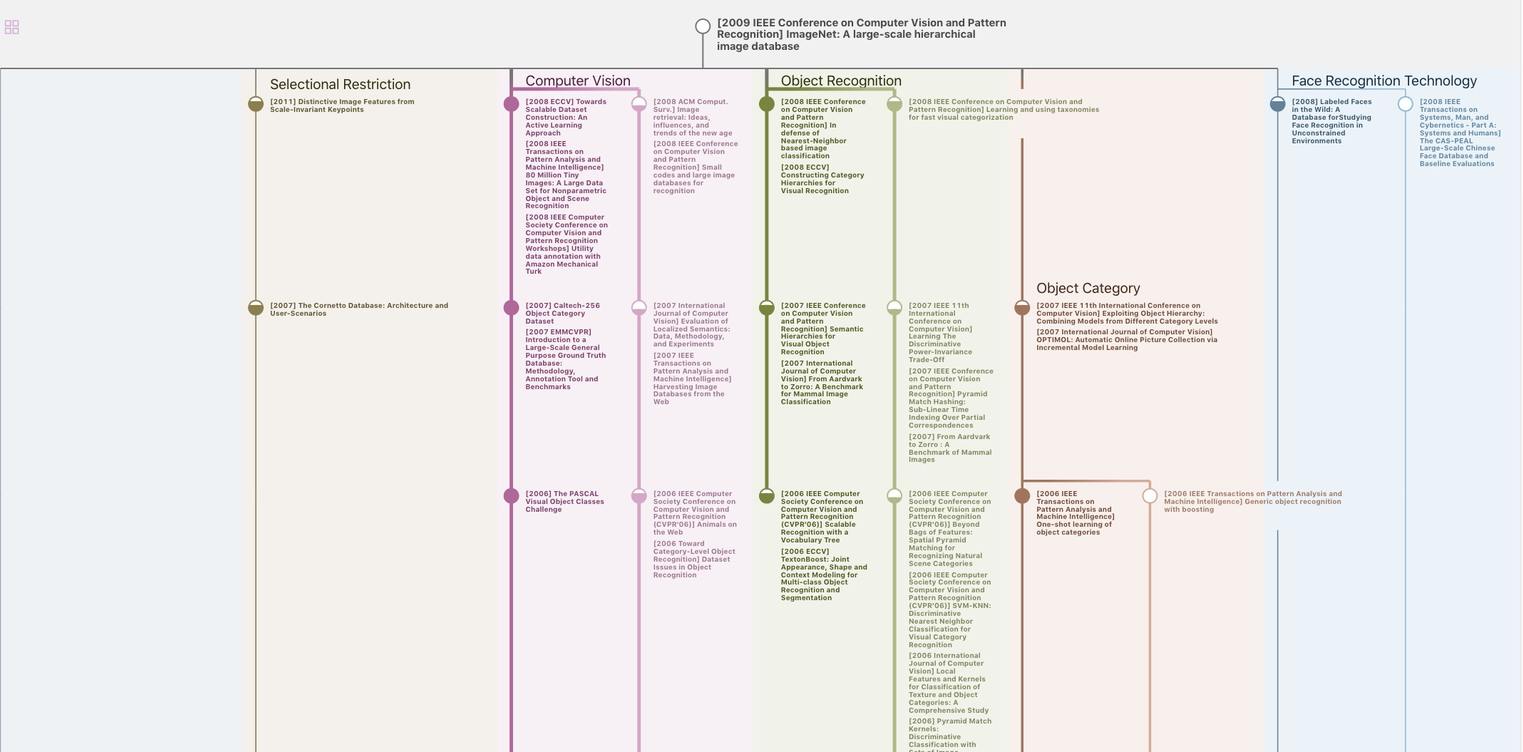
生成溯源树,研究论文发展脉络
Chat Paper
正在生成论文摘要