Modeling and Prediction of Environmental Factors and Chlorophyll a Abundance by Machine Learning Based on Tara Oceans Data
JOURNAL OF MARINE SCIENCE AND ENGINEERING(2022)
摘要
It is of great theoretical and practical significance to understand the inherent relationship and evolution patterns among various environmental factors in the oceans. In this study, we used scientific data obtained by the Tara Oceans Project to conduct a comprehensive correlation analysis of marine environmental factors. Using artificial intelligence and machine learning methods, we evaluated different methods of modeling and predicting chlorophyll a (Chl-alpha) concentrations at the surface water layer of selected Tara Oceans data after the raw data processing. Then, a Pearson correlation and characteristic importance analysis between marine environmental factors and the Chl-alpha concentrations was conducted, and thus a comprehensive correlation model for environmental factors was established. With these obtained data, we developed a new prediction model for the Chl-alpha abundance based on the eXtreme Gradient Boosting (XGBoost) algorithm with intelligent parameter optimization strategy. The proposed model was used to analyze and predict the abundance of Chl-a abundance of TOP. The obtained predicted results were also compared with those by using other three widely-used machine learning methods including the random forest (RF), support vector regression (SVR) and linear regression (LR) algorithms. Our results show that the proposed comprehensive correlation evaluation model can identify the effective features closely related to Chl-alpha, abundance, and the prediction model can reveal the potential relationship between environmental factors and the Chl-alpha concentrations in the oceans.
更多查看译文
关键词
comprehensive correlation model,multi-environmental factors prediction model,chlorophyll a,machine learning,Tara Oceans data,XGBoost
AI 理解论文
溯源树
样例
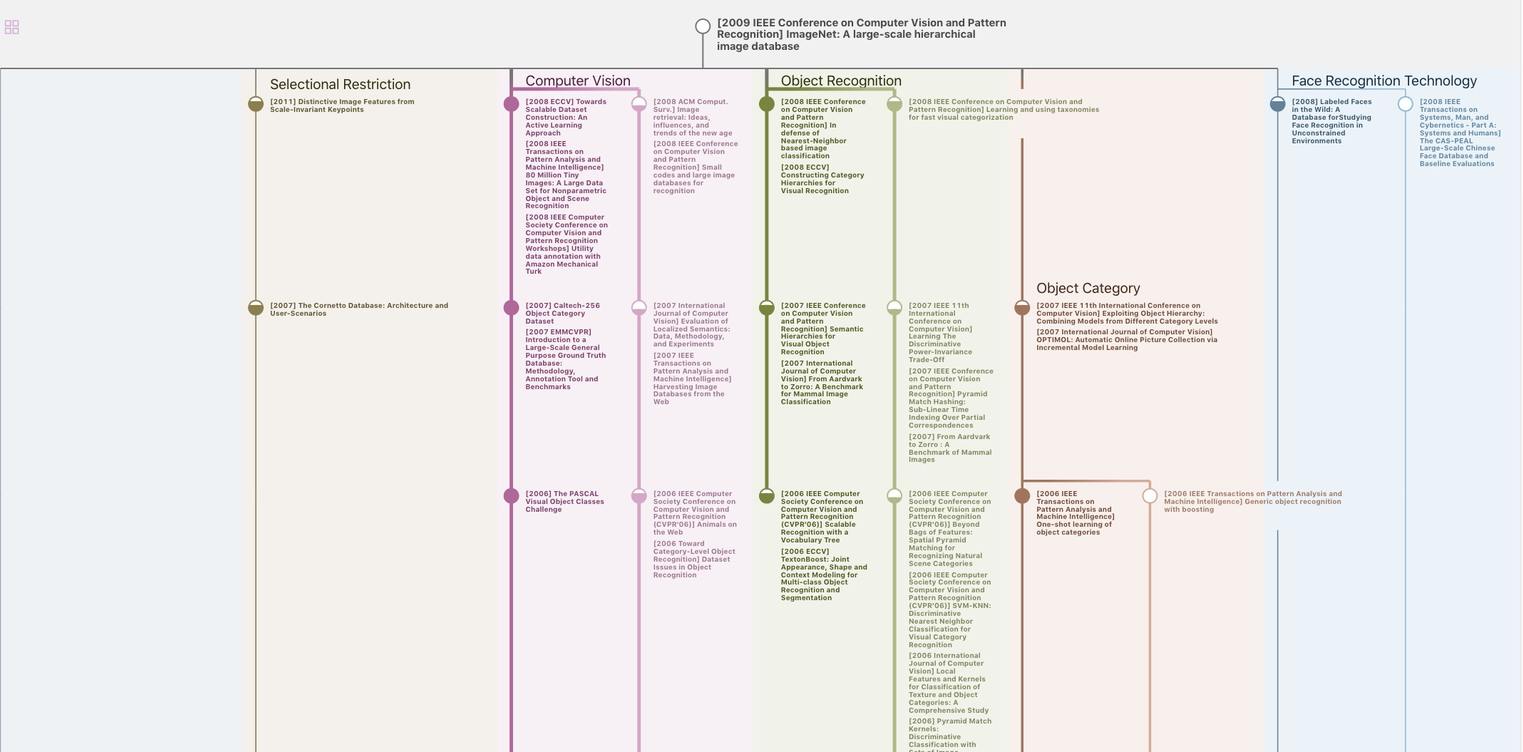
生成溯源树,研究论文发展脉络
Chat Paper
正在生成论文摘要