Comparative analysis of deep and machine learning approaches for daily carbon monoxide pollutant concentration estimation
INTERNATIONAL JOURNAL OF ENVIRONMENTAL SCIENCE AND TECHNOLOGY(2022)
摘要
Air pollution is an increasingly critical global issue around the world largely attributed to rapid industrial development, the rise in the world’s population, and the ever increasing number of cars on the roads. Air pollution is a serious health risk and environmental concerns in many countries. Turkey has seen major industrial development in recent years which has led to increasing levels of air pollution. In this study, the air pollution levels of Sakarya, a major industrial city in Turkey, is analysed by calculating and modelling daily carbon monoxide ( CO) concentrations. The CO values are calculated using deep learning (Long short-term memory (LSTM) and bidirectional long short-term memory (Bi-LSTM)) models. These models are benchmarked against machine learning (Artificial neural networks (ANN), k-nearest neighbours (kNN), and support vector regression (SVR)) methods. The daily antecedent values of observed CO and PM 10 parameters were used as inputs. Considering the correlation between these parameters, 6 different input scenarios with maximum 3-day delays were created and the daily CO values were calculated. The results showed that the LSTM and Bi-LSTM models were the most effective with a correlation coefficient of R = 0.91, then the kernel-based SVR was ranked with a correlation coefficient of R = 0.88. Among the 6 scenarios used, the scenario whereby the inputs were 3-lag time with CO values and one-lag delay of PM 10 values was found to be the most effective. The deep learning methods have been observed to give reliable results in air pollution modelling.
更多查看译文
关键词
Air pollution,CO,PM10,Deep learning,Machine learning
AI 理解论文
溯源树
样例
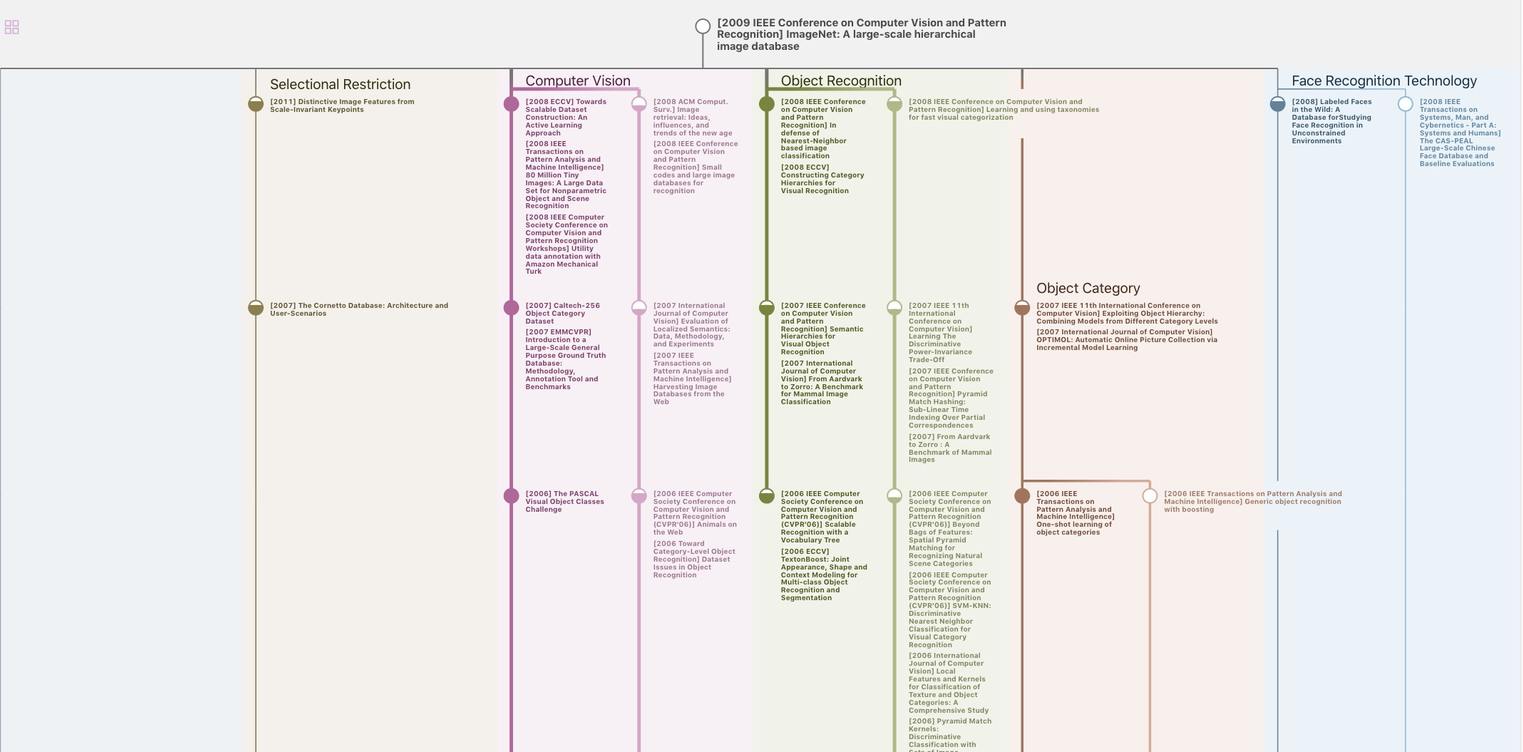
生成溯源树,研究论文发展脉络
Chat Paper
正在生成论文摘要