Optimal Subsampling Bootstrap for Massive Data
JOURNAL OF BUSINESS & ECONOMIC STATISTICS(2024)
摘要
The bootstrap is a widely used procedure for statistical inference because of its simplicity and attractive statistical properties. However, the vanilla version of bootstrap is no longer feasible computationally for many modern massive datasets due to the need to repeatedly resample the entire data. Therefore, several improvements to the bootstrap method have been made in recent years, which assess the quality of estimators by subsampling the full dataset before resampling the subsamples. Naturally, the performance of these modern subsampling methods is influenced by tuning parameters such as the size of subsamples, the number of subsamples, and the number of resamples per subsample. In this article, we develop a novel hyperparameter selection methodology for selecting these tuning parameters. Formulated as an optimization problem to find the optimal value of some measure of accuracy of an estimator subject to computational cost, our framework provides closed-form solutions for the optimal hyperparameter values for subsampled bootstrap, subsampled double bootstrap and bag of little bootstraps, at no or little extra time cost. Using the mean square errors as a proxy of the accuracy measure, we apply our methodology to study, compare and improve the performance of these modern versions of bootstrap developed for massive data through numerical study. The results are promising.
更多查看译文
关键词
Bag of little bootstraps,Bootstrap,Computational cost,Subsampled double bootstrap,Subsampling
AI 理解论文
溯源树
样例
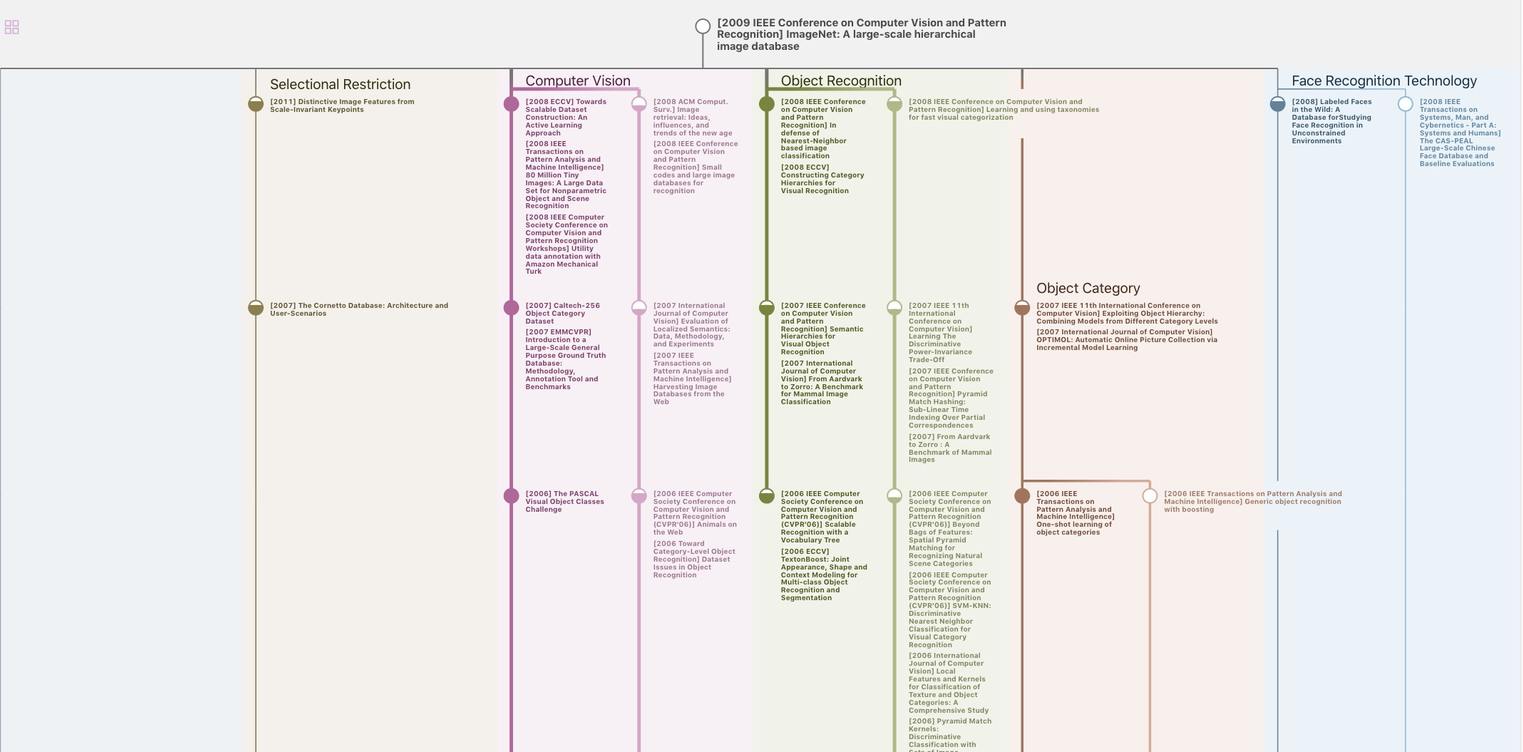
生成溯源树,研究论文发展脉络
Chat Paper
正在生成论文摘要