Towards Optimal Compression: Joint Pruning and Quantization
arxiv(2023)
摘要
Compression of deep neural networks has become a necessary stage for optimizing model inference on resource-constrained hardware. This paper presents FITCompress, a method for unifying layer-wise mixed precision quantization and pruning under a single heuristic, as an alternative to neural architecture search and Bayesian-based techniques. FITCompress combines the Fisher Information Metric, and path planning through compression space, to pick optimal configurations given size and operation constraints with single-shot fine-tuning. Experiments on ImageNet validate the method and show that our approach yields a better trade-off between accuracy and efficiency when compared to the baselines. Besides computer vision benchmarks, we experiment with the BERT model on a language understanding task, paving the way towards its optimal compression.
更多查看译文
AI 理解论文
溯源树
样例
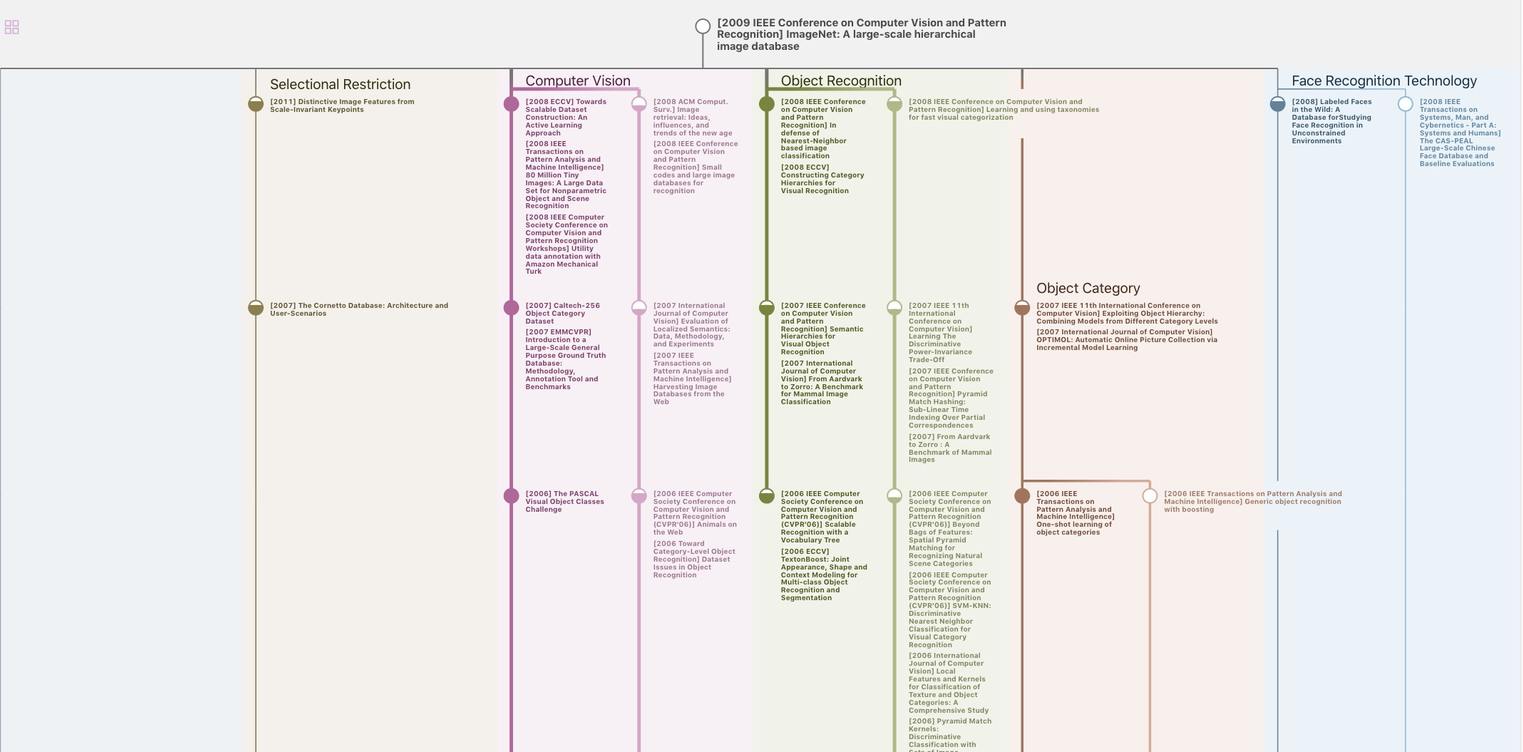
生成溯源树,研究论文发展脉络
Chat Paper
正在生成论文摘要