Denoising Diffusion Probabilistic Models for Robust Image Super-Resolution in the Wild
arxiv(2023)
摘要
Diffusion models have shown promising results on single-image super-resolution and other image- to-image translation tasks. Despite this success, they have not outperformed state-of-the-art GAN models on the more challenging blind super-resolution task, where the input images are out of distribution, with unknown degradations. This paper introduces SR3+, a diffusion-based model for blind super-resolution, establishing a new state-of-the-art. To this end, we advocate self-supervised training with a combination of composite, parameterized degradations for self-supervised training, and noise-conditioing augmentation during training and testing. With these innovations, a large-scale convolutional architecture, and large-scale datasets, SR3+ greatly outperforms SR3. It outperforms Real-ESRGAN when trained on the same data, with a DRealSR FID score of 36.82 vs. 37.22, which further improves to FID of 32.37 with larger models, and further still with larger training sets.
更多查看译文
关键词
denoising,robust image
AI 理解论文
溯源树
样例
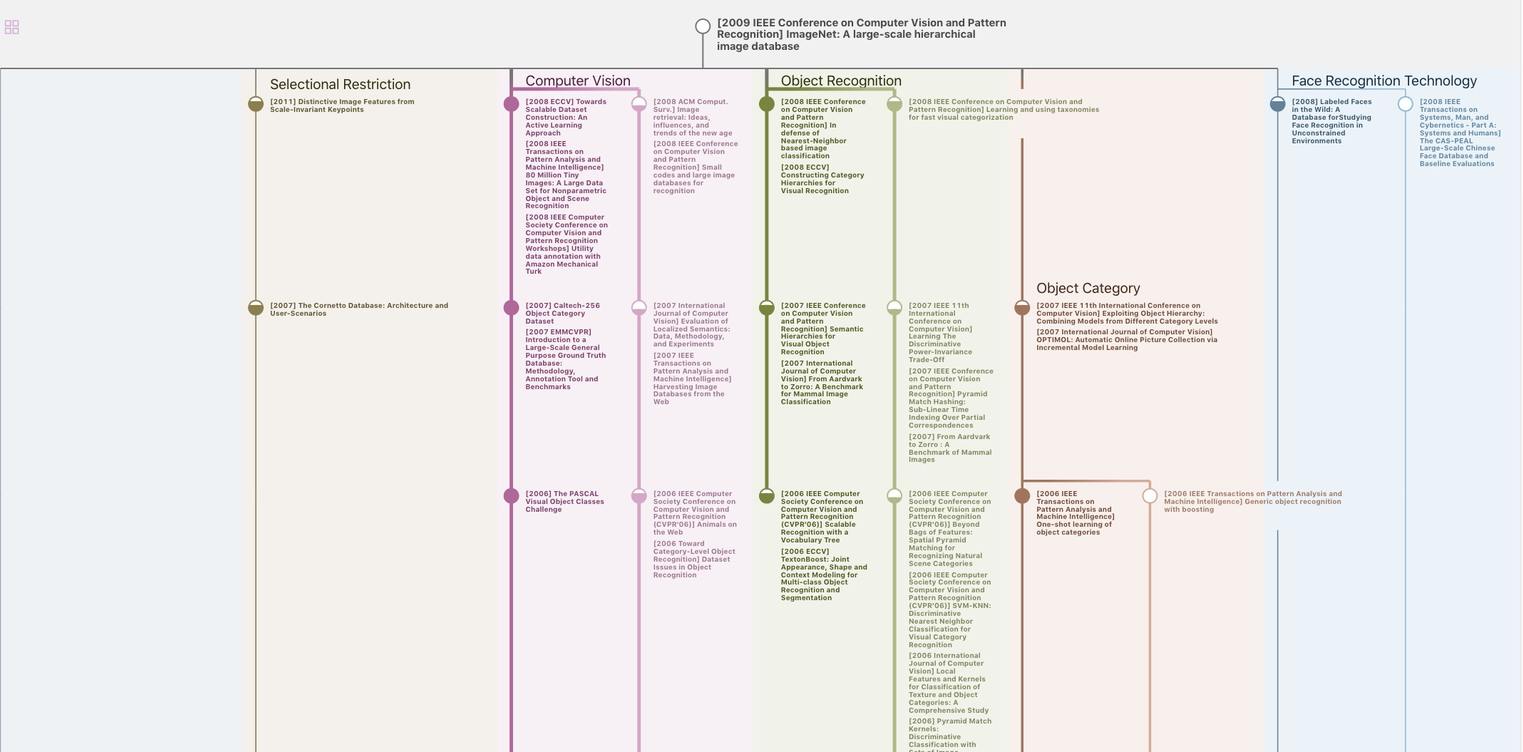
生成溯源树,研究论文发展脉络
Chat Paper
正在生成论文摘要