Using graph neural networks for site-of-metabolism prediction and its applications to ranking promiscuous enzymatic products
BIOINFORMATICS(2023)
摘要
Motivation: While traditionally utilized for identifying site-specific metabolic activity within a compound to alter its interaction with a metabolizing enzyme, predicting the site-of-metabolism (SOM) is essential in analyzing the promiscuity of enzymes on substrates. The successful prediction of SOMs and the relevant promiscuous products has a wide range of applications that include creating extended metabolic models (EMMs) that account for enzyme promiscuity and the construction of novel heterologous synthesis pathways. There is therefore a need to develop generalized methods that can predict molecular SOMs for a wide range of metabolizing enzymes.Results: This article develops a Graph Neural Network (GNN) model for the classification of an atom (or a bond) being an SOM. Our model, GNN-SOM, is trained on enzymatic interactions, available in the KEGG database, that span all enzyme commission numbers. We demonstrate that GNN-SOM consistently outperforms baseline machine learning models, when trained on all enzymes, on Cytochrome P450 (CYP) enzymes, or on non-CYP enzymes. We showcase the utility of GNN-SOM in prioritizing predicted enzymatic products due to enzyme promiscuity for two biological applications: the construction of EMMs and the construction of synthesis pathways.
更多查看译文
关键词
ranking promiscuous enzymatic products,graph neural networks,site-of-metabolism
AI 理解论文
溯源树
样例
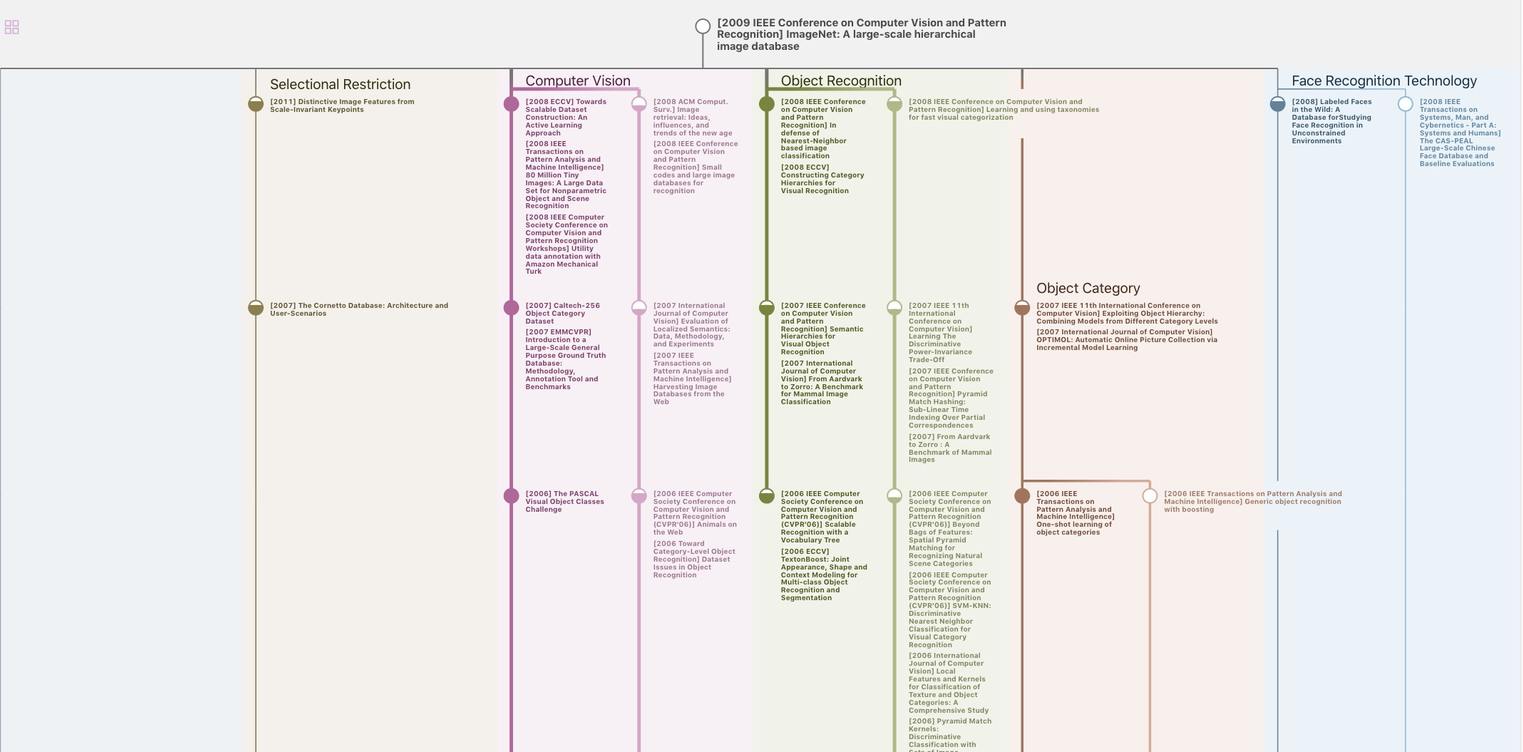
生成溯源树,研究论文发展脉络
Chat Paper
正在生成论文摘要