Multilingual Document Concept Topic Modeling
2022 European Conference on Natural Language Processing and Information Retrieval (ECNLPIR)(2022)
摘要
Probabilistic topic models have demonstrated a high level of success in mining and analyzing topics from data generated. According to the previous study every topic is denoted as a probability distribution over words. This theory remains unsatisfactory; existing topic models do not implement well when it comes to extracting cross-lingual topics from simple words in different languages. Here, we present a novel framework for extracting topics from document collections. Knowledge is transferred to a cross-lingual model by incorporating a concept layer between the topic and word layers, with the ultimate goal of simplifying the process of extracting shared topics in text data across languages. In particular, we propose a novel Multilingual Document Concept Topic Modeling Method (MDCTM). Training algorithm models based on Gibbs sampling was used to develop MDCTM. Two datasets were evaluated. Using jieba for word segmentation, we demonstrate that the MDCTM model can effectively extract concept topic models from multilingual text data. Furthermore, our proposed model is presented to achieve state-of-the-art performance.
更多查看译文
关键词
Topic models,concept topic models,bilingual topic models,multilingual
AI 理解论文
溯源树
样例
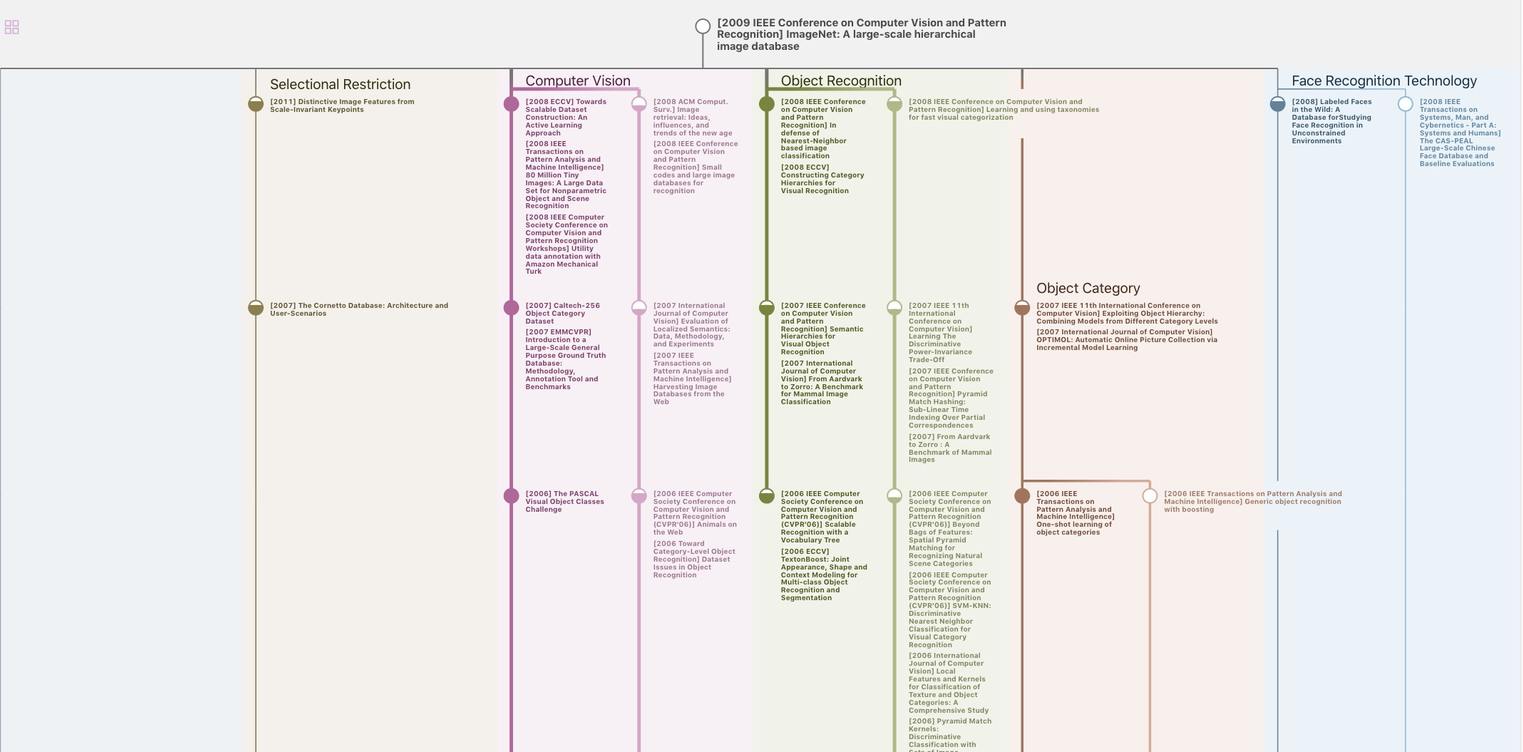
生成溯源树,研究论文发展脉络
Chat Paper
正在生成论文摘要