Detecting and approximating decision boundaries in low dimensional spaces
CoRR(2023)
摘要
A method for detecting and approximating fault lines or surfaces, respectively, or decision curves in two and three dimensions with guaranteed accuracy is presented. Reformulated as a classification problem, our method starts from a set of scattered points along with the corresponding classification algorithm to construct a representation of a decision curve by points with prescribed maximal distance to the true decision curve. Hereby, our algorithm ensures that the representing point set covers the decision curve in its entire extent and features local refinement based on the geometric properties of the decision curve. We demonstrate applications of our method to problems related to the detection of faults, to Multi-Criteria Decision Aid and, in combination with Kirsch's factorization method, to solving an inverse acoustic scattering problem. In all applications we considered in this work, our method requires significantly less pointwise classifications than previously employed algorithms.
更多查看译文
关键词
Fault detection,Fault approximation,Inverse scattering problem,MCDA
AI 理解论文
溯源树
样例
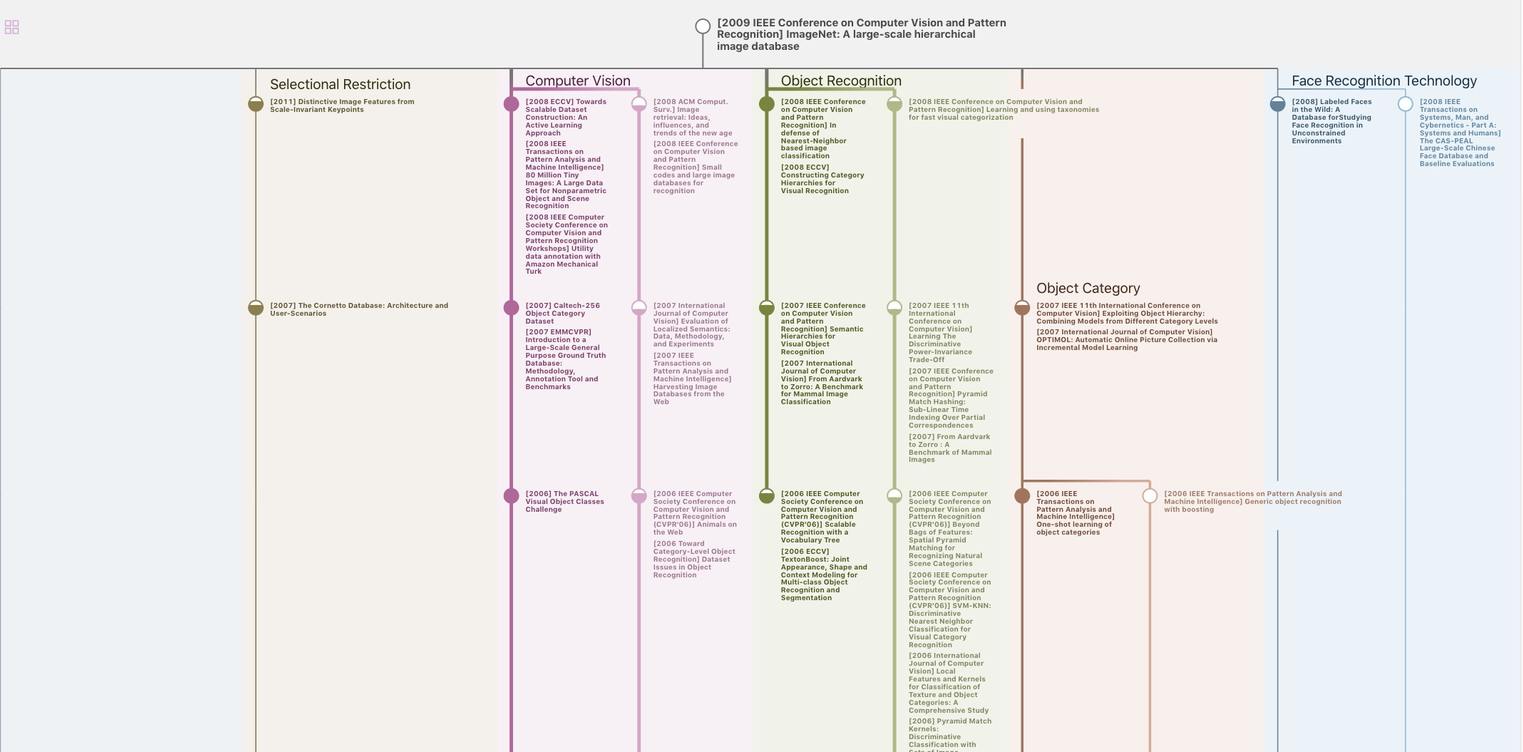
生成溯源树,研究论文发展脉络
Chat Paper
正在生成论文摘要