You shall know a species by the company it keeps: leveraging co-occurrence data to improve ecological prediction
bioRxiv (Cold Spring Harbor Laboratory)(2023)
摘要
Making predictions about species, including how they respond to environmental change, is a central challenge for ecologists. Due to the huge number of species, ecologists seek generalizations based on species' traits and phylogenetic relationships, but the predictive power of trait-based and phylogenetic models is often low. Species co-occurrence patterns may contain additional information about species' ecological attributes not captured by traits or phylogenies. We propose using ordination to encode the information contained in species co-occurrence data in low-dimensional vectors that can be used to represent species in ecological prediction. We present an efficient method to derive species vectors from co-occurrence data using GloVe, an unsupervised learning algorithm originally designed for language modeling. To demonstrate the method, we used GloVe to generate vectors for nearly 40,000 plant species using co-occurrence statistics derived from a global vegetation dataset and tested their ability to predict elevational range shifts in European montane plant species. Co-occurrence-based species vectors were weakly correlated with traits or phylogeny, indicating that they encode unique information about species. Models including co-occurrence-based vectors explained twice as much variation in species range shifts as models including only traits or phylogenetic information. Given the widespread availability of species occurrence data, species vectors learned from co-occurrence patterns are a widely applicable and powerful tool for encoding ecological information about species, with many potential applications for describing and predicting the ecology of species, communities, and ecosystems.
### Competing Interest Statement
The authors have declared no competing interest.
更多查看译文
关键词
ecological prediction,species,data,co-occurrence
AI 理解论文
溯源树
样例
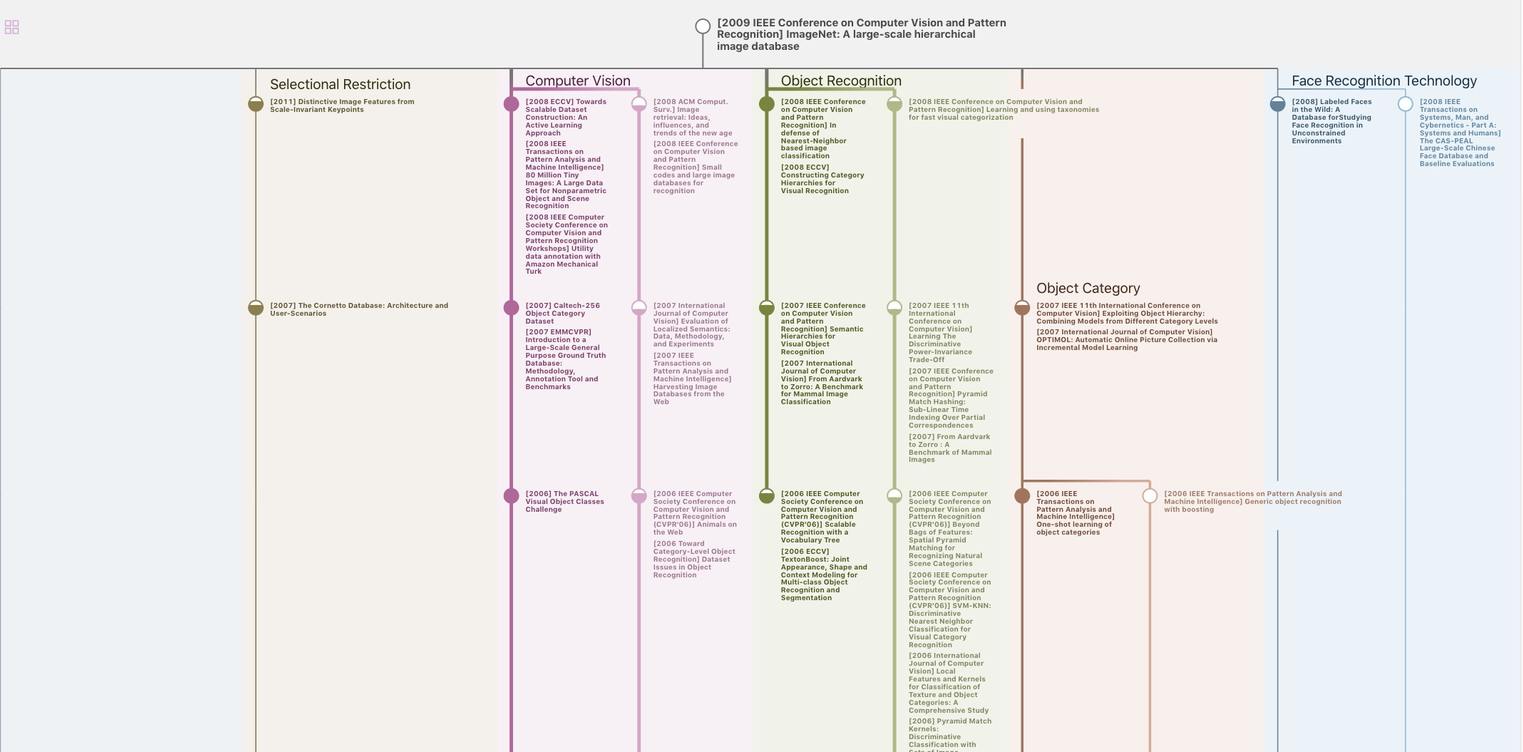
生成溯源树,研究论文发展脉络
Chat Paper
正在生成论文摘要