Multi-Stage Joint Level Motion Planning for Robot Assisted Peg-in-Hole Task
2022 International Conference on Industrial Automation, Robotics and Control Engineering (IARCE)(2022)
摘要
This paper proposes a joint level motion planning method for a six-DOF robot using deep reinforcement learning to execute the peg-in-hole task. We propose a multistage method based Soft Actor-Critic (SAC) network to learn the mapping from the task space to the joint space and make sequential control decisions. This scheme avoids laborious hand-crafted derived differential kinematics and inverse kinematics. To improve the performance, the training procedure is divided in two different stages. Each stage is trained with its focal point by designing a specific reward function. In the first stage, the robot carries the peg to a pre-insertion pose that locates vertically over the target, guided by a distance error based reward function. In the second stage, the robot inserts the peg into the hole with constant orientation and moving direction, and an orientation error based term is added into the reward function. These two stages are associated by a switching strategy considering the steps and the distance to target. In order make the two stages compatible with each other, the trained model of the first stage is directly used in the second stage training to execute pre-movement. After multi- stage training, our approach can successfully make sequential movement decisions in the joint level for peg-in-hole task.
更多查看译文
关键词
robotics,joint control,reinforcement learning,peg-in-hole,insertion
AI 理解论文
溯源树
样例
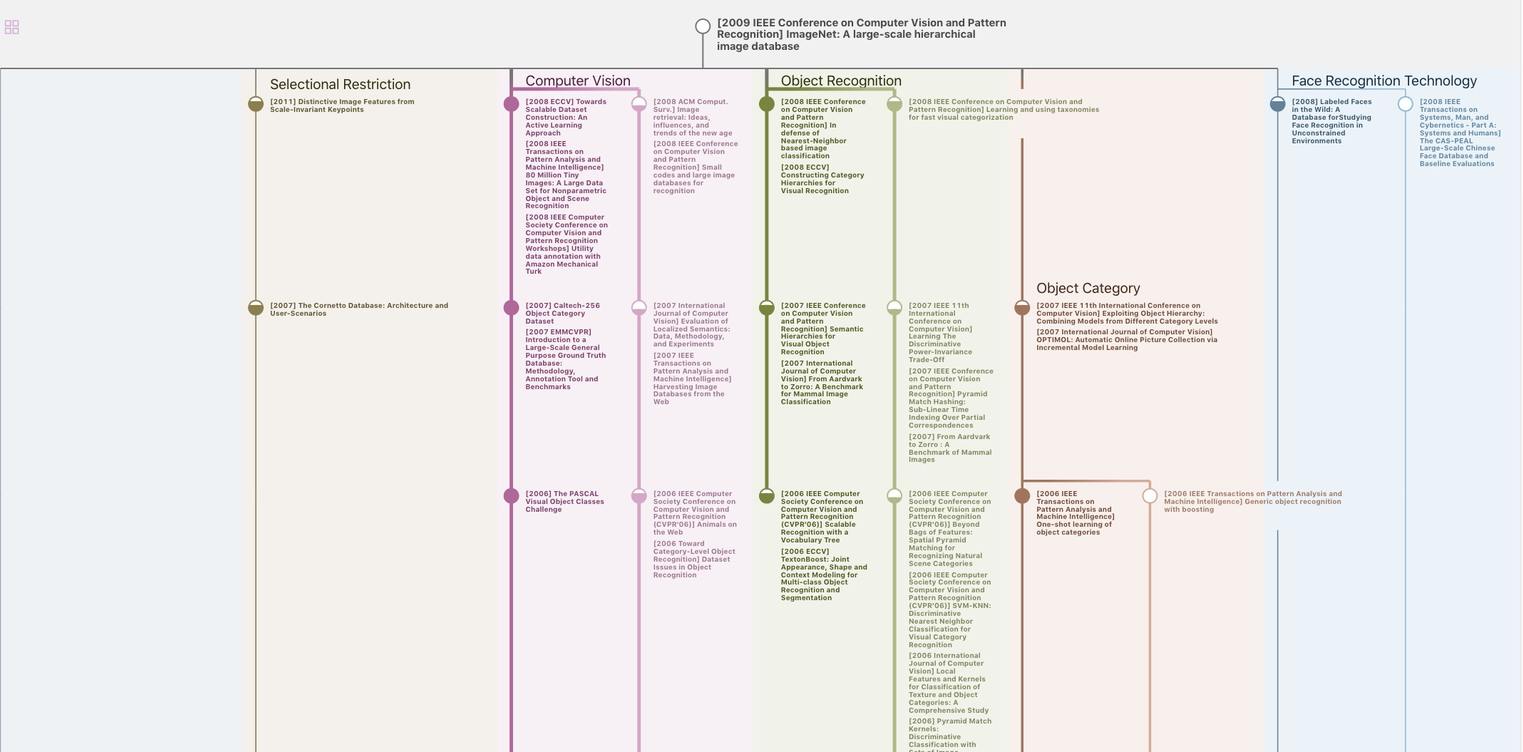
生成溯源树,研究论文发展脉络
Chat Paper
正在生成论文摘要