Advancements in the future of automating micromanipulation techniques in the IVF laboratory using deep convolutional neural networks
Journal of assisted reproduction and genetics(2022)
摘要
Purpose To determine if deep learning artificial intelligence algorithms can be used to accurately identify key morphologic landmarks on oocytes and cleavage stage embryo images for micromanipulation procedures such as intracytoplasmic sperm injection (ICSI) or assisted hatching (AH). Methods Two convolutional neural network (CNN) models were trained, validated, and tested over three replicates to identify key morphologic landmarks used to guide embryologists when performing micromanipulation procedures. The first model (CNN-ICSI) was trained ( n = 13,992), validated ( n = 1920), and tested ( n = 3900) to identify the optimal location for ICSI through polar body identification. The second model (CNN-AH) was trained ( n = 13,908), validated ( n = 1908), and tested ( n = 3888) to identify the optimal location for AH on the zona pellucida that maximizes distance from healthy blastomeres. Results The CNN-ICSI model accurately identified the polar body and corresponding optimal ICSI location with 98.9% accuracy (95% CI 98.5–99.2%) with a receiver operator characteristic (ROC) with micro and macro area under the curves (AUC) of 1. The CNN-AH model accurately identified the optimal AH location with 99.41% accuracy (95% CI 99.11–99.62%) with a ROC with micro and macro AUCs of 1. Conclusion Deep CNN models demonstrate powerful potential in accurately identifying key landmarks on oocytes and cleavage stage embryos for micromanipulation. These findings are novel, essential stepping stones in the automation of micromanipulation procedures.
更多查看译文
关键词
Artificial intelligence,Assisted hatching,Assisted reproductive technology,ICSI,Machine learning,Micromanipulation
AI 理解论文
溯源树
样例
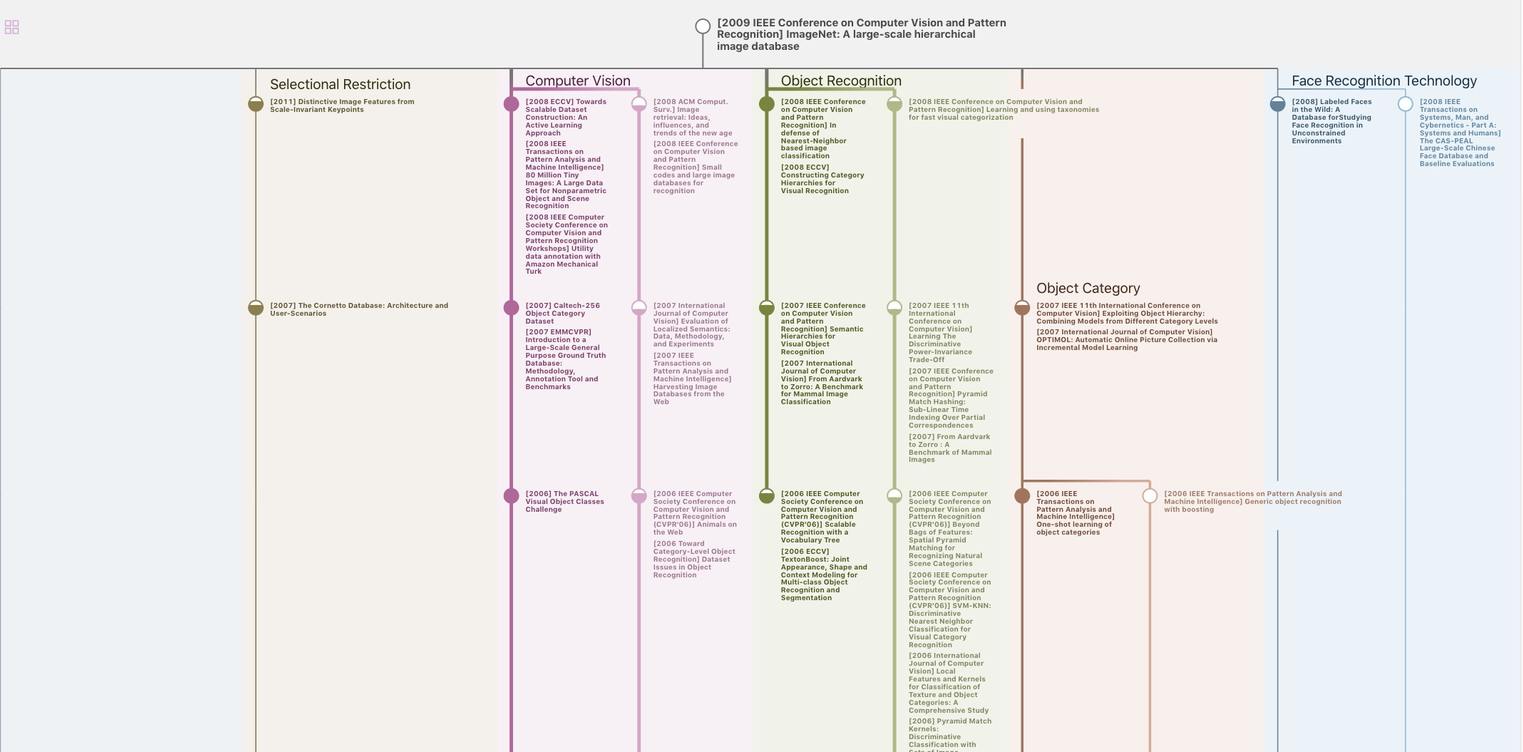
生成溯源树,研究论文发展脉络
Chat Paper
正在生成论文摘要