Stress-testing pelvic autosegmentation algorithms using anatomical edge cases.
Physics and imaging in radiation oncology(2023)
摘要
Commercial autosegmentation has entered clinical use, however real-world performance may suffer in certain cases. We aimed to assess the influence of anatomic variants on performance. We identified 112 prostate cancer patients with anatomic variations (edge cases). Pelvic anatomy was autosegmented using three commercial tools. To evaluate performance, Dice similarity coefficients, and mean surface and 95% Hausdorff distances were calculated versus clinician-delineated references. Deep learning autosegmentation outperformed atlas-based and model-based methods. However, edge case performance was lower versus the normal cohort (0.12 mean DSC reduction). Anatomic variation presents challenges to commercial autosegmentation.
更多查看译文
关键词
Anatomical variability,Autosegmentation,Deep learning,Edge case,Prostate cancer
AI 理解论文
溯源树
样例
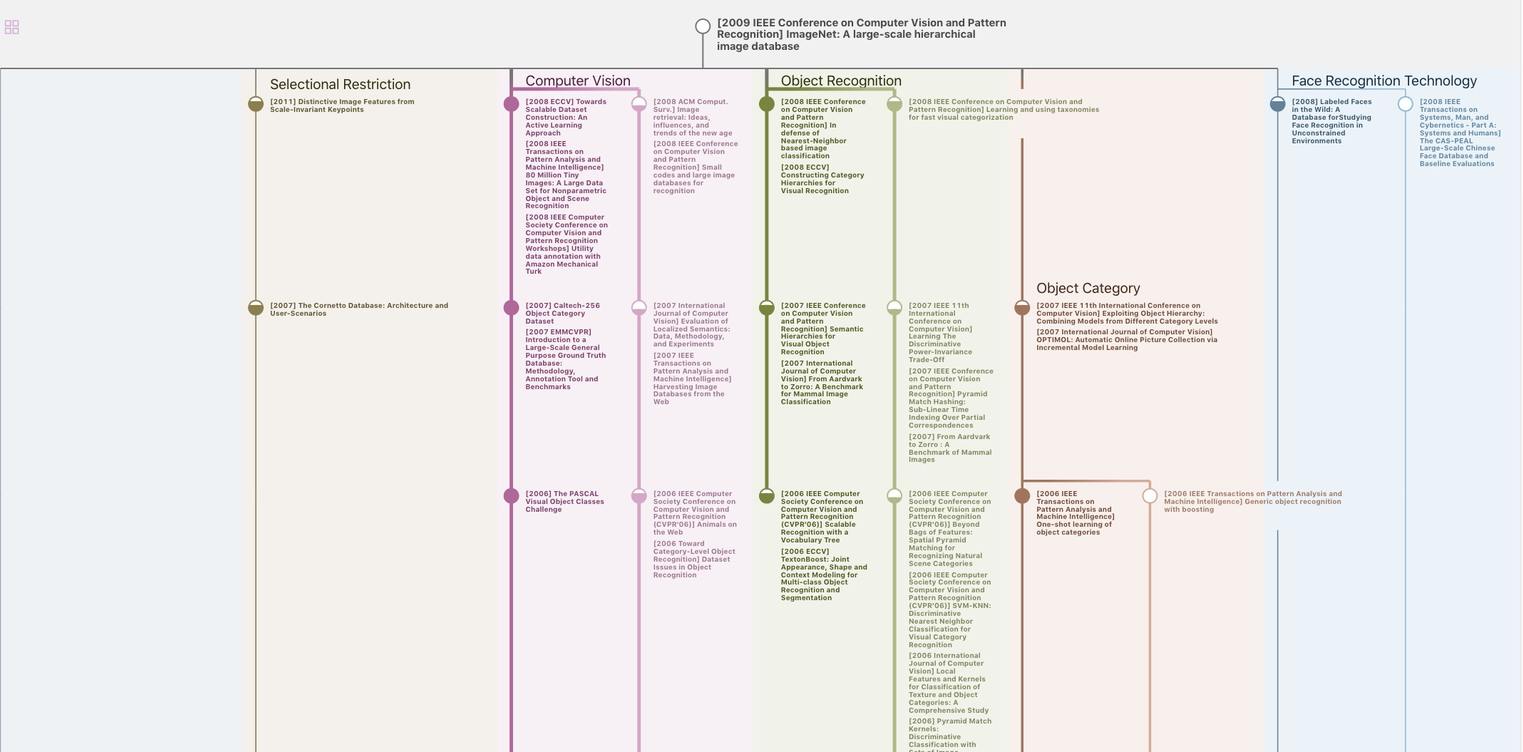
生成溯源树,研究论文发展脉络
Chat Paper
正在生成论文摘要