Improving risk prediction for target subpopulations: Predicting suicidal behaviors among multiple sclerosis patients.
PloS one(2023)
摘要
Several recent studies have applied machine learning techniques to develop risk algorithms that predict subsequent suicidal behavior based on electronic health record data. In this study we used a retrospective cohort study design to test whether developing more tailored predictive models-within specific subpopulations of patients-would improve predictive accuracy. A retrospective cohort of 15,117 patients diagnosed with multiple sclerosis (MS), a diagnosis associated with increased risk of suicidal behavior, was used. The cohort was randomly divided into equal sized training and validation sets. Overall, suicidal behavior was identified among 191 (1.3%) of the patients with MS. A Naïve Bayes Classifier model was trained on the training set to predict future suicidal behavior. With 90% specificity, the model detected 37% of subjects who later demonstrated suicidal behavior, on average 4.6 years before the first suicide attempt. The performance of a model trained only on MS patients was better at predicting suicide in MS patients than that a model trained on a general patient sample of a similar size (AUC of 0.77 vs. 0.66). Unique risk factors for suicidal behavior among patients with MS included pain-related codes, gastroenteritis and colitis, and history of smoking. Future studies are needed to further test the value of developing population-specific risk models.
更多查看译文
关键词
suicidal behaviors,multiple sclerosis,risk prediction,target subpopulations
AI 理解论文
溯源树
样例
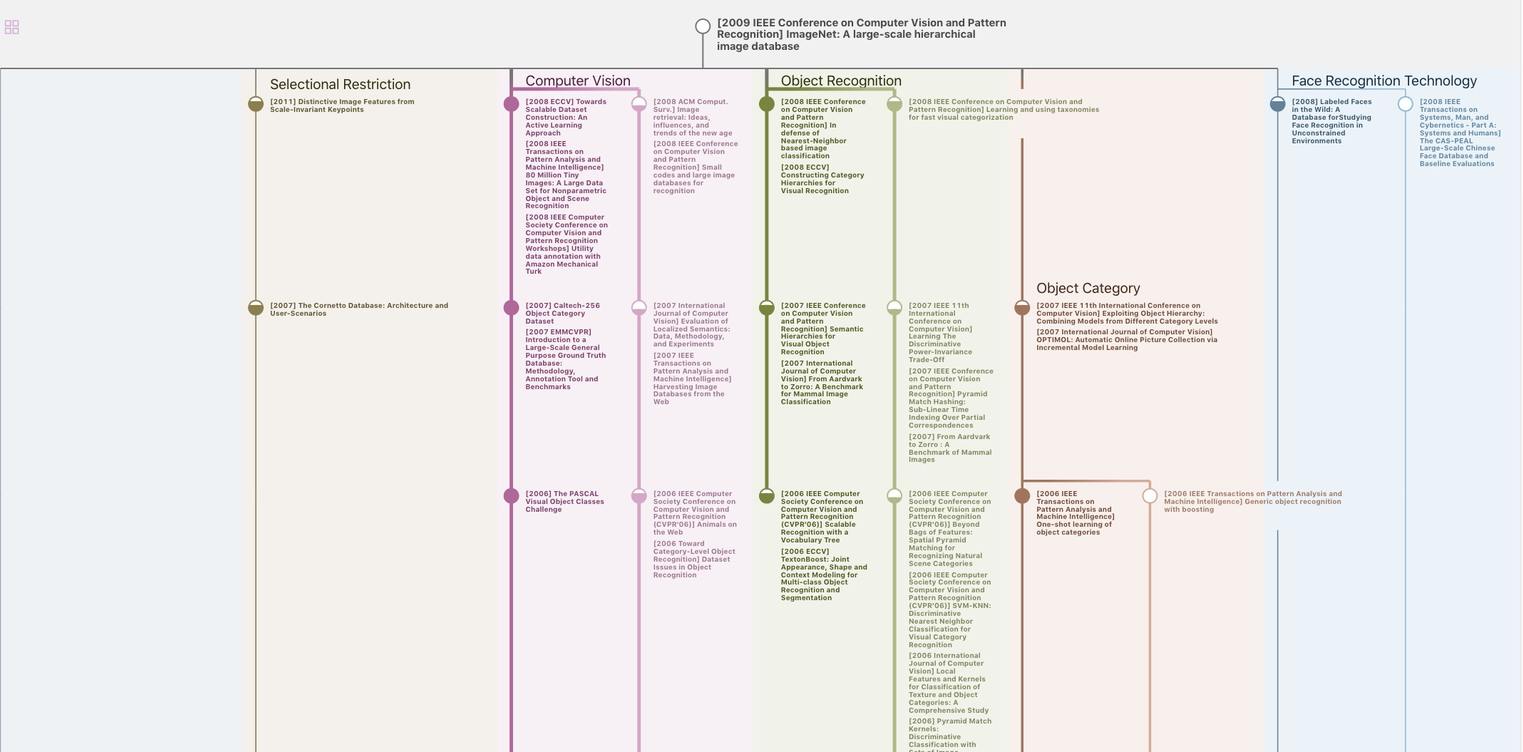
生成溯源树,研究论文发展脉络
Chat Paper
正在生成论文摘要