TopNEXt: Automatic DDA Exclusion Framework for Multi-Sample Mass Spectrometry Experiments
Bioinformatics(2023)
摘要
Motivation Liquid Chromatography Tandem Mass Spectrometry (LC-MS/MS) experiments aim to produce high quality fragmentation spectra which can be used to identify metabolites. However, current Data-Dependent Acquisition (DDA) approaches may fail to collect spectra of sufficient quality and quantity for experimental outcomes, and extend poorly across multiple samples by failing to share information across samples or by requiring manual expert input. Results We present TopNEXt, a real-time scan prioritisation framework that improves data acquisition in multi-sample LC-MS/MS metabolomics experiments. TopNEXt extends traditional DDA exclusion methods across multiple samples by using a Region of Interest (RoI) and intensity-based scoring system. Through both simulated and lab experiments we show that methods incorporating these novel concepts acquire fragmentation spectra for an additional 10% of our set of target peaks and with an additional 20% of acquisition intensity. By increasing the quality and quantity of fragmentation spectra, TopNEXt can help improve metabolite identification with a potential impact across a variety of experimental contexts. Availability TopNEXt is implemented as part of the ViMMS framework and the latest version can be found at https://github.com/glasgowcompbio/vimms. A stable version used to produce our results can be found at 10.5281/zenodo.7468914. Data can be found at 10.5525/gla.researchdata.1382. Contact r.mcbride.1@research.gla.ac.uk or vinny.davies@glasgow.ac.uk Supplementary information Supplementary data are available at Bioarxiv online.
更多查看译文
关键词
High-Performance Liquid Chromatography (HPLC),Liquid Chromatography
AI 理解论文
溯源树
样例
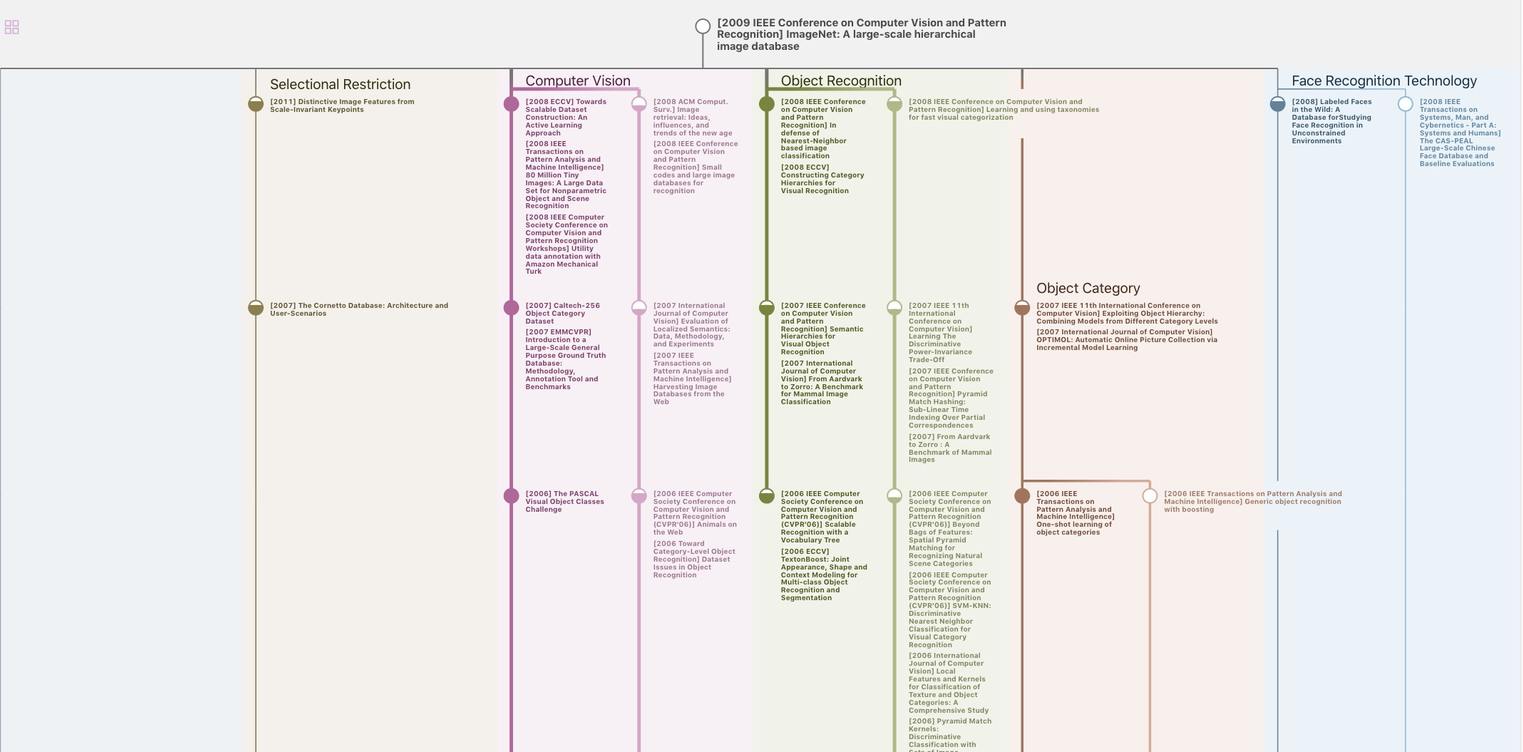
生成溯源树,研究论文发展脉络
Chat Paper
正在生成论文摘要