Top-K Confidence Map Aggregation for Robust Semantic Segmentation Against Unexpected Degradation.
2023 IEEE International Conference on Consumer Electronics (ICCE)(2023)
摘要
Convolutional Neural Networks (CNNs) have become the mainstream for computer vision tasks like semantic segmentation. The CNNs have substantially improved the performance of semantic segmentation. The implicit assumption for those CNN approaches is that the input image is clean without any image degradation. In an actual situation, however, the input image contains many unexpected image degradations such as noise, blur, or compression distortion. The performance of the conventional CNN approach is severely degraded by those unexpected image degradations. This paper presents a simple framework that makes the semantic segmentation algorithms robust against image degradation without re-training the original semantic segmentation CNN. Our key observation is that the down-sampled image is less sensitive to various types of degradation than the original-sized image. Therefore, we adopt a multi-scale test time augmentation (TTA). In the multi-scale TTA, the input image is firstly down-sampled with multiple scales. Then, inferred confidence maps are aggregated to obtain the final inference result. We also propose a top-K confidence map aggregation to further performance improvement. Experimental results demonstrate that our framework is more robust than existing methods for images with degradations and a real compressed video with H.264.
更多查看译文
关键词
semantic segmentation,image degradation,test time augmentation,confidence map aggregation
AI 理解论文
溯源树
样例
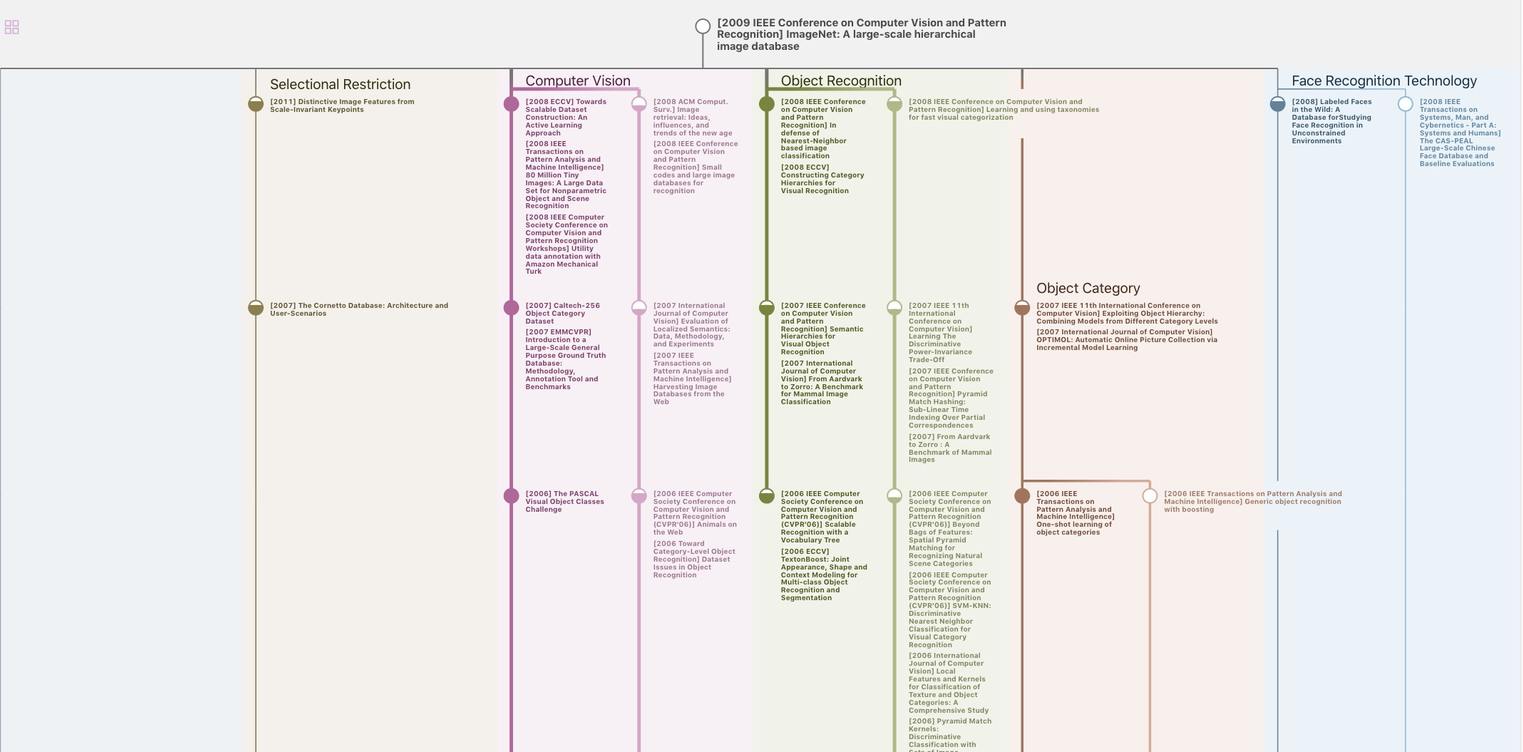
生成溯源树,研究论文发展脉络
Chat Paper
正在生成论文摘要