Enhancing Classification with Hierarchical Scalable Query on Fusion Transformer
2023 IEEE International Conference on Consumer Electronics (ICCE)(2023)
摘要
Real-world vision based applications require fine-grained classification for various applications of interest like e-commerce, mobile applications, warehouse management, etc. where reducing the severity of mistakes and improving the classification accuracy is of utmost importance. This paper proposes a method to boost fine-grained classification through a hierarchical approach via learnable independent query embeddings. This is achieved through a classification network that uses coarse class predictions to improve the fine class accuracy in a stage-wise sequential manner. We exploit the idea of hierarchy to learn query embeddings that are scalable across all levels, thus making this a relevant approach even for extreme classification where we have a large number of classes. The query is initialized with a weighted Eigen image calculated from training samples to best represent and capture the variance of the object. We introduce transformer blocks to fuse intermediate layers at which query attention happens to enhance the spatial representation of feature maps at different scales. This multi-scale fusion helps improve the accuracy of small-size objects. We propose a twofold approach for the unique representation of learnable queries. First, at each hierarchical level, we leverage cluster based loss that ensures maximum separation between inter-class query embeddings and helps learn a better (query) representation in higher dimensional spaces. Second, we fuse coarse level queries with finer level queries weighted by a learned scale factor. We additionally introduce a novel block called Cross Attention on Multi-level queries with Prior (CAMP) Block that helps reduce error propagation from coarse level to finer level, which is a common problem in all hierarchical classifiers. Our method is able to outperform the existing methods with an improvement of about 11% at the fine-grained classification.
更多查看译文
关键词
hierarchical scalable query,fusion,classification
AI 理解论文
溯源树
样例
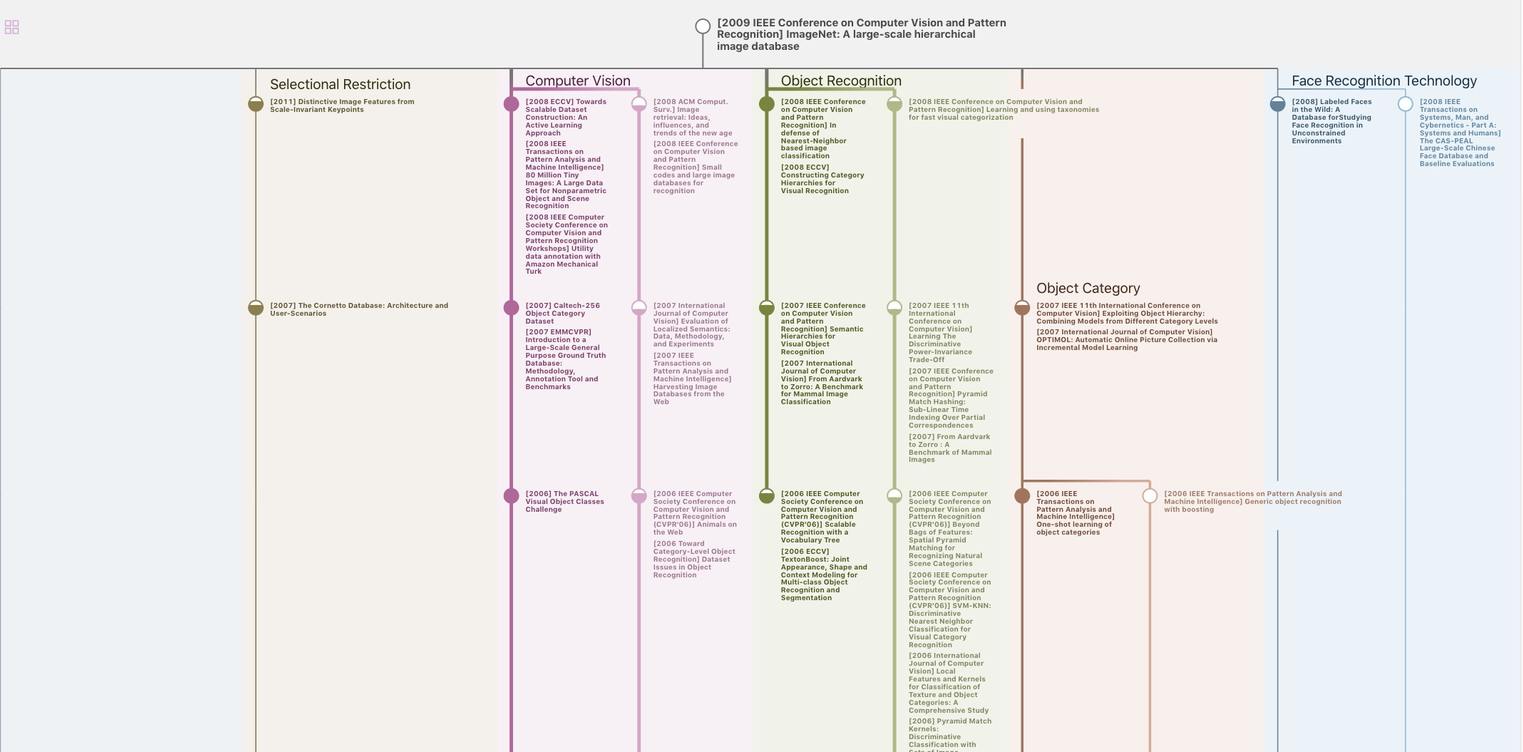
生成溯源树,研究论文发展脉络
Chat Paper
正在生成论文摘要