Seismic arrival-time picking on distributed acoustic sensing data using semi-supervised learning
Nature Communications(2023)
摘要
Distributed Acoustic Sensing (DAS) is an emerging technology for earthquake monitoring and subsurface imaging. However, its distinct characteristics, such as unknown ground coupling and high noise level, pose challenges to signal processing. Existing machine learning models optimized for conventional seismic data struggle with DAS data due to its ultra-dense spatial sampling and limited manual labels. We introduce a semi-supervised learning approach to address the phase-picking task of DAS data. We use the pre-trained PhaseNet model to generate noisy labels of P/S arrivals in DAS data and apply the Gaussian mixture model phase association (GaMMA) method to refine these noisy labels and build training datasets. We develop PhaseNet-DAS, a deep learning model designed to process 2D spatio-temporal DAS data to achieve accurate phase picking and efficient earthquake detection. Our study demonstrates a method to develop deep learning models for DAS data, unlocking the potential of integrating DAS in enhancing earthquake monitoring.
更多查看译文
关键词
acoustic sensing data,arrival-time,semi-supervised
AI 理解论文
溯源树
样例
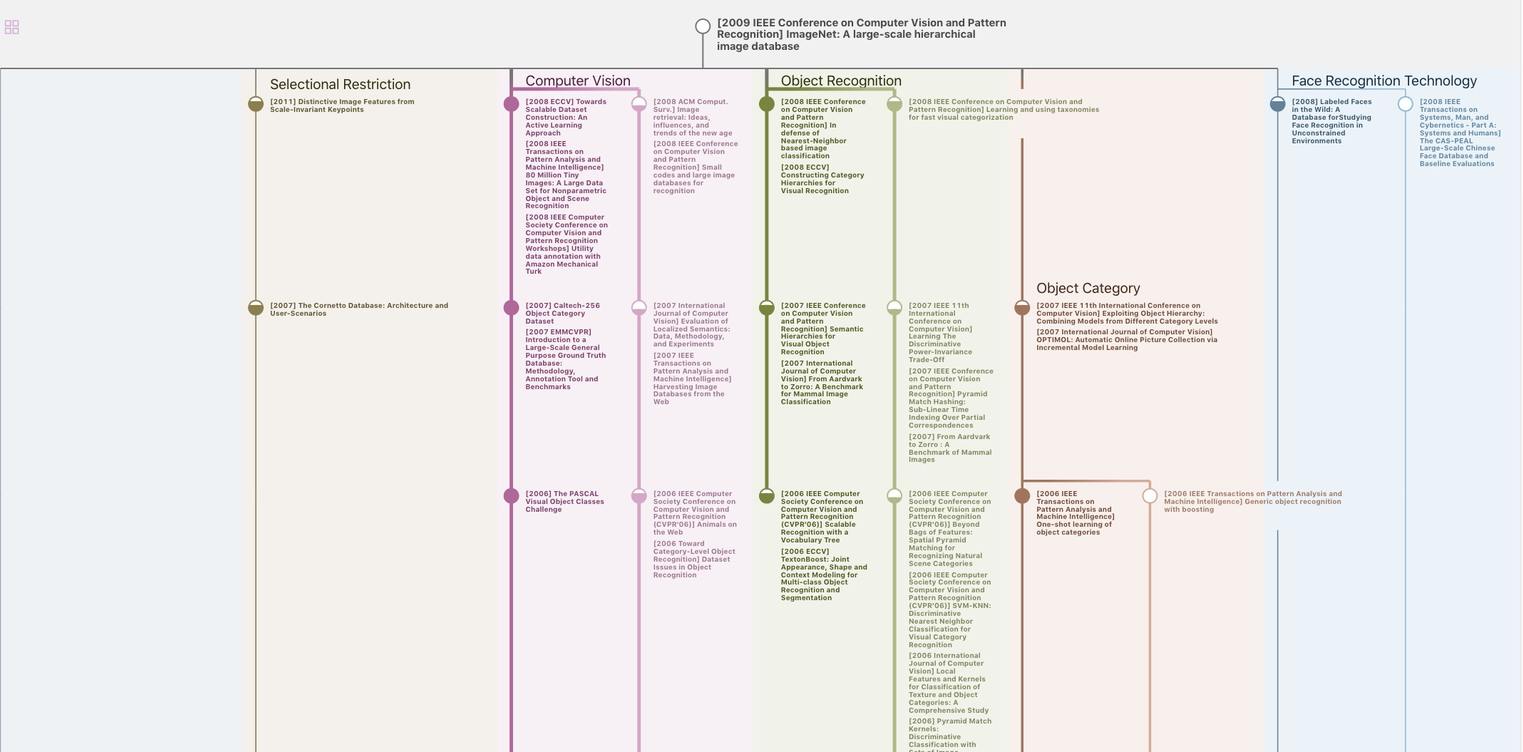
生成溯源树,研究论文发展脉络
Chat Paper
正在生成论文摘要