Vision, Deduction and Alignment: An Empirical Study on Multi-modal Knowledge Graph Alignment
arxiv(2023)
摘要
Entity alignment (EA) for knowledge graphs (KGs) plays a critical role in knowledge engineering. Existing EA methods mostly focus on utilizing the graph structures and entity attributes (including literals), but ignore images that are common in modern multi-modal KGs. In this study we first constructed Multi-OpenEA -- eight large-scale, image-equipped EA benchmarks, and then evaluated some existing embedding-based methods for utilizing images. In view of the complementary nature of visual modal information and logical deduction, we further developed a new multi-modal EA method named LODEME using logical deduction and multi-modal KG embedding, with state-of-the-art performance achieved on Multi-OpenEA and other existing multi-modal EA benchmarks.
更多查看译文
关键词
entity alignment,entity attributes,graph structures,image-equipped EA benchmark,knowledge engineering,LODEME,logical deduction,multimodal EA method,multimodal KG embedding,multimodal knowledge graph alignment,multiOpenEA,visual modal information
AI 理解论文
溯源树
样例
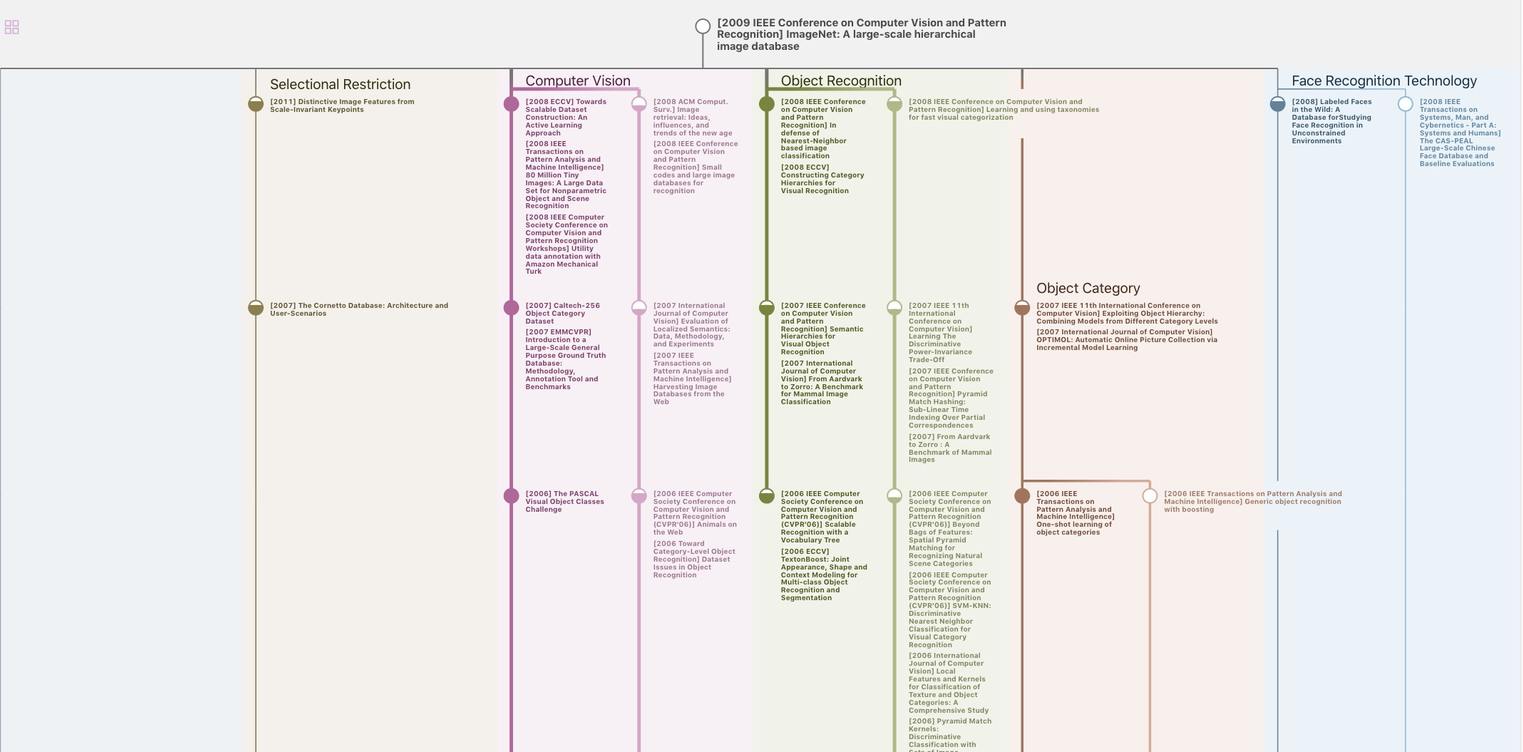
生成溯源树,研究论文发展脉络
Chat Paper
正在生成论文摘要