Few-shot 3D LiDAR Semantic Segmentation for Autonomous Driving
arxiv(2023)
摘要
In autonomous driving, the novel objects and lack of annotations challenge the traditional 3D LiDAR semantic segmentation based on deep learning. Few-shot learning is a feasible way to solve these issues. However, currently few-shot semantic segmentation methods focus on camera data, and most of them only predict the novel classes without considering the base classes. This setting cannot be directly applied to autonomous driving due to safety concerns. Thus, we propose a few-shot 3D LiDAR semantic segmentation method that predicts both novel classes and base classes simultaneously. Our method tries to solve the background ambiguity problem in generalized few-shot semantic segmentation. We first review the original cross-entropy and knowledge distillation losses, then propose a new loss function that incorporates the background information to achieve 3D LiDAR few-shot semantic segmentation. Extensive experiments on SemanticKITTI demonstrate the effectiveness of our method.
更多查看译文
关键词
autonomous driving,base classes,deep learning,few-shot 3D LiDAR semantic segmentation method,few-shot learning,few-shot semantic segmentation methods,traditional 3D LiDAR semantic segmentation
AI 理解论文
溯源树
样例
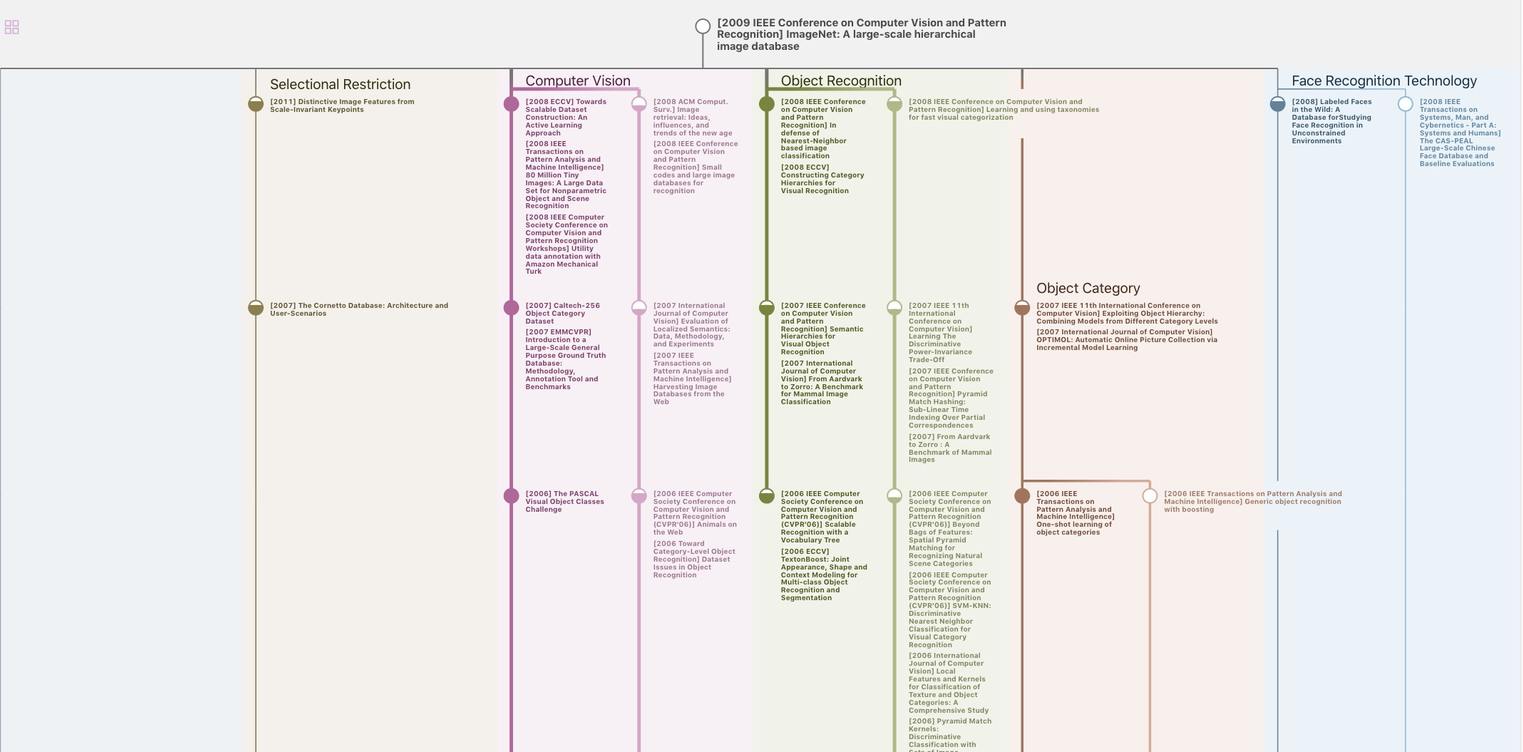
生成溯源树,研究论文发展脉络
Chat Paper
正在生成论文摘要