Towards Resource-aware DNN Partitioning for Edge Devices with Heterogeneous Resources.
GLOBECOM(2022)
摘要
Collaborative deep neural network (DNN) inference over edge and cloud is emerging as an effective approach for enabling several Internet of Things (IoT) applications. Edge devices are mainly resource-constrained and hence can not afford the computational complexity manifested by DNNs. Thereby, researchers have resorted to a collaborative computing approach, where a DNN is partitioned between edge and cloud. Recent art on DNN partitioning has either focused on bandwidth-specific partitioning or relied on offline benchmarking of DNN layers. However, edge devices are inherently heterogeneous and possess inconsistent levels and types of resources. Therefore, in this work, we propose a resource-aware partitioning of DNNs for accelerating collaborative inference over edge-cloud. The proposed approach provides the flexibility of partitioning a DNN with respect to the available nature and scale of resources for a certain edge device. Unlike state-of-the-art, we exploit different types of DNN complexities for partitioning them on heterogeneous edge devices. For example, in a bandwidth-constrained scenario, our approach gained 40% efficiency as compared to the offline benchmarking approach. Therefore, given the different nature of edge devices' computational, storage, and energy requirements, this approach provides a suitable configuration for edge-cloud synergetic inference.
更多查看译文
关键词
Edge intelligence,Collaborative DNN inference,Resource efficiency,Internet of things
AI 理解论文
溯源树
样例
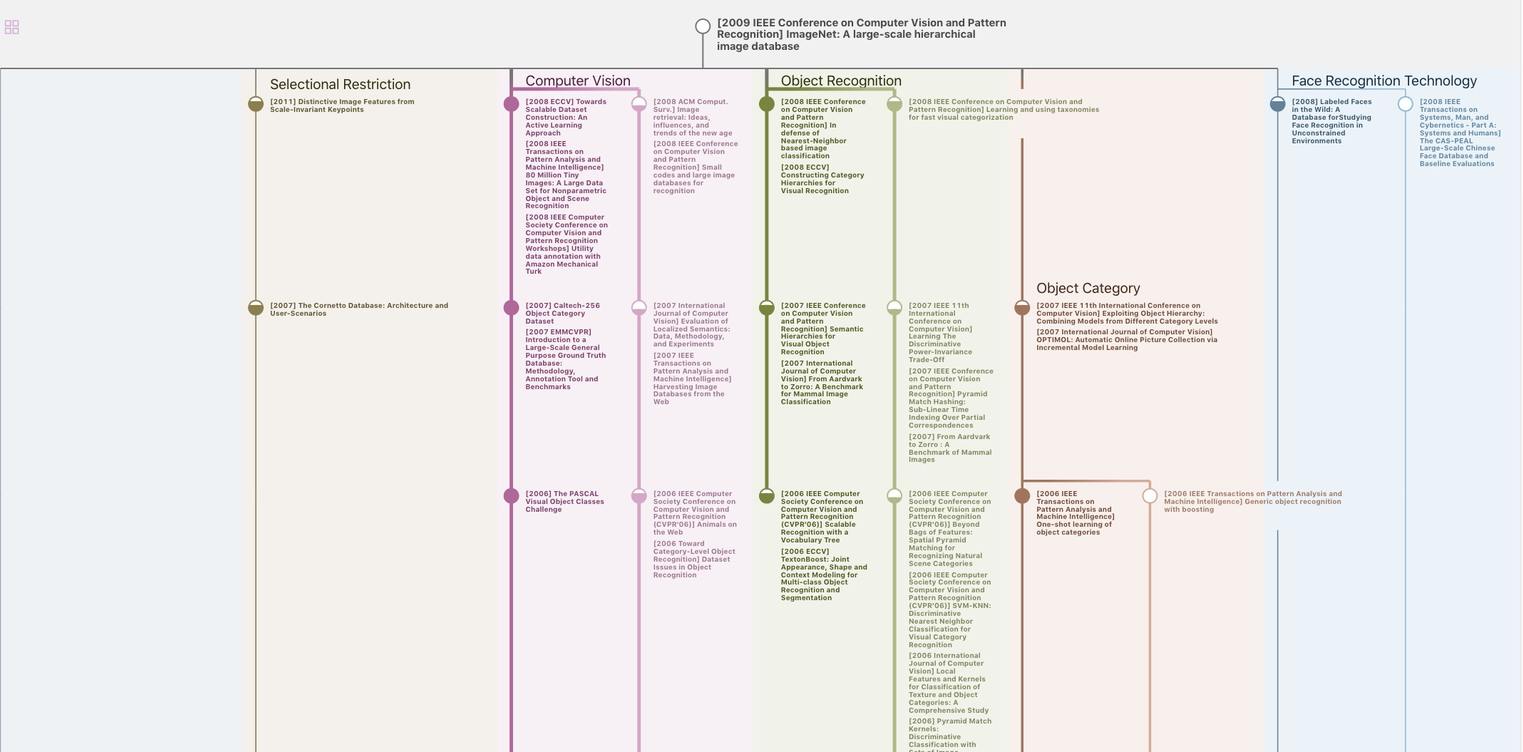
生成溯源树,研究论文发展脉络
Chat Paper
正在生成论文摘要