Radio Frequency Identification for Drones Using Spectrogram and CNN.
GLOBECOM(2022)
摘要
Over the past few years, commercial drones have grown in popularity. However, the pervasive use of drones may pose a range of secure risks to sensitive areas such as airports and military bases. Hence, drone detection and identification are critical and necessary for governments and security agencies. This paper proposes a radio frequency identification (RFI) system for drones based on spectrogram and convolutional neural network (CNN). Specifically, spectrogram is used to represent fine-grained time-frequency characteristics of drone signals. Then CNN is designed to infer drone types by identifying their spectrograms. In practice, drones have different operating channels, and any one of them can be selected for signal transmission. It means that the carrier frequencies of their signals are unknown, which may result in misclassifications. To address this problem, we collect drone signals from all potential frequency bands, and demonstrate that carrier frequency offset (CFO) compensation can significantly improve the system performance. Experimental evaluation is performed in real wireless environments involving 6 drones and a Universal Software Radio Peripheral (USRP) X310 platform. Moreover, the proposed spectrogram-based CNN can reach the best performance compared with the IQ-based and FFT-based CNNs. The classification accuracy is beyond 98% for drones operating on arbitrary channels.
更多查看译文
关键词
Drone classification, short-time Fourier transform, CFO compensation, CNN
AI 理解论文
溯源树
样例
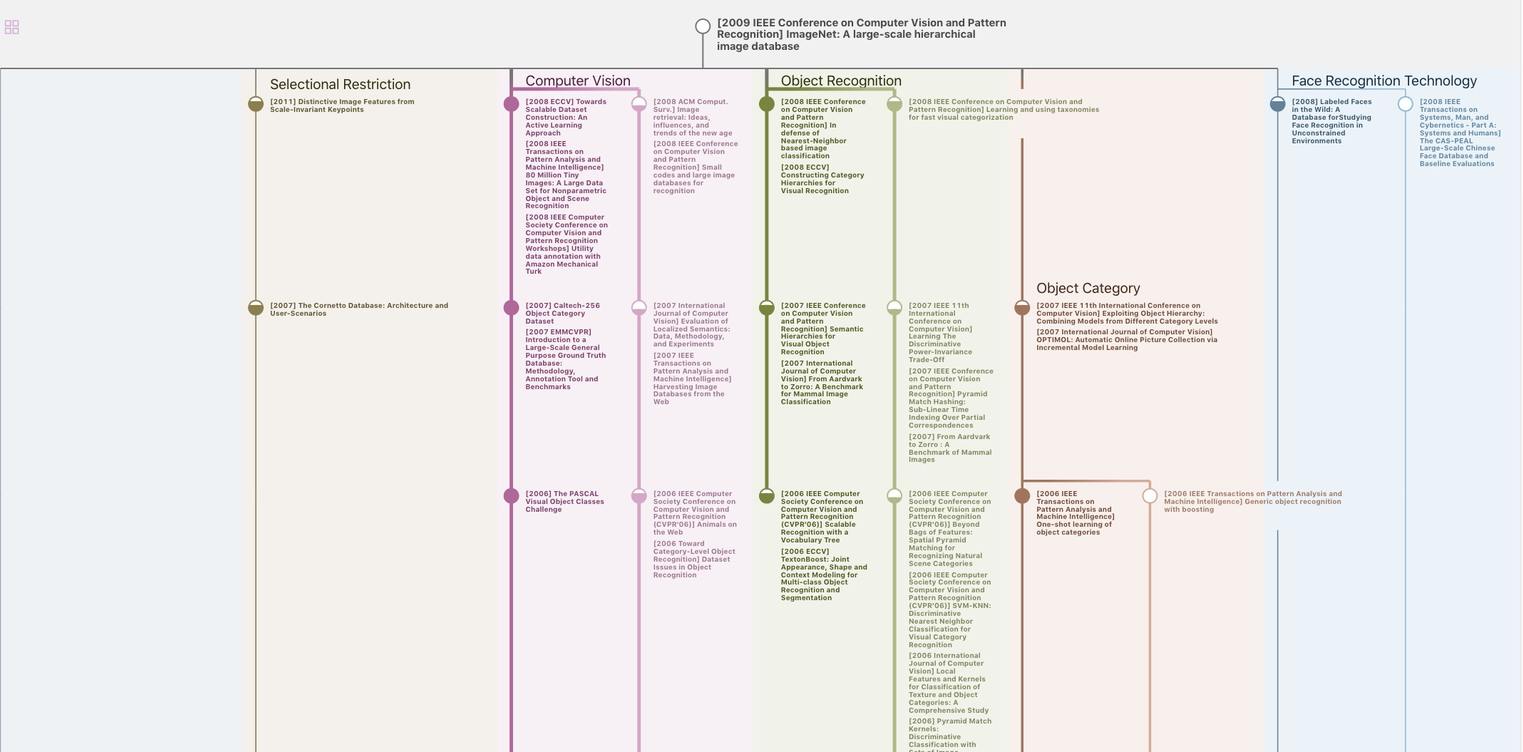
生成溯源树,研究论文发展脉络
Chat Paper
正在生成论文摘要