Power-Aware Traffic Engineering for Data Center Networks via Deep Reinforcement Learning.
GLOBECOM(2022)
摘要
The issue of high energy consumption and low energy utilization in data center networks (DCNs) has always been the focus of attention of both academia and industry. One general solution is to select a subset of network devices that can meet the traffic transmission requirements, thereby turning off the remaining redundant devices. However, modeling the problem as integer linear programming introduces significant time overhead, while heuristic approaches often suffer from poor generalizability. In this paper, we propose GreenDCN.ai, a closed-loop control system, which utilizes In-band Network Telemetry to collect the network-wide device-internal state, and leverages a Deep Reinforcement Learning-based energy-saving algorithm to make rapid decisions to turn on or off network device ports in response to the real-time network state. The trained GreenDCN.ai can adaptively adjust its energy-saving strategy without human intervention when the DCN topology changes. Besides, based on the regularity of the DCN topology, we design two training complexity reduction methods to address the non-convergence issue under large-scale DCN topologies. Specifically, we split the large-scale DCN topology into sub-topologies for parallel training on each sub-topology without breaking the DCN topology connectivity. Evaluation on software P4 switches suggests that GreenDCN.ai can achieve stable convergence within 590 episodes, generate effective action decisions within 79 mu s, and save about 34% to 39% of the network energy consumption.
更多查看译文
关键词
closed-loop control system,complexity reduction methods,data center networks,DCN topology,deep reinforcement learning,deep reinforcement learning-based energy-saving algorithm,energy consumption,energy utilization,greenDCN,in-band network telemetry,integer linear programming,network energy consumption,network-wide device-internal state,power-aware traffic engineering,software P4 switches,traffic transmission
AI 理解论文
溯源树
样例
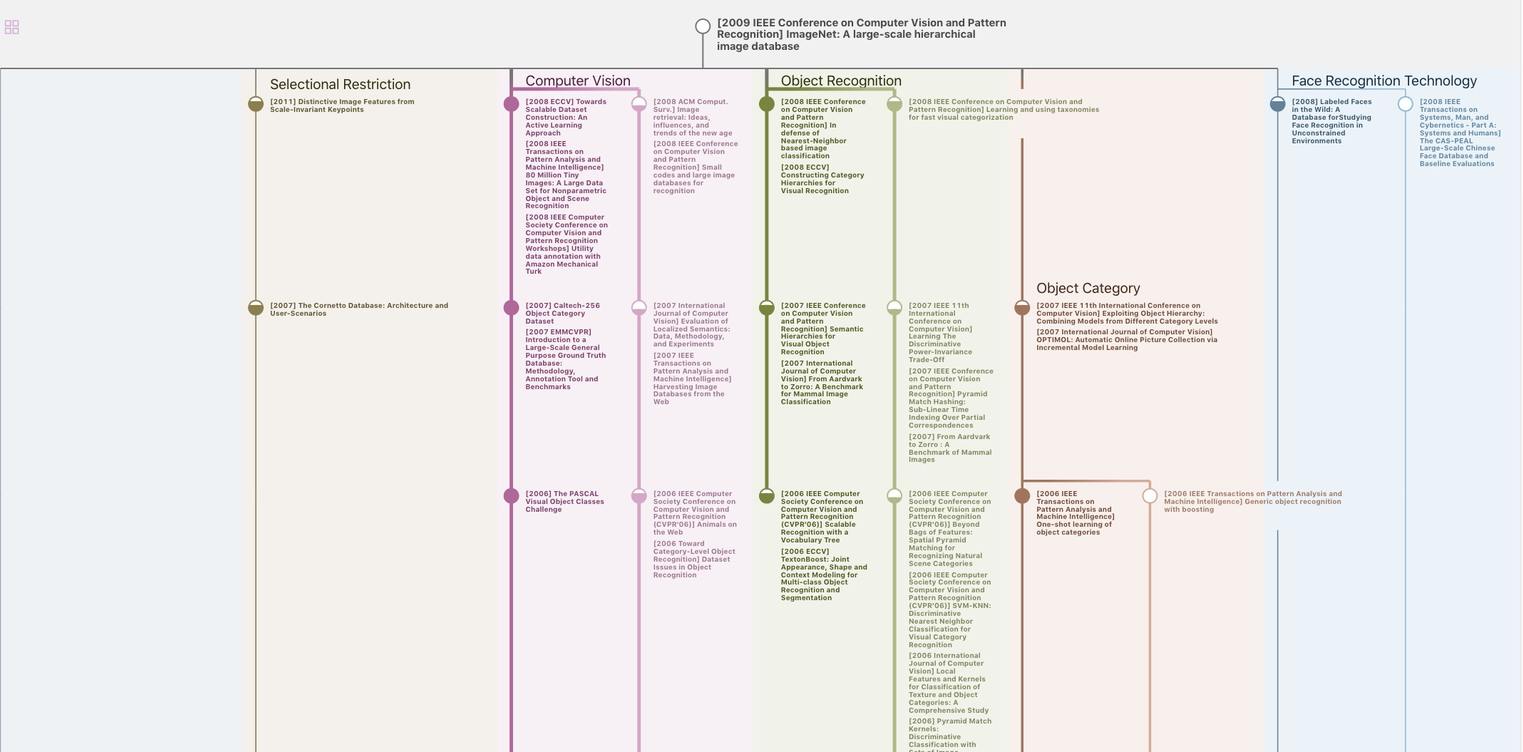
生成溯源树,研究论文发展脉络
Chat Paper
正在生成论文摘要