FCM-GCN-based upstream and downstream dependence model for air traffic flow networks
Knowledge-Based Systems(2023)
摘要
The upstream and downstream dependence of air traffic flow networks (ATFN) is a key step in identifying the changing characteristics and interaction patterns of air traffic flow. This requires mining the flow state and operation information of en route flights from multiple angles. However there have been challenges in terms of methodology and practical application. In this study, we propose a hybrid model which combines fuzzy c-means (FCM) and graph convolution network (GCN) for air traffic dependence. Practice has proven that the ATFN graph structure generated by clustering trajectory data using FCM can clearly reflect crossing points and mainstream paths. The GCN is used to detect the trajectory deviation, time delay, and tolerance of the ATFN at different times or paths and visualise the output characteristic layer, which can effectively identify the temporal and spatial correlation of the flow state. Experimental results on real data show that FCM-GCN is significantly better than other baseline models in medium-and long-term traffic prediction tasks. It achieved its best performance in the 60-min traffic prediction compared with existing methods. The experimental results in the selected case area propose a general research framework on the upstream and downstream dependence of ATFN and reflect the efficiency of the organisation and differentiation of ATFN. Its integration with air traffic flow management is conducive to the optimisation of highly interconnected and interdependent flow networks. (c) 2022 Elsevier B.V. All rights reserved.
更多查看译文
关键词
Graph convolution network,Fuzzy c -means,Dependence,Air traffic prediction
AI 理解论文
溯源树
样例
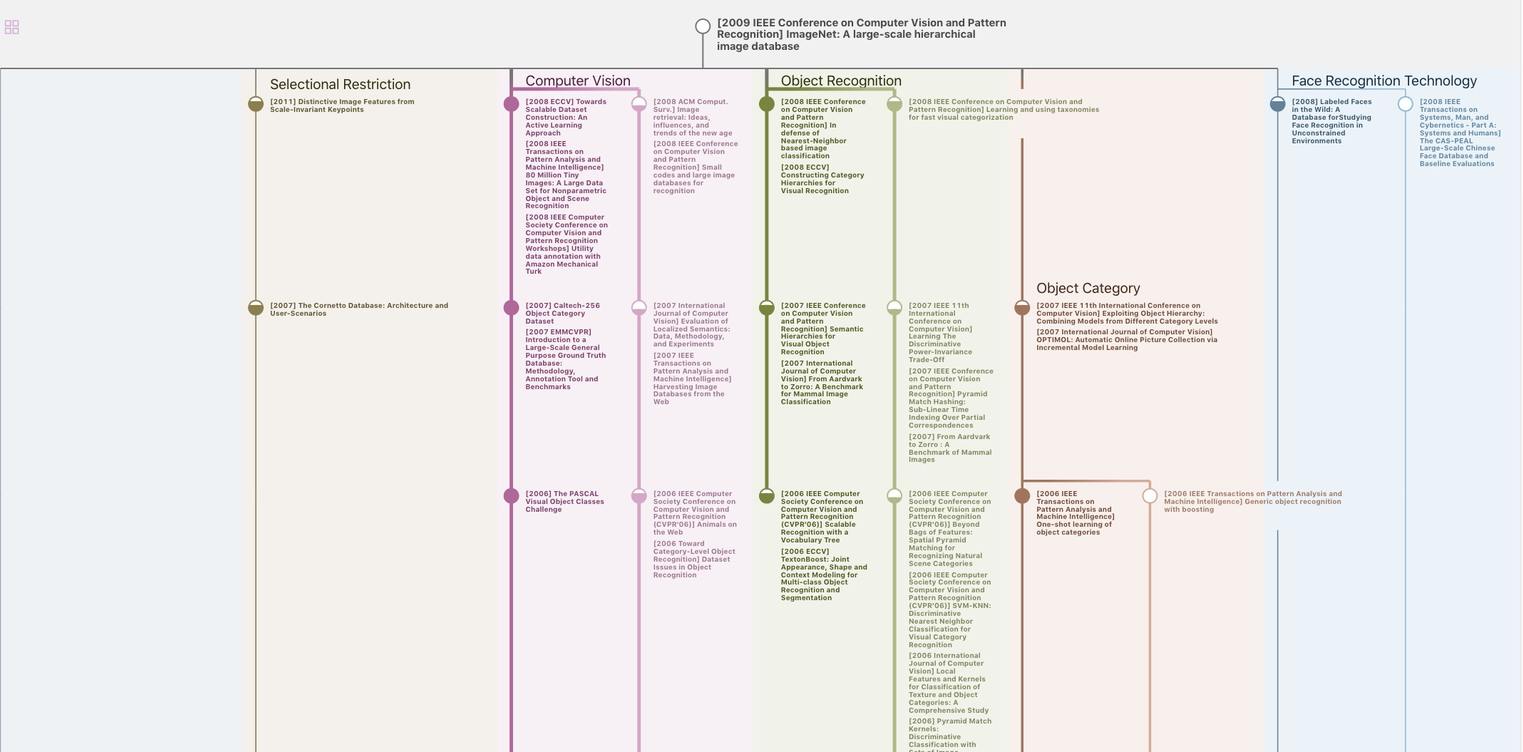
生成溯源树,研究论文发展脉络
Chat Paper
正在生成论文摘要