Two-phase self-supervised pretraining for object re-identification
Knowledge-Based Systems(2023)
摘要
Self-supervised pretraining (e.g., MAE) based on reconstructing masked image patches has become increasingly popular in recent months. It commonly pretrains models on the large-scale ImageNet dataset with a considerably wider range of image categories, and then finetunes on downstream tasks such as classification, detection, segmentation, etc. However, we discover that for finetuning domain-specific tasks usually with a few or limited semantic spaces (e.g., object re-identification (ReID)), the model pretrained on a wider variety of sources (e.g., ImageNet) fails to be a good initialization. To address the problem, we propose a second-phase self-supervised pretraining on the specific domain, based on the self-supervised ImageNet pretraining models in the first phase. We perform studies on 2 domains of object ReID, i.e., person and vehicle ReID, where person ReID domain involves four datasets (Market-1501, DukeMTMC, MSMT17, Occluded-Duke) and vehicle ReID domain contains 2 datasets (VeRi-776, VehicleID). Through extensive experiments, we observe significant improvements in every single dataset based on the second-phase self-supervised pretraining over the entire unlabeled domain data. Specifically in person ReID task, compared to the self-supervised model in the first phase, our approach achieves +8.5%/+11.5%/+13.3%/+17.2% mAP improvements in Market-1501, DukeMTMC, MSMT17 and Occluded-Duke datasets, respectively. Furthermore, it also outperforms the supervised pretraining baselines, achieving improved performance by +1.5%/+4.3%/+3.9%/+4.2% mAP. We observe similar inspiring improvements in vehicle ReID tasks. Additionally, we also perform the second-phase pretraining on the specific tasks using its own unlabeled data, namely task-specific pretraining (TSP), which also consistently shows encouraging results across object ReID tasks.
更多查看译文
关键词
Self-supervised pretraining,MAE,Domain adaptation,Person re-identification,Vehicle re-identification
AI 理解论文
溯源树
样例
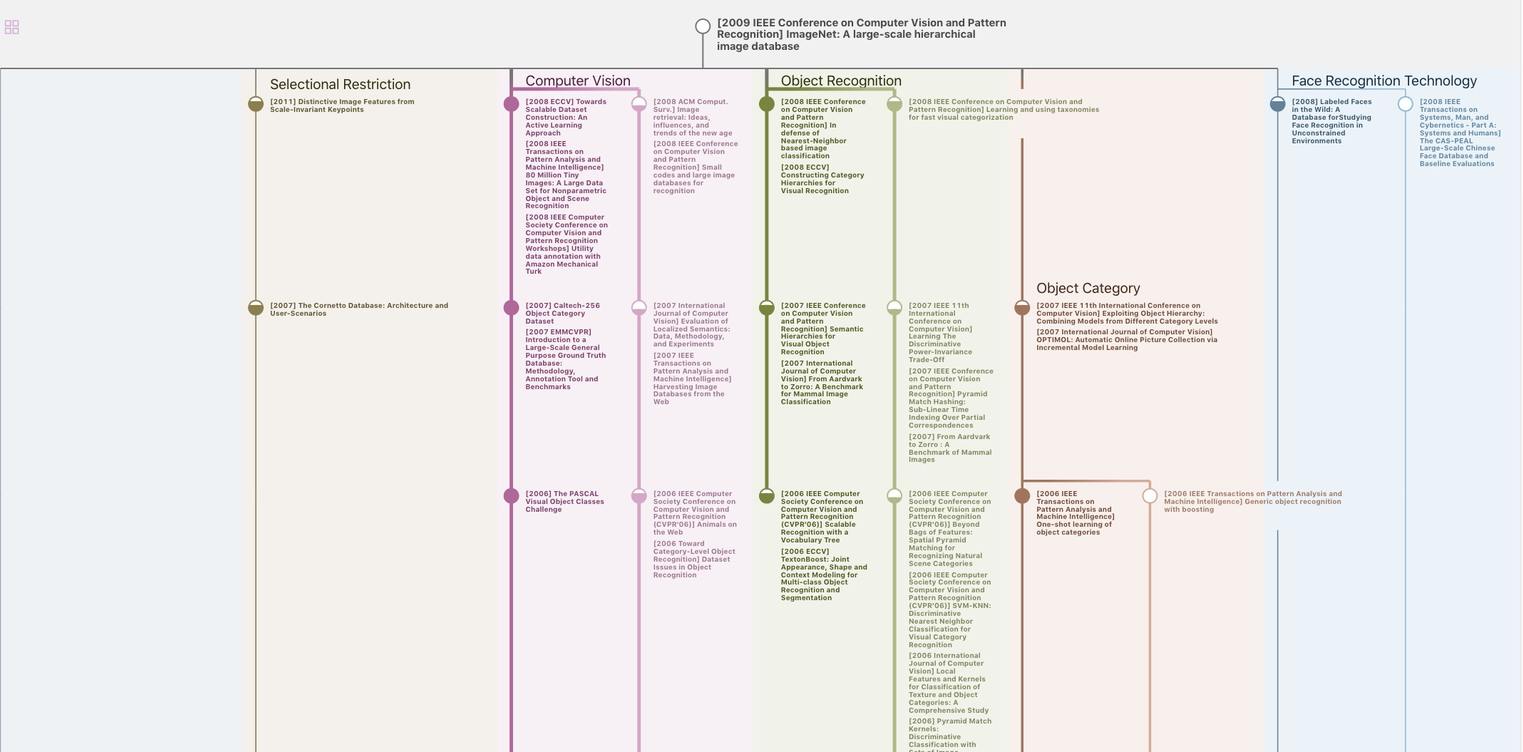
生成溯源树,研究论文发展脉络
Chat Paper
正在生成论文摘要