Multi-Aspect co-Attentional Collaborative Filtering for extreme multi-label text classification
Knowledge-Based Systems(2023)
摘要
The extreme multi-label text classification (XMTC) keeps attracting researchers’ attention due to its wide application. Recent studies have been trying to enhance text representation or reduce the number of labels to optimize the lack of information in a text or the sparsity of the possibility vector. In the social recommendation field, a similar problem has already been defined and studied extensively, the methods from which can be adopted in XMTC to identify matching relations in large datasets accurately. Thus, we proposed a general architecture enhanced by a pre-defined global nearest label neighbor group for XMTC, which reformulates the learning task to an interaction function between document and label by a multi-layer perceptron. Further, with co-attention mechanism and neural collaborative filtering, our architecture learns informative label representation enhanced by document-specific label group vector and label-specific text feature vector, which builds an effective interaction function to get a matching score. Through extensive experiments comparing various models and ablation studies, results show that our proposed architecture outperforms most existing methods for XMTC and significantly improves the performance of elemental document encoders.
更多查看译文
关键词
Extreme multi-label text classification,Multi-aspect representation,Co-attention mechanism,Neural collaborative filtering
AI 理解论文
溯源树
样例
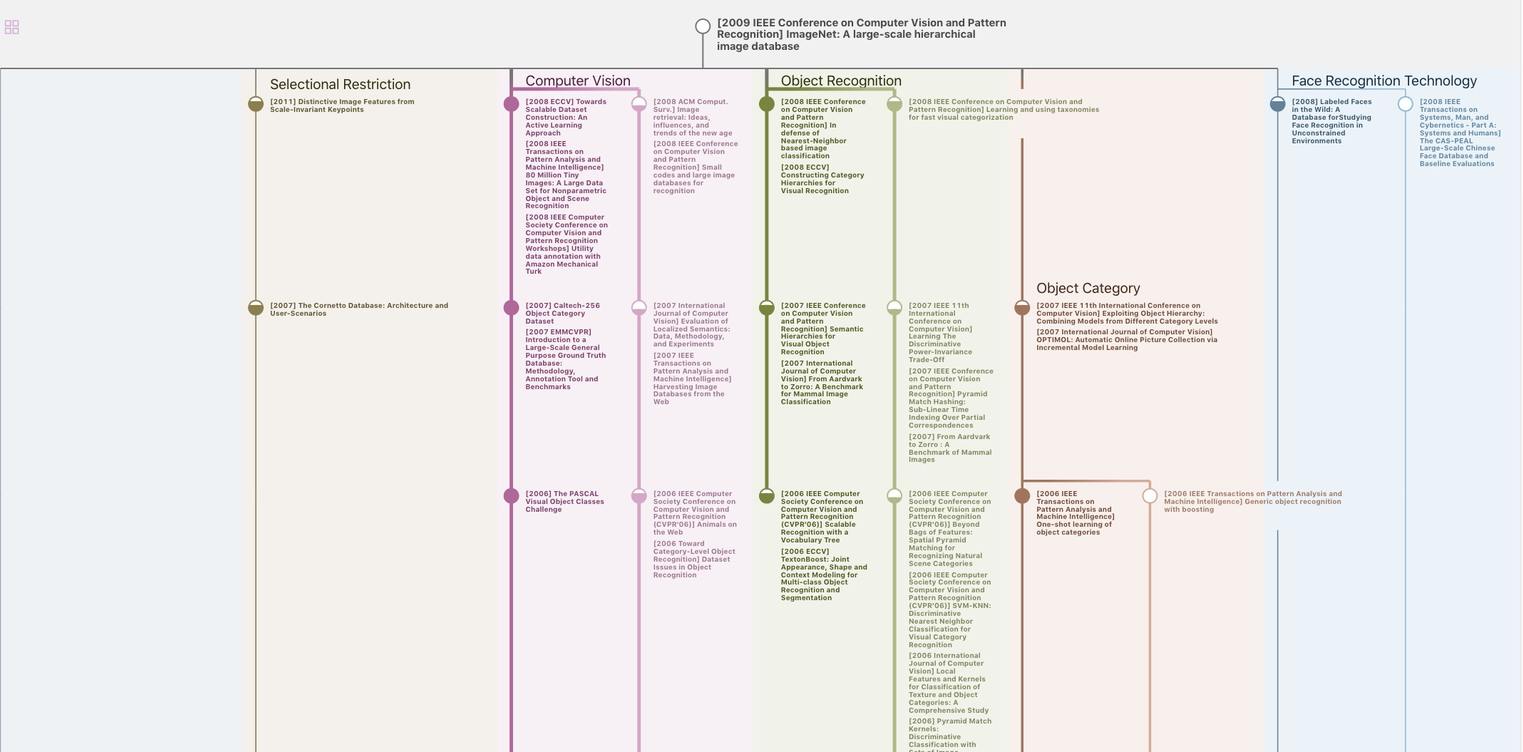
生成溯源树,研究论文发展脉络
Chat Paper
正在生成论文摘要