Modeling and predicting user preferences with multiple item attributes for sequential recommendations
Knowledge-Based Systems(2023)
摘要
Sequential recommendations have become a focus of attention across the deep learning community owing to their fitness to the actual application scenario. Although recently we have witnessed a surge of work on sequential recommender systems, they are still insufficient in exploring and exploiting item-attribute relations to enhance prediction accuracy. In this work, we propose a novel technological framework, MIA-SR, for sequential recommendation (SR) by modeling and predicting user preferences with multiple item attributes (MIA). When modeling the dynamic behavior of a user, not only the item sequence but also the attribute sequence is used to generate the fused representation of users. Further, we propose using a graph convolution network on the item-attribute bipartite graph to enhance the representations of items and attribute entities. Moreover, MIA-SR is naturally empowered with a multi-tasking strategy to exploit inductive bias among different preference signals and enhance item recommendation. Extensive experiments on public benchmark datasets have verified the merits of MIA-SR. The source code and data are available at: https://github.com/619496775/MIA-SR.
更多查看译文
关键词
Sequential recommendation,User preferences,Graph convolution network,Multi-task learning,Item- attribute relationship
AI 理解论文
溯源树
样例
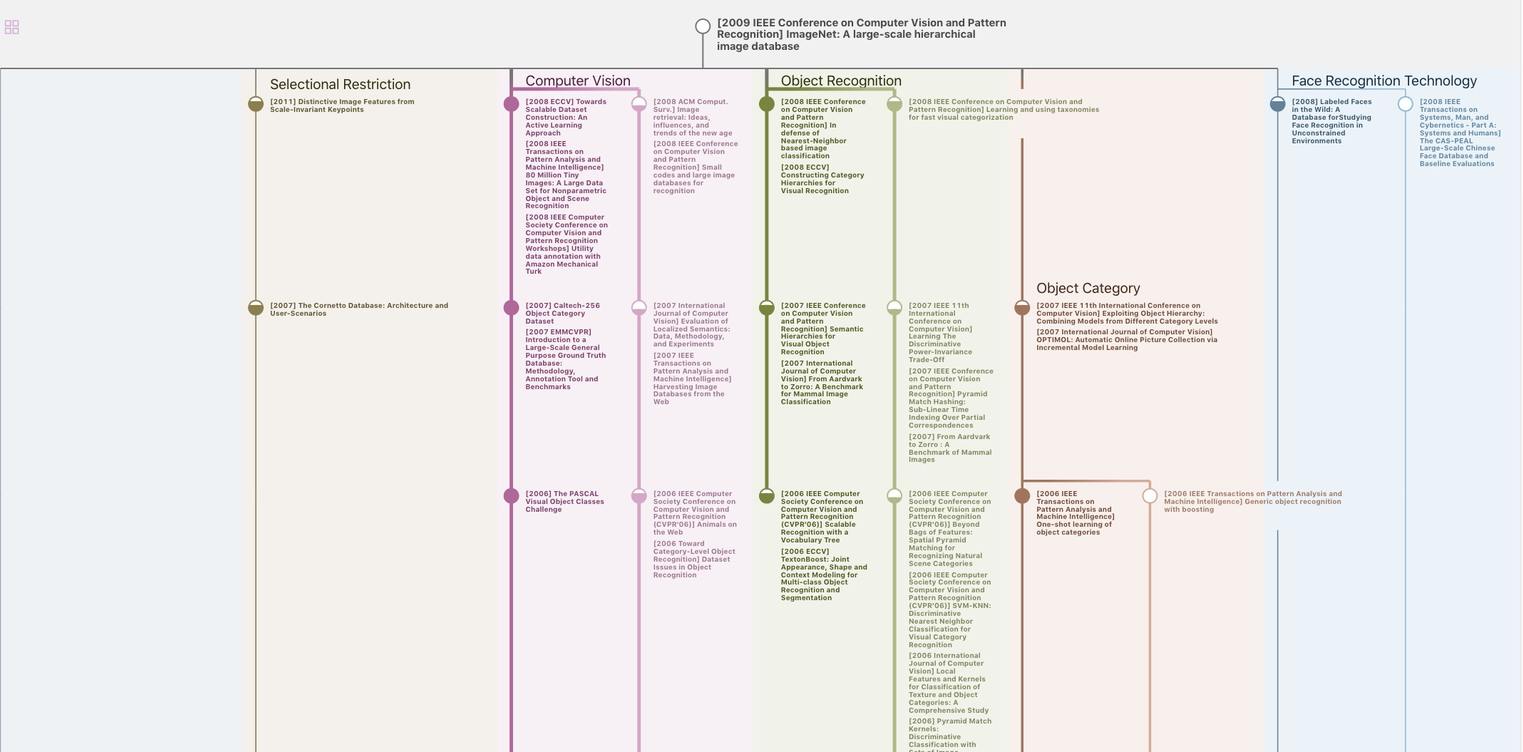
生成溯源树,研究论文发展脉络
Chat Paper
正在生成论文摘要