Improving anomaly detection in SCADA network communication with attribute extension
Energy Inform.(2022)
摘要
Network anomaly detection for critical infrastructure supervisory control and data acquisition (SCADA) systems is the first line of defense against cyber-attacks. Often hybrid methods, such as machine learning with signature-based intrusion detection methods, are employed to improve the detection results. Here an attempt is made to enhance the support vector-based outlier detection method by leveraging behavioural attribute extension of the network nodes. The network nodes are modeled as graph vertices to construct related attributes that enhance network characterisation and potentially improve unsupervised anomaly detection ability for SCADA network. IEC 104 SCADA protocol communication data with good domain fidelity is utilised for empirical testing. The results demonstrate that the proposed approach achieves significant improvements over the baseline approach (average F_1 score increased from 0.6 to 0.9, and Matthews correlation coefficient (MCC) from 0.3 to 0.8). The achieved outcome also surpasses the unsupervised scores of related literature. For critical networks, the identification of attacks is indispensable. The result shows an insignificant missed-alert rate ( 0.3% on average), the lowest among related works. The gathered results show that the proposed approach can expose rouge SCADA nodes reasonably and assist in further pruning the identified unusual instances.
更多查看译文
关键词
Supervisory control and data acquisition,Network intrusion detection,Machine learning,IEC 60870-5-104,Attribute extension
AI 理解论文
溯源树
样例
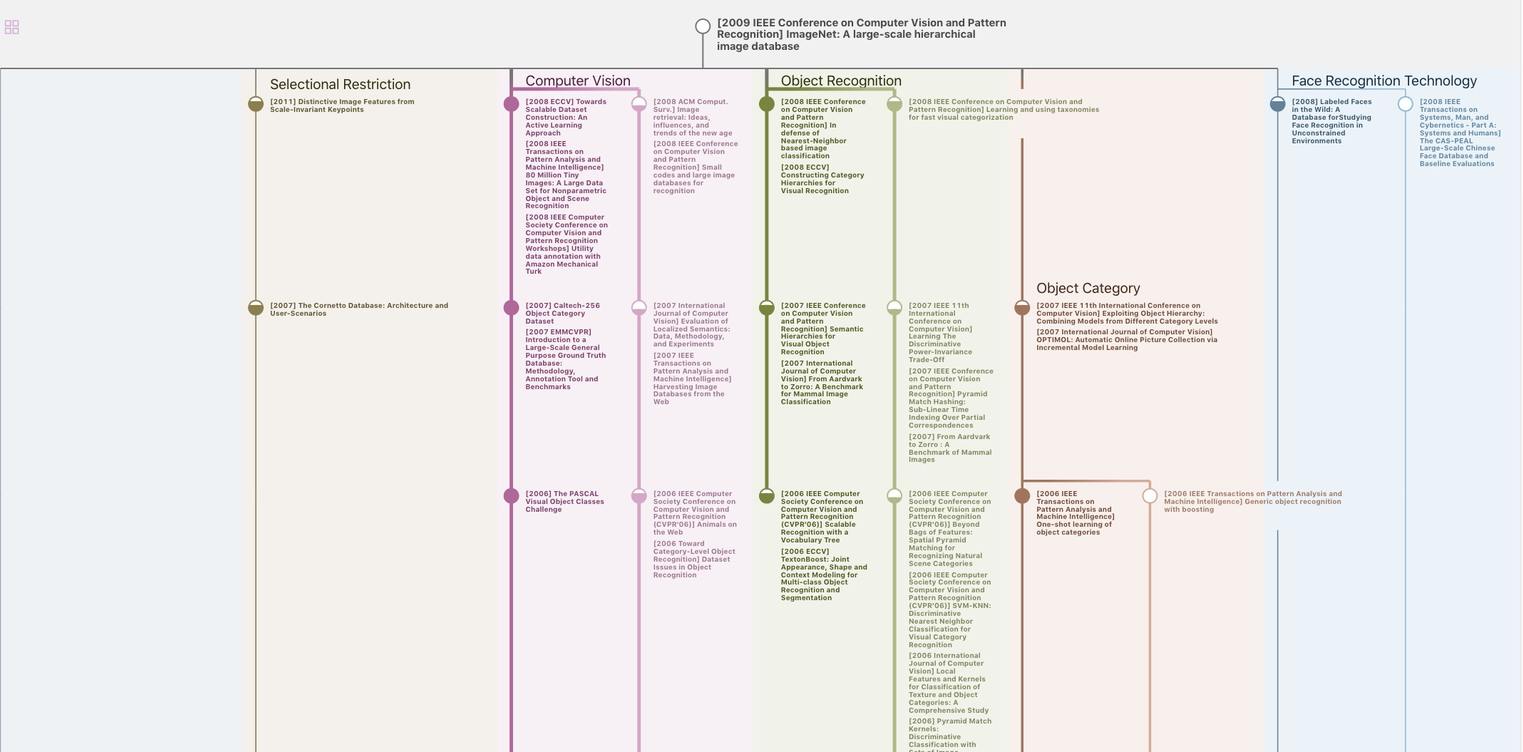
生成溯源树,研究论文发展脉络
Chat Paper
正在生成论文摘要