Acoustic Structural Integrity Assessment of Ceramics using Supervised Machine Learning and Uncertainty-Based Rejection.
SIGKDD Explor.(2022)
摘要
Industry and Quality 4.0 pose the opportunity to integrate artificial intelligence-based technology into the quality management of products/services. Particularly, quality control procedures of tableware ceramics require a demanding and faulty human manual (visual and acoustic) inspection. In this paper, we propose an uncertainty-aware automated acoustic inspection using a supervised machine learning model based on a set of novel acoustic features to classify ceramic plates, as cracked and uncracked. We conducted experiments on a dataset of 31 ceramic plates (16 cracked and 15 uncracked), collected in the laboratory. Data quality check and augmentation strategies were also performed, resulting in 2900 samples. The main contributions of this paper are: 1) description of 192 features selected for the acoustic inspection of ceramic plates; 2) comparison of model calibration results regarding three different classifiers; 3) study of different sources of uncertainty for classification with rejection option, through uncertainty quantification measures, and the effect of feature selection on it. We performed two experiments that differ in the usage of a supervised feature selection method. We split the augmented dataset into train/test sets in a proportion of 90/10. The calibrated SVM was selected as the best classifier based on model calibration and cross-validation results and was used in the prediction on the test set. The uncertainty-based rejection improved the train and test sets' classification results. In the experiment with feature selection, the classification performance remained high, while the uncertainty about the predictions and the percentage of rejected samples decreased.
更多查看译文
关键词
acoustic structural integrity assessment,ceramics,supervised machine learning,machine learning,uncertainty-based
AI 理解论文
溯源树
样例
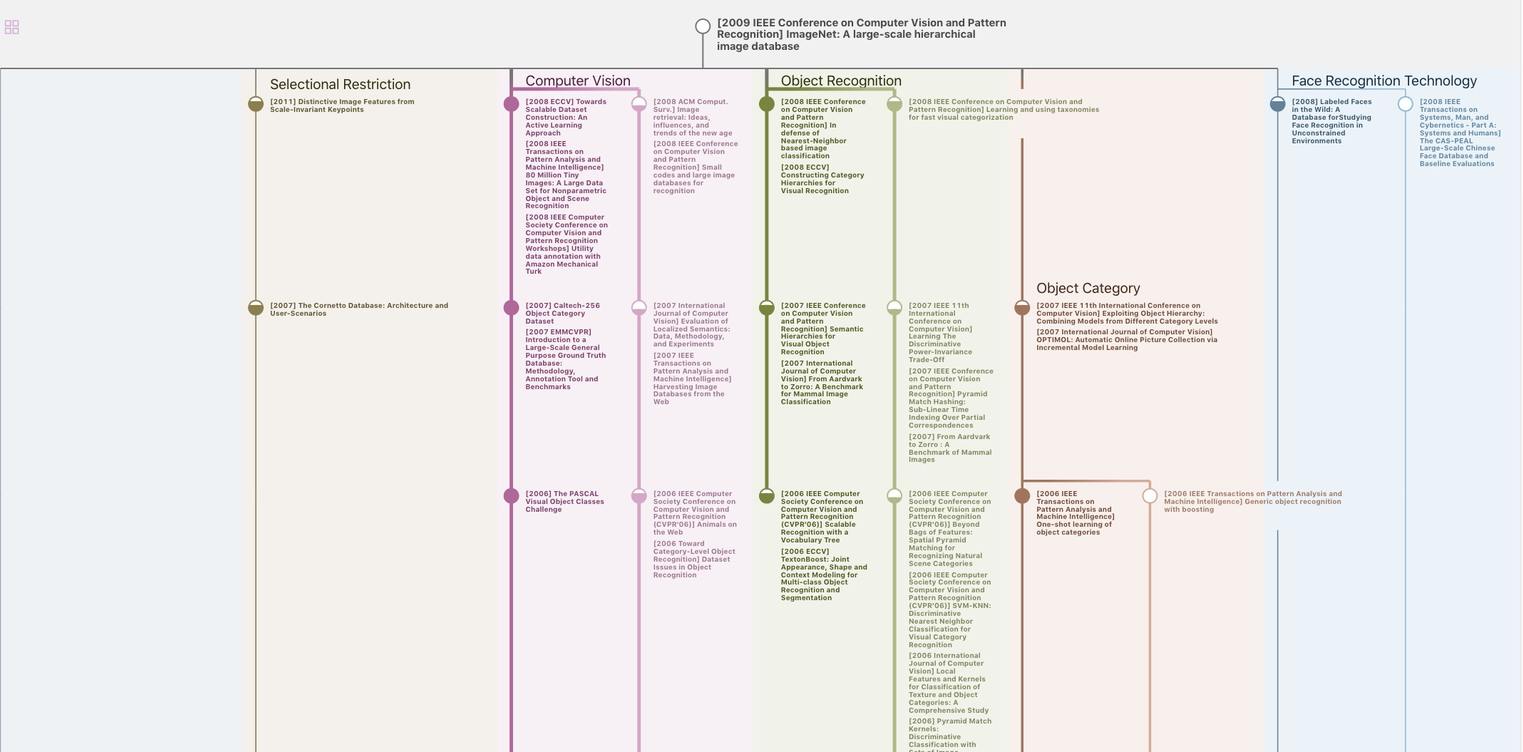
生成溯源树,研究论文发展脉络
Chat Paper
正在生成论文摘要