Comprehensive Comparisons of Uniform Quantization in Deep Image Compression.
IEEE Access(2023)
摘要
In deep image compression, uniform quantization is applied to latent representations obtained by using an auto-encoder architecture for reducing bits and entropy coding. Quantization is a problem encountered in the end-to-end training of deep image compression. Quantization's gradient is zero, and it cannot backpropagate meaningful gradients. Many methods have been proposed to address the approximations of quantization to obtain gradients. However, there have not been equitable comparisons among them. In this study, we comprehensively compare the existing approximations of uniform quantization. Furthermore, we evaluate possible combinations of quantizers for the decoder and the entropy model, as the approximated quantizers can be different for them. We conduct experiments using three network architectures on two test datasets. The experimental results reveal that the best approximated quantization differs by the network architectures, and the best approximations of the three are different from the original ones used for the architectures. We also show that the combination of quantizers that uses universal quantization for the entropy model and differentiable soft quantization for the decoder is a comparatively good choice for different architectures and datasets.
更多查看译文
关键词
Quantization (signal),Image coding,Entropy,Decoding,Training,Network architecture,Neural networks,Image compression,neural networks,quantization
AI 理解论文
溯源树
样例
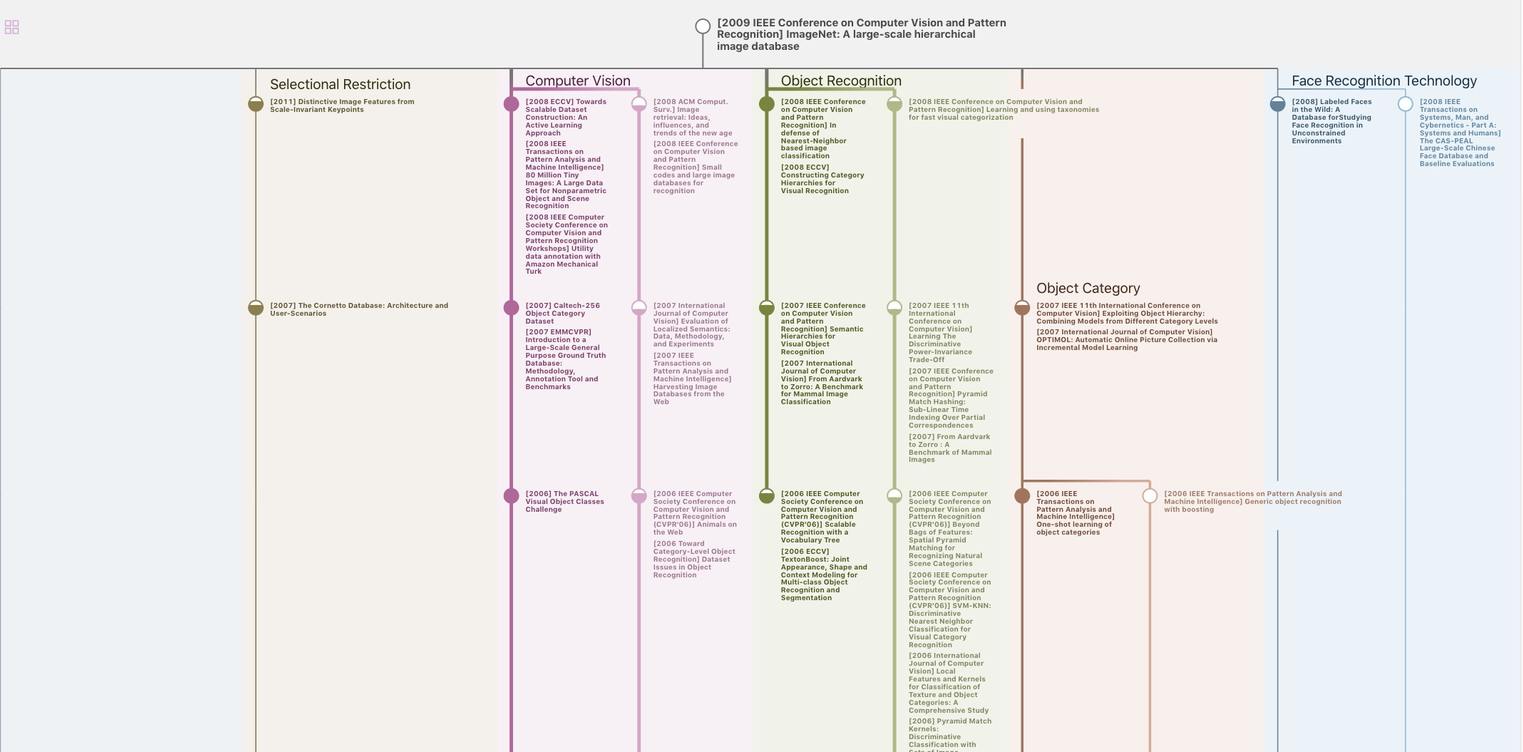
生成溯源树,研究论文发展脉络
Chat Paper
正在生成论文摘要