Physics-guided deep reinforcement learning for flow field denoising
arxiv(2023)
摘要
A multi-agent deep reinforcement learning (DRL)-based model is presented in this study to reconstruct flow fields from noisy data. A combination of the reinforcement learning with pixel-wise rewards (PixelRL), physical constraints represented by the momentum equation and the pressure Poisson equation and the known boundary conditions is utilised to build a physics-guided deep reinforcement learning (PGDRL) model that can be trained without the target training data. In the PGDRL model, each agent corresponds to a point in the flow field and it learns an optimal strategy for choosing pre-defined actions. The proposed model is efficient considering the visualisation of the action map and the interpretation of the model performance. The performance of the model is tested by utilising synthetic direct numerical simulation (DNS)-based noisy data and experimental data obtained by particle image velocimetry (PIV). Qualitative and quantitative results show that the model can reconstruct the flow fields and reproduce the statistics and the spectral content with commendable accuracy. These results demonstrate that the combination of DRL-based models and the known physics of the flow fields can potentially help solve complex flow reconstruction problems, which can result in a remarkable reduction in the experimental and computational costs.
更多查看译文
关键词
deep reinforcement learning,reinforcement learning,flow field,physics-guided
AI 理解论文
溯源树
样例
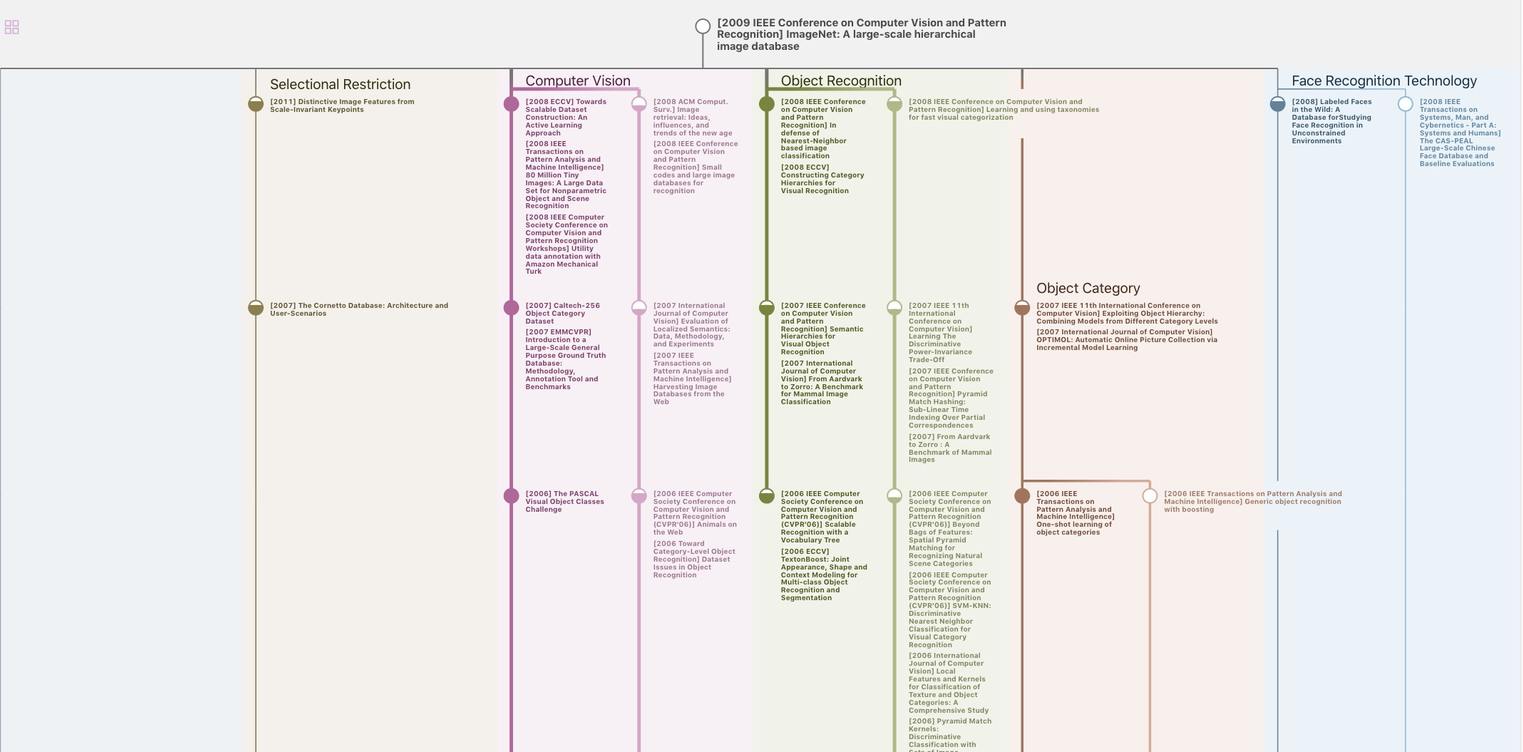
生成溯源树,研究论文发展脉络
Chat Paper
正在生成论文摘要