VQE-generated Quantum Circuit Dataset for Machine Learning
arxiv(2023)
摘要
Quantum machine learning has the potential to computationally outperform classical machine learning, but it is not yet clear whether it will actually be valuable for practical problems. While some artificial scenarios have shown that certain quantum machine learning techniques may be advantageous compared to their classical counterpart, it is unlikely that quantum machine learning will outclass traditional methods on popular classical datasets such as MNIST. In contrast, dealing with quantum data, such as quantum states or circuits, may be the task where we can benefit from quantum methods. Therefore, it is important to develop practically meaningful quantum datasets for which we expect quantum methods to be superior. In this paper, we propose a machine learning task that is likely to soon arise in the real world: clustering and classification of quantum circuits. We provide a dataset of quantum circuits optimized by the variational quantum eigensolver. We utilized six common types of Hamiltonians in condensed matter physics, with a range of 4 to 16 qubits, and applied ten different ans\"{a}tze with varying depths (ranging from 3 to 32) to generate a quantum circuit dataset of six distinct classes, each containing 300 samples. We show that this dataset can be easily learned using quantum methods. In particular, we demonstrate a successful classification of our dataset using real 4-qubit devices available through IBMQ. By providing a setting and an elementary dataset where quantum machine learning is expected to be beneficial, we hope to encourage and ease the advancement of the field.
更多查看译文
关键词
quantum circuit dataset,machine learning,vqe-generated
AI 理解论文
溯源树
样例
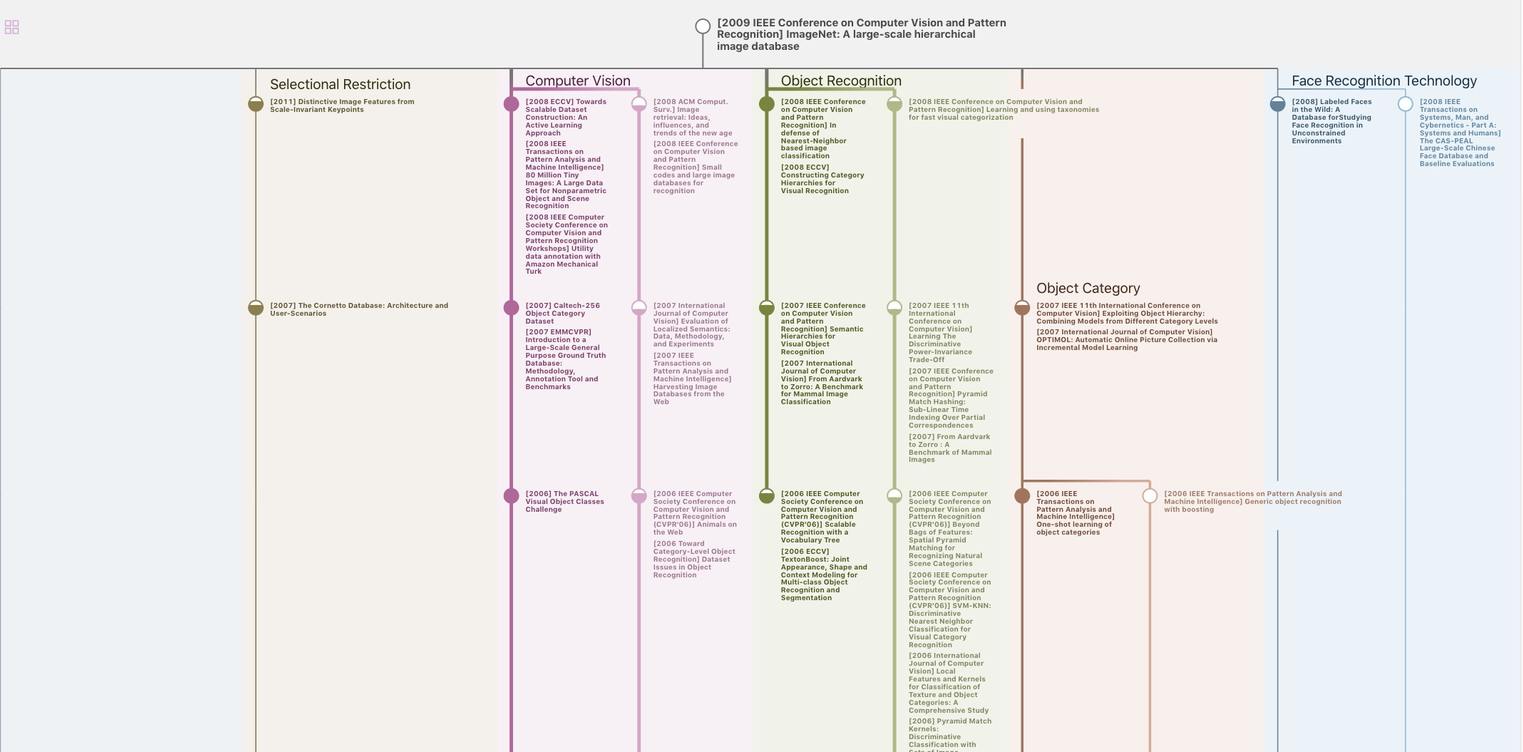
生成溯源树,研究论文发展脉络
Chat Paper
正在生成论文摘要