Unsupervised Layer-Wise Score Aggregation for Textual OOD Detection
AAAI 2024(2024)
Abstract
Out-of-distribution (OOD) detection is a rapidly growing field due to new robustness and security requirements driven by an increased number of AI-based systems. Existing OOD textual detectors often rely on anomaly scores (\textit{e.g.}, Mahalanobis distance) computed on the embedding output of the last layer of the encoder. In this work, we observe that OOD detection performance varies greatly depending on the task and layer output. More importantly, we show that the usual choice (the last layer) is rarely the best one for OOD detection and that far better results can be achieved, provided that an oracle selects the best layer. We propose a data-driven, unsupervised method to leverage this observation to combine layer-wise anomaly scores. In addition, we extend classical textual OOD benchmarks by including classification tasks with a more significant number of classes (up to 150), which reflects more realistic settings. On this augmented benchmark, we show that the proposed post-aggregation methods achieve robust and consistent results comparable to using the best layer according to an oracle while removing manual feature selection altogether.
MoreTranslated text
Key words
NLP: Safety and Robustness,NLP: Ethics -- Bias, Fairness, Transparency & Privacy,NLP: Text Classification
AI Read Science
Must-Reading Tree
Example
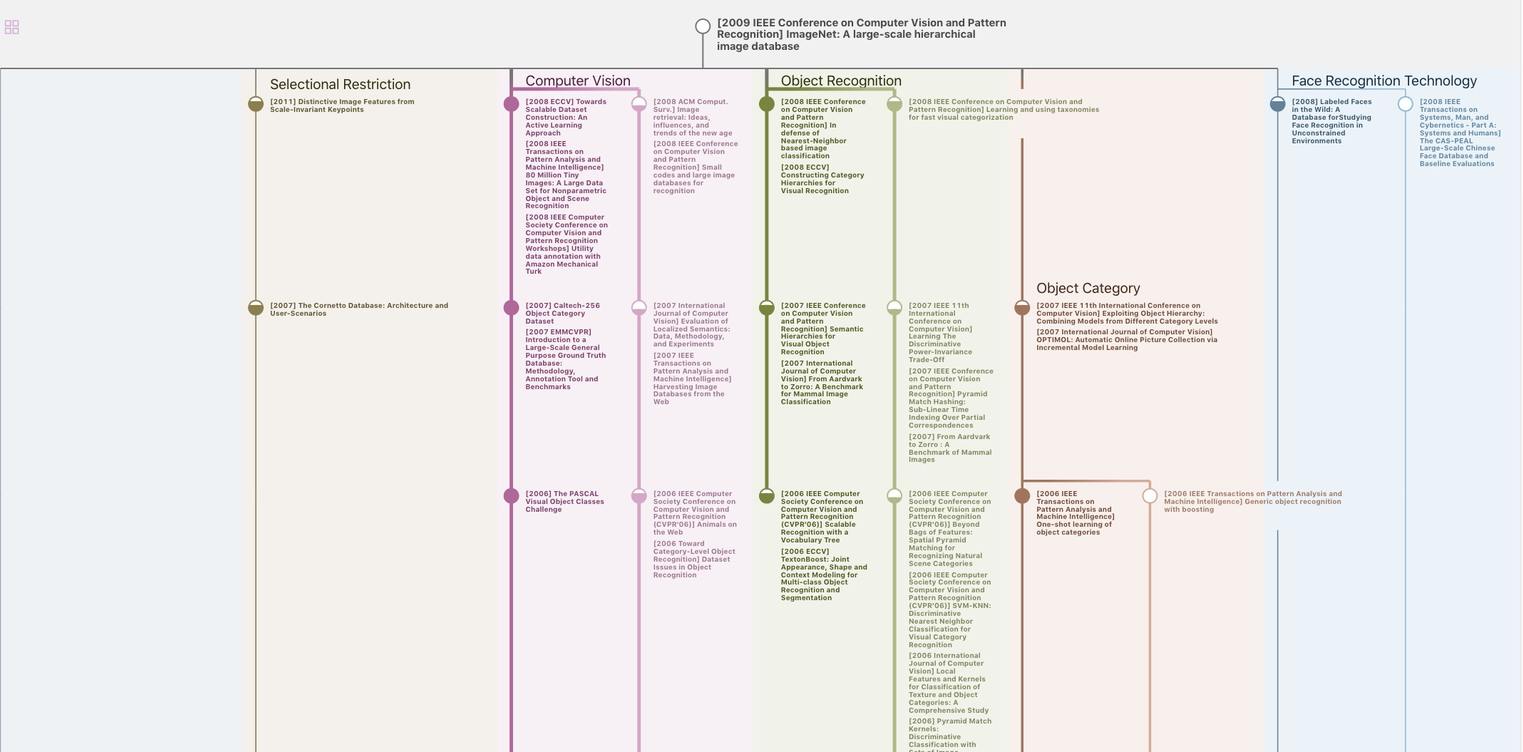
Generate MRT to find the research sequence of this paper
Chat Paper
Summary is being generated by the instructions you defined