Stability-based Generalization Analysis for Mixtures of Pointwise and Pairwise Learning
Proceedings of the AAAI Conference on Artificial Intelligence(2023)
摘要
Recently, some mixture algorithms of pointwise and pair-wise learning (PPL) have been formulated by employing the hybrid error metric of "pointwise loss + pairwise loss" and have shown empirical effectiveness on feature selection, ranking and recommendation tasks. However, to the best of our knowledge, the learning theory foundation of PPL has not been touched in the existing works. In this paper, we try to fill this theoretical gap by investigating the generalization properties of PPL. After extending the definitions of algorithmic stability to the PPL setting, we establish the high-probability generalization bounds for uniformly stable PPL algorithms. Moreover, explicit convergence rates of stochastic gradient descent (SGD) and regularized risk minimization (RRM) for PPL are stated by developing the stability analysis technique of pairwise learning. In addition, the refined generalization bounds of PPL are obtained by replacing uniform stability with on-average stability.
更多查看译文
关键词
Clustering Analysis
AI 理解论文
溯源树
样例
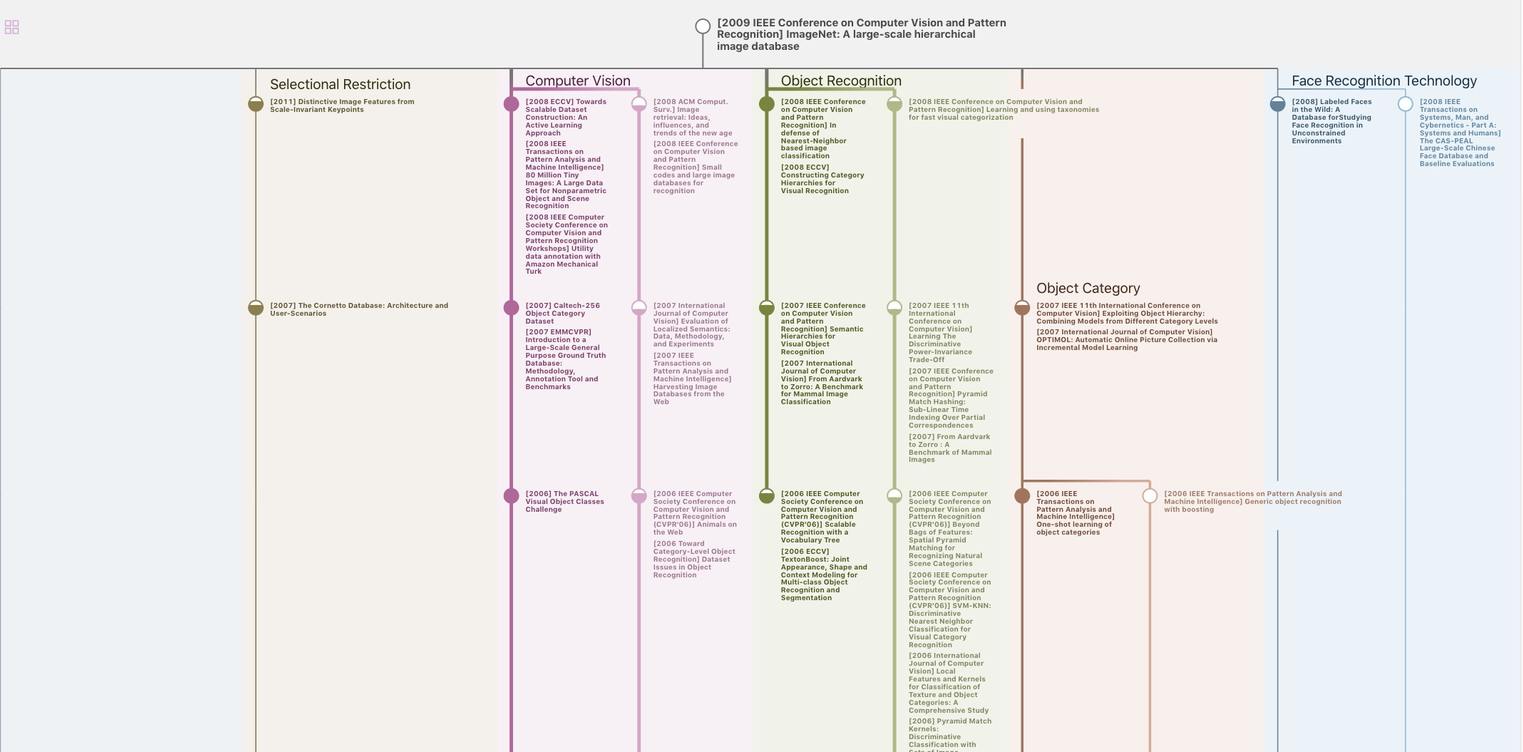
生成溯源树,研究论文发展脉络
Chat Paper
正在生成论文摘要