Optimizing the Cross-Day Performance of Electromyogram Biometric Decoder
IEEE Internet of Things Journal(2023)
摘要
With massive data collected in Internet of Things (IoT)-based smart environment, improving privacy preservation via client verification and identification is crucial. Surface electromyogram (sEMG) has emerged as a cancelable neuromuscular biometric trait, which makes up the noncancelability flaw of the traditional face and fingerprint biometrics. Current studies are in the proof-of-concept stage. In-depth studies to find the optimal solution to decode sEMG biometrics with excellent cross-day performance are very scarce. For neurophysiological biometrics, the permanence across time is a crucial factor. Our work aims to optimize the cross-day performance of the sEMG biometric decoder. We systematically evaluated the performance of 28 hand gestures to generate sEMG, 55 temporal–spectral–spatial features to represent sEMG, 9 distance measures and 9 classifiers to make decisions. Both biometric verification and identification were investigated in rigorous cross-day validations. Results show that the optimal combination of ≥ 10 temporal–spectral–spatial features achieved the best cross-day performance with city-block distance and support vector machine (SVM) applied. EMG generated by middle finger extension and hand close is preferred as biometric tokens. Using the optimized decoder, a cross-day identification accuracy of 88.75% and verification error rate of 9.85% were achieved. The verification error rate could be further reduced to 2.45% if impostors input sEMG under random gestures. Moreover, our work proved the reliability of sEMG biometrics even under muscle fatigue for the first time. This is also the first study to systematically evaluate the cross-day performance of different components in sEMG biometric decoding systems, serving as a technique-screening tool for future studies.
更多查看译文
关键词
Biometrics,machine learning,surface electromyogram (sEMG)
AI 理解论文
溯源树
样例
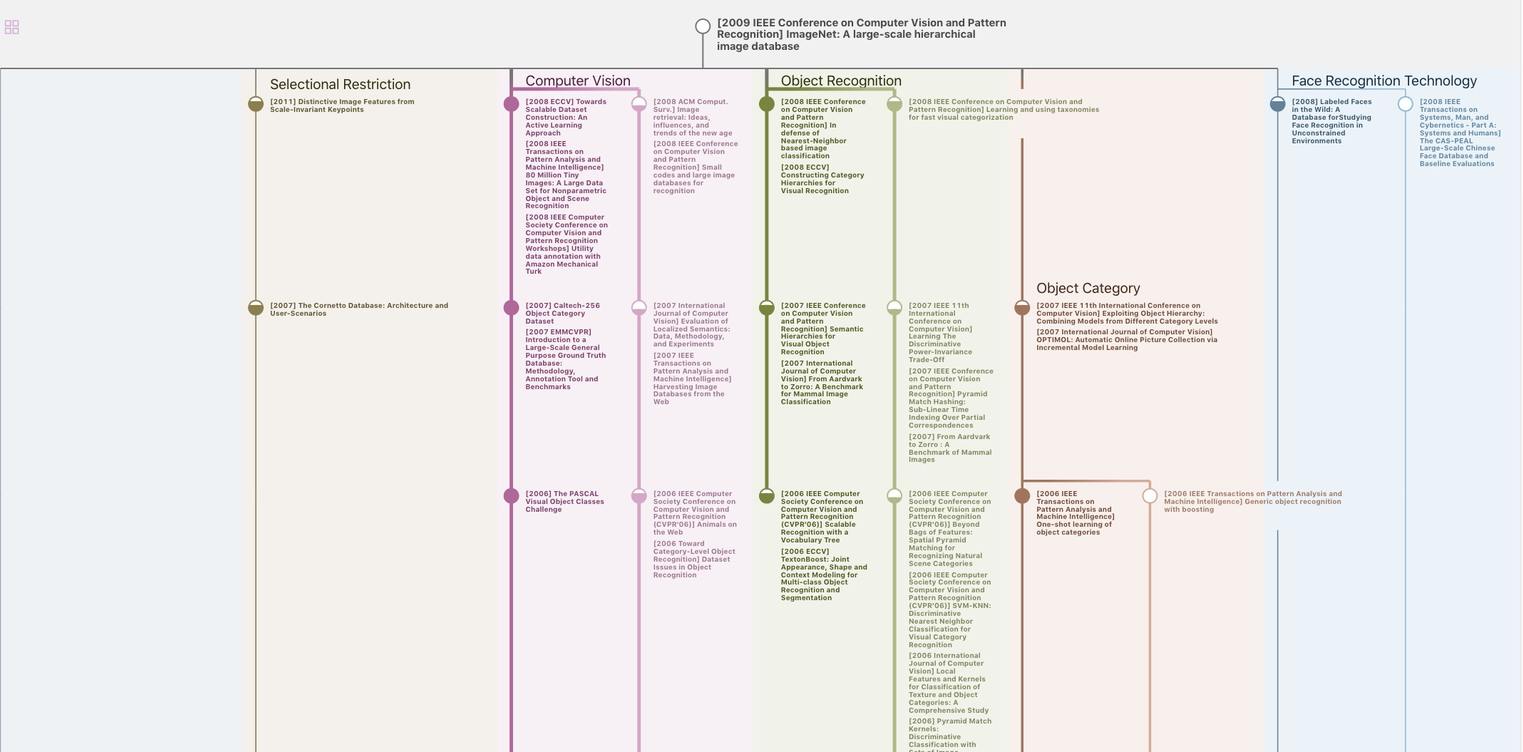
生成溯源树,研究论文发展脉络
Chat Paper
正在生成论文摘要