On distributional graph signals
arXiv (Cornell University)(2023)
摘要
Graph signal processing (GSP) studies graph-structured data, where the central concept is the vector space of graph signals. To study a vector space, we have many useful tools up our sleeves. However, uncertainty is omnipresent in practice, and using a vector to model a real signal can be erroneous in some situations. In this paper, we want to use the Wasserstein space as a replacement for the vector space of graph signals, to account for signal stochasticity. The Wasserstein is strictly more general in which the classical graph signal space embeds isometrically. An element in the Wasserstein space is called a distributional graph signal. On the other hand, signal processing for a probability space of graphs has been proposed in the literature. In this work, we propose a unified framework that also encompasses existing theories regarding graph uncertainty. We develop signal processing tools to study the new notion of distributional graph signals. We also demonstrate how the theory can be applied by using real datasets.
更多查看译文
关键词
signals,graph
AI 理解论文
溯源树
样例
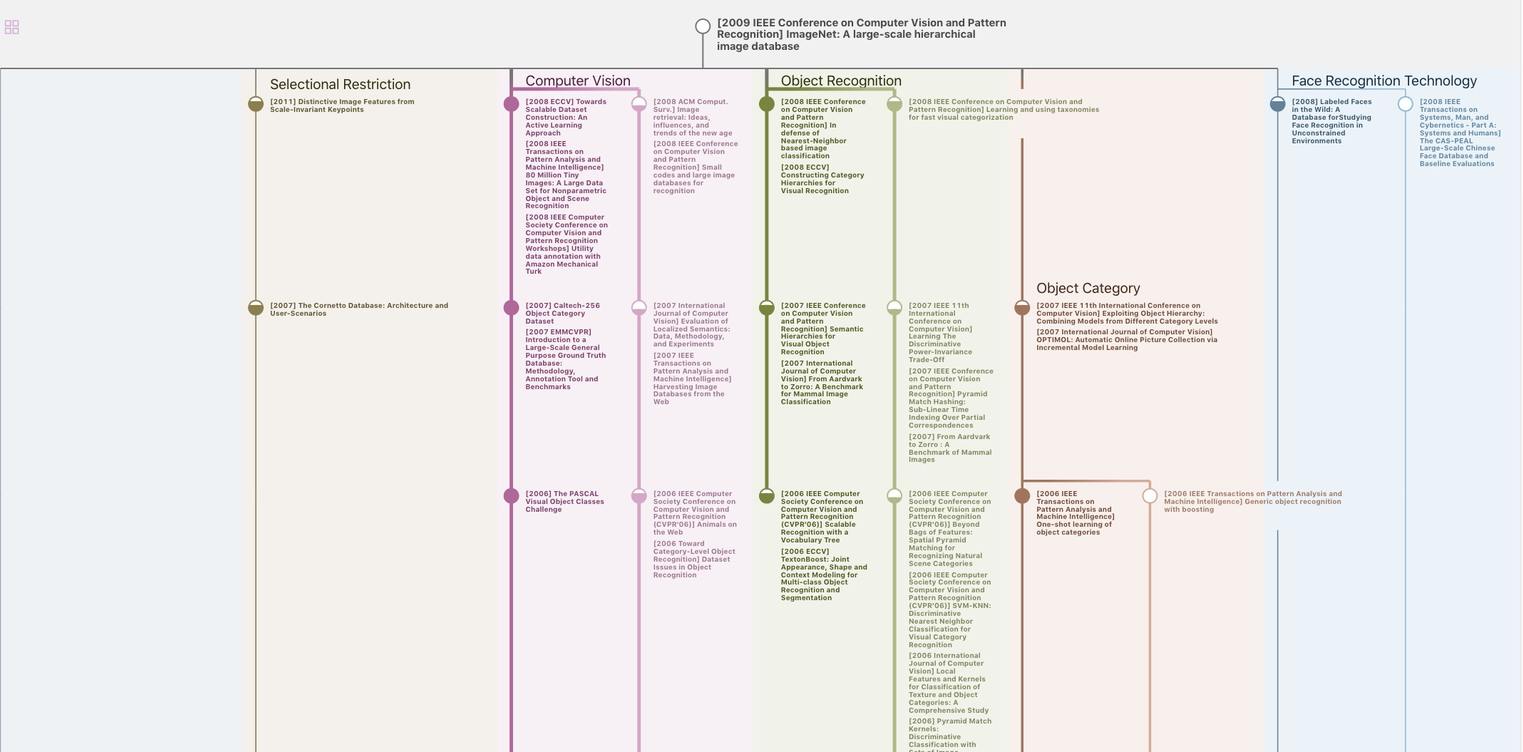
生成溯源树,研究论文发展脉络
Chat Paper
正在生成论文摘要