Debiased Distillation by Transplanting the Last Layer
CoRR(2023)
摘要
Deep models are susceptible to learning spurious correlations, even during the post-processing. We take a closer look at the knowledge distillation -- a popular post-processing technique for model compression -- and find that distilling with biased training data gives rise to a biased student, even when the teacher is debiased. To address this issue, we propose a simple knowledge distillation algorithm, coined DeTT (Debiasing by Teacher Transplanting). Inspired by a recent observation that the last neural net layer plays an overwhelmingly important role in debiasing, DeTT directly transplants the teacher's last layer to the student. Remaining layers are distilled by matching the feature map outputs of the student and the teacher, where the samples are reweighted to mitigate the dataset bias. Importantly, DeTT does not rely on the availability of extensive annotations on the bias-related attribute, which is typically not available during the post-processing phase. Throughout our experiments, DeTT successfully debiases the student model, consistently outperforming the baselines in terms of the worst-group accuracy.
更多查看译文
关键词
distillation
AI 理解论文
溯源树
样例
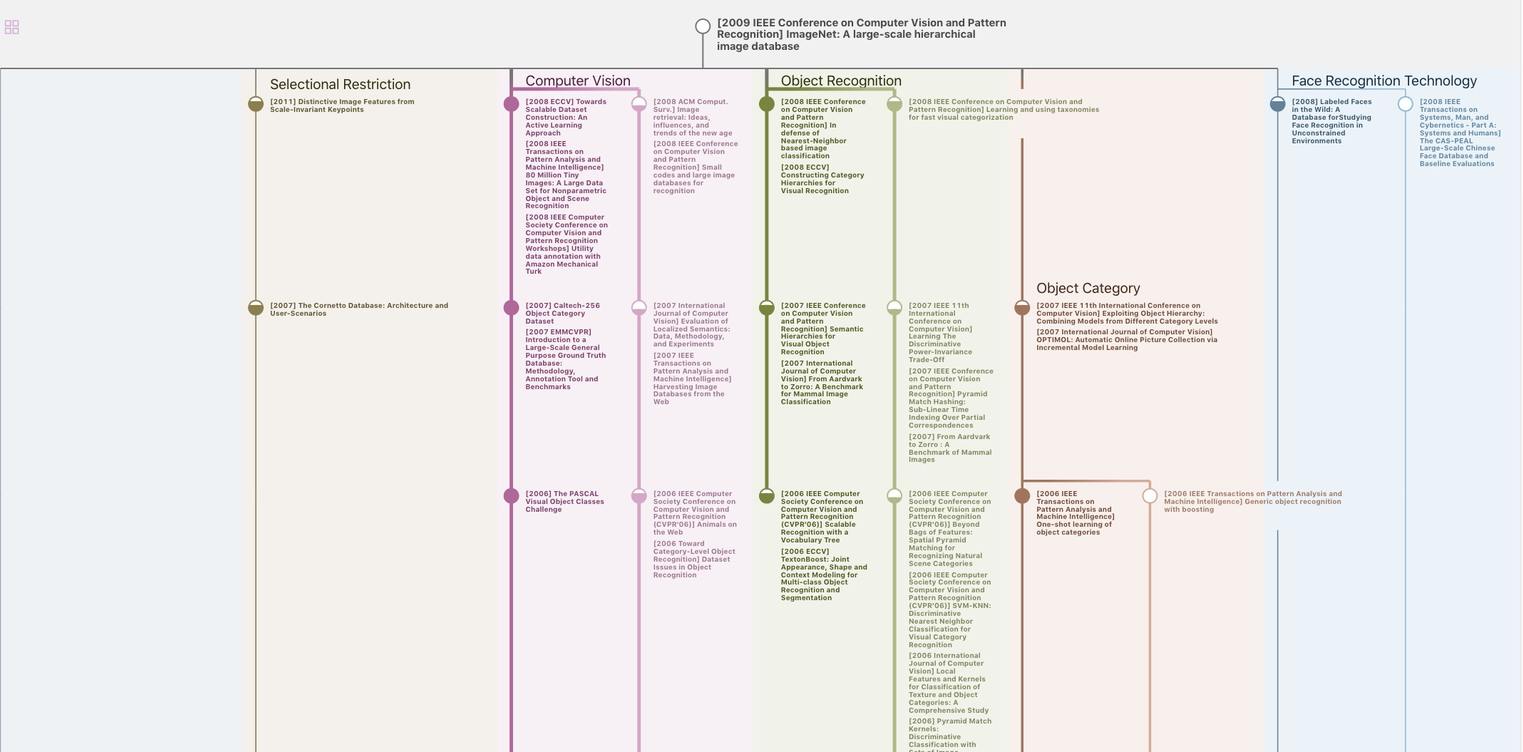
生成溯源树,研究论文发展脉络
Chat Paper
正在生成论文摘要