Time-varying Signals Recovery via Graph Neural Networks
arxiv(2023)
摘要
The recovery of time-varying graph signals is a fundamental problem with numerous applications in sensor networks and forecasting in time series. Effectively capturing the spatio-temporal information in these signals is essential for the downstream tasks. Previous studies have used the smoothness of the temporal differences of such graph signals as an initial assumption. Nevertheless, this smoothness assumption could result in a degradation of performance in the corresponding application when the prior does not hold. In this work, we relax the requirement of this hypothesis by including a learning module. We propose a Time Graph Neural Network (TimeGNN) for the recovery of time-varying graph signals. Our algorithm uses an encoder-decoder architecture with a specialized loss composed of a mean squared error function and a Sobolev smoothness operator.TimeGNN shows competitive performance against previous methods in real datasets.
更多查看译文
关键词
signals recovery,neural networks,graph,time-varying
AI 理解论文
溯源树
样例
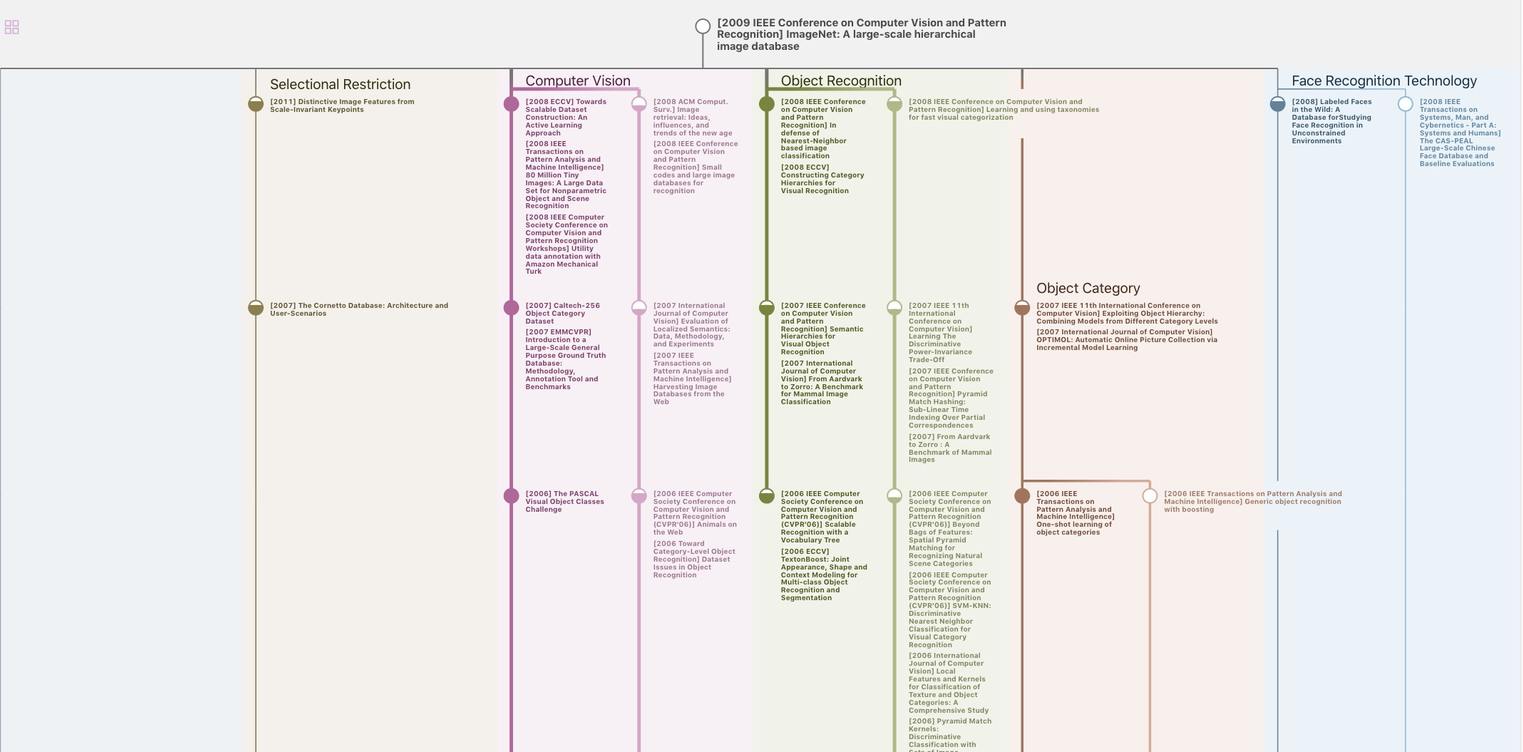
生成溯源树,研究论文发展脉络
Chat Paper
正在生成论文摘要