Accelerating convergence in wireless federated learning by sharing marginal data
2023 International Conference on Information Networking (ICOIN)(2023)
摘要
Deploying federated learning (FL) over wireless mobile networks can be expensive because of the cost of wireless communication resources. Efforts have been made to reduce communication costs by accelerating model convergence, leading to the development of model-driven methods based on feature extraction, model-integrated algorithms, and client selection. However, the resulting performance gains are limited by the dependence of neural network convergence on input data quality. This work, therefore, investigates the use of marginal shared data (e.g., a single data entry) to accelerate model convergence and thereby reduce communication costs in FL. Experimental results show that sharing even a single piece of data can improve performance by 14.6% and reduce communication costs by 61.13% when using the federated averaging algorithm (FedAvg). Marginal data sharing could therefore be an attractive and practical solution in privacy-flexible environments or collaborative operational systems such as fog robotics and vehicles. Moreover, by assigning new labels to the shared data, it is possible to extend the number of classifying labels of an FL model even when the initial input datasets lack the labels in question.
更多查看译文
关键词
Edge computing,federated learning,data sharing,wireless mobile network
AI 理解论文
溯源树
样例
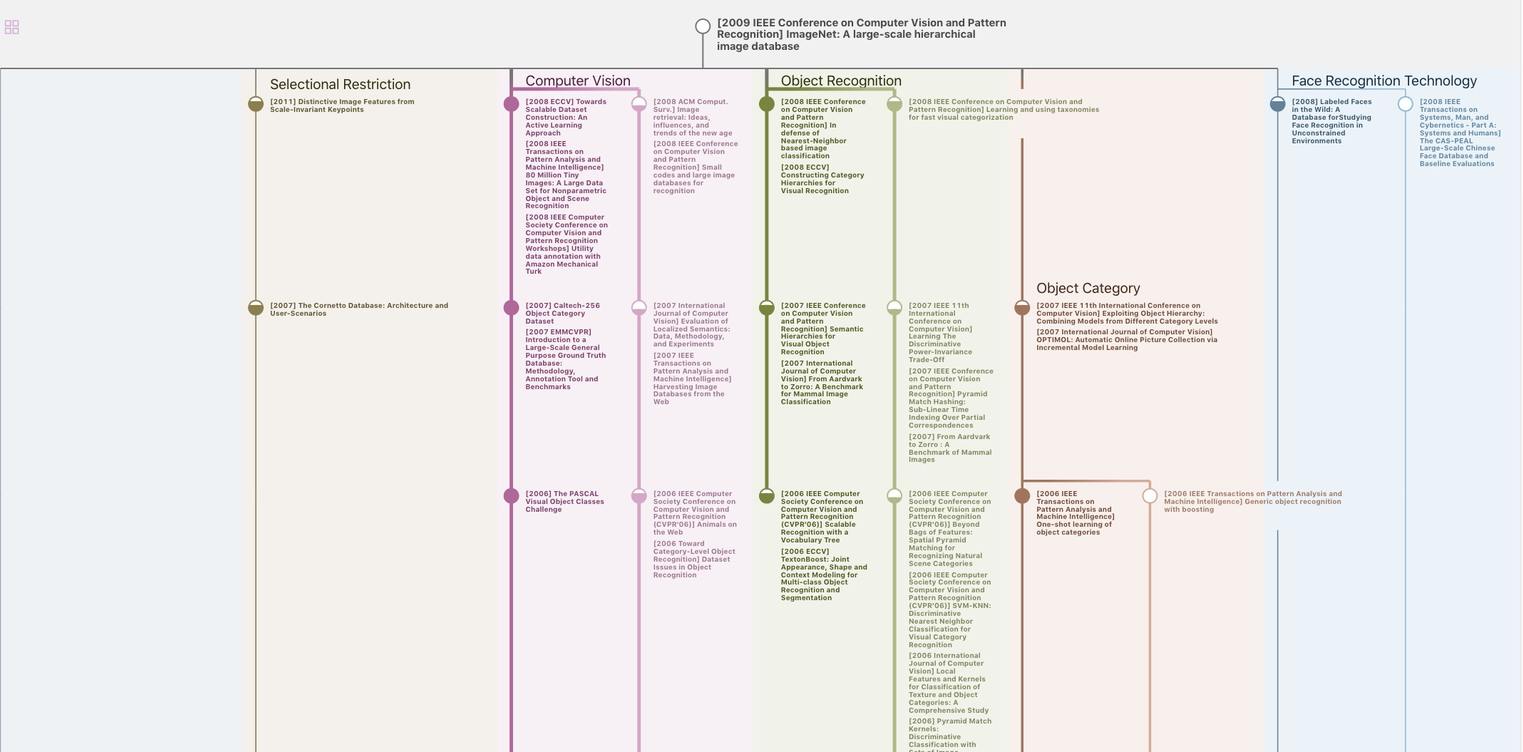
生成溯源树,研究论文发展脉络
Chat Paper
正在生成论文摘要