Open-World Object Detection via Discriminative Class Prototype Learning
arxiv(2023)
摘要
Open-world object detection (OWOD) is a challenging problem that combines object detection with incremental learning and open-set learning. Compared to standard object detection, the OWOD setting is task to: 1) detect objects seen during training while identifying unseen classes, and 2) incrementally learn the knowledge of the identified unknown objects when the corresponding annotations is available. We propose a novel and efficient OWOD solution from a prototype perspective, which we call OCPL: Open-world object detection via discriminative Class Prototype Learning, which consists of a Proposal Embedding Aggregator (PEA), an Embedding Space Compressor (ESC) and a Cosine Similarity-based Classifier (CSC). All our proposed modules aim to learn the discriminative embeddings of known classes in the feature space to minimize the overlapping distributions of known and unknown classes, which is beneficial to differentiate known and unknown classes. Extensive experiments performed on PASCAL VOC and MS-COCO benchmark demonstrate the effectiveness of our proposed method.
更多查看译文
关键词
detection,prototype,learning,object,open-world
AI 理解论文
溯源树
样例
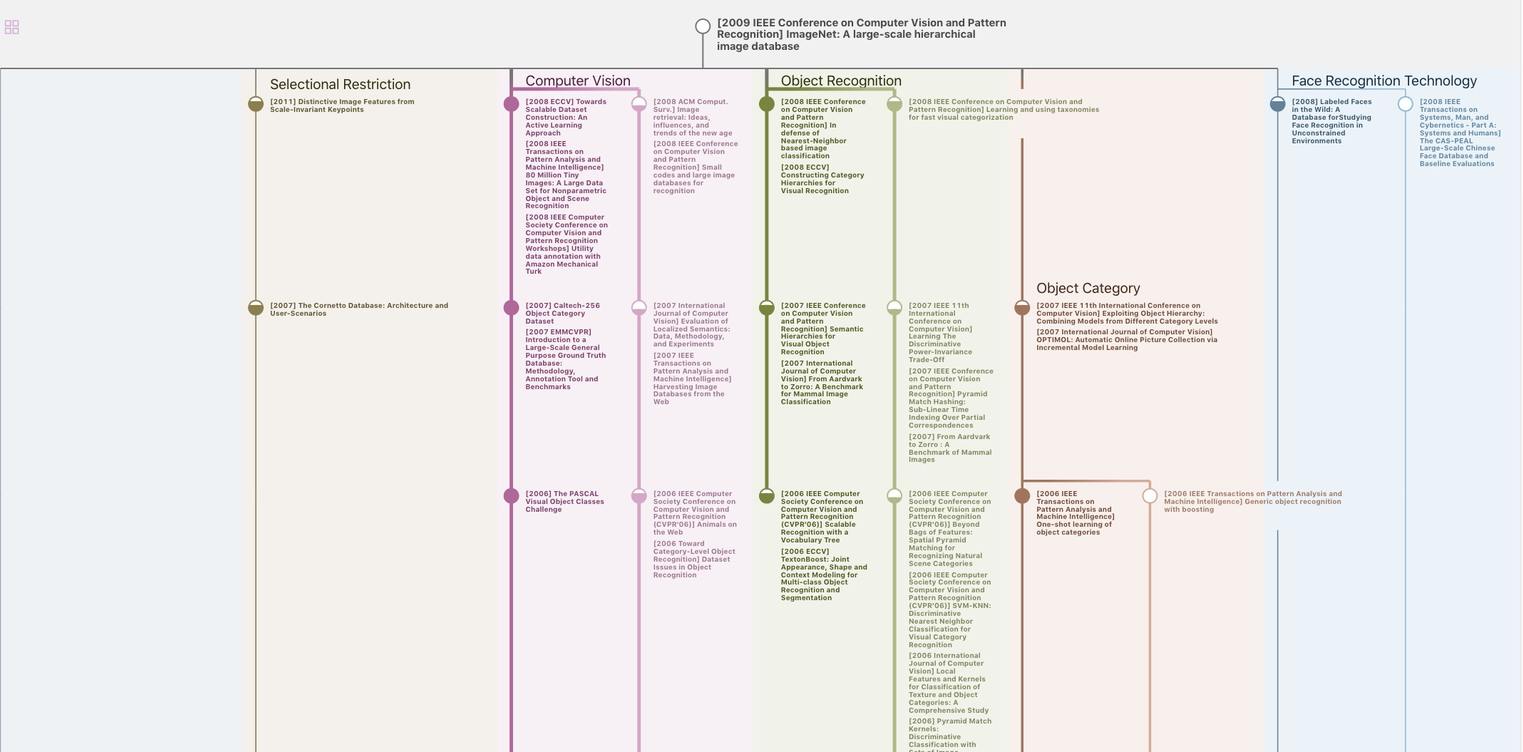
生成溯源树,研究论文发展脉络
Chat Paper
正在生成论文摘要